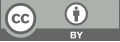
Label-refined EEG emotion classification based on intelligent media communication
- 1 Central South University
- 2 Central South University
* Author to whom correspondence should be addressed.
Abstract
With the development of cognitive computing and intelligent media communication, recognizing the user’s emotion using electroencephalography (EEG) has garnered increasing attention. However, building a cross-subject emotion recognition model with good generalization performance is really difficult. To achieve remarkable performance, a label-refined domain adversarial neural network is designed and EEG samples are subdivided into 6 groups according to the differences in users' brain activities under different movie stimuli. Based on the experiment of SEED and SEED-IV datasets, the classification accuracy of our method reaches 93.35±4.14% and 84.08±6.01%, achieving the state-of-the-art performance. Experiments show that using different movie stimuli to refine labels can achieve efficient cross-subject emotion recognition.
Keywords
EEG emotion recognition, label refinement, domain adversarial neural network
[1]. Cowie R, Douglas-Cowie E, Tsapatsoulis N, et al. (2001). Emotion recognition in human-computer interaction[J]. IEEE Signal processing magazine. 18(1): 32-80.
[2]. Alarcão S M, Fonseca M J. (2019). Emotions Recognition Using EEG Signals: A Survey[J]. IEEE Transactions on Affective Computing. 10(3): 374-393.
[3]. Li G, Chen N, Jin J. (2022). Semi-supervised EEG emotion recognition model based on enhanced graph fusion and GCN[J]. Journal of Neural Engineering. (No.2): 26039.
[4]. Peng Y, Liu H, Kong W, et al. (2022). Joint EEG Feature Transfer and Semi-supervised Cross-subject Emotion Recognition[J]. IEEE Transactions on Industrial Informatics. 1-12.
[5]. Wan Z, Yang R, Huang M, et al. (2021). A review on transfer learning in EEG signal analysis[J]. Neurocomputing. 1-14.
[6]. Ma Y, Zhao W, Meng M, et al. (2023). Cross-Subject Emotion Recognition Based on Domain Similarity of EEG Signal Transfer Learning[J]. IEEE Transactions on Neural Systems and Rehabilitation Engineering. 936-943.
[7]. Quan J, Li Y, Wang L, et al. (2023). EEG-based cross-subject emotion recognition using multi-source domain transfer learning[J]. Biomedical Signal Processing and Control. 104741.
[8]. Zhou K, Liu Z, Qiao Y, et al. (2023). Domain Generalization: A Survey[J]. IEEE Transactions on Pattern Analysis and Machine Intelligence. (No.4): 4396-4415.
[9]. Wang Y, Liu J, Ruan Q, et al. (2021). Cross-subject EEG emotion classification based on few-label adversarial domain adaption[J]. Expert Systems with Applications.
[10]. Fan J, Wang Y, Guan H, et al. (2022). Toward few-shot domain adaptation with perturbation-invariant representation and transferable prototypes[J]. Frontiers of Computer Science. (No.3): 163347.
[11]. Li J, Qiu S, Shen Y, et al. (2020). Multisource Transfer Learning for Cross-Subject EEG Emotion Recognition[J]. IEEE transactions on cybernetics. (No.7): 3281-3293.
[12]. Shen X, Liu X, Hu X, et al. (2022). Contrastive Learning of Subject-Invariant EEG Representations for Cross-Subject Emotion Recognition[J]. IEEE Transactions on Affective Computing. (No.99): 1.
[13]. Lin Y P, Wang C H, Jung T P, et al. (2010). EEG-based emotion recognition in music listening[J]. IEEE Transactions on Biomedical Engineering, vol. 57, no. 7, pp.1798-1806.
[14]. Duan R-N, Zhu, J-Y and Lu B-L. (2013). Differential entropy feature for eeg-based emotion classification. In 2013 6th International IEEE/EMBS Conference on Neural Engineering (NER). IEEE, pp. 81–84.
[15]. Zheng W-L, Liu W, Lu Y, Lu B-L, and A. Cichocki. (2018). Emotionmeter: A multimodal framework for recognizing human emotions. IEEE Transactions on Cybernetics, vol. 49, no. 3, pp. 1110–1122.
[16]. Zheng W-L, Lu B-L. (2016). Personalizing EEG-based affective models with transfer learning[C]//Proceedings of the 25th International Joint Conference on Artificial Intelligence. 2732-2738.
[17]. Shi L C, Jiao Y Y, Lu B L. (2013). Differential entropy feature for EEG-based vigilance estimation[C]. In: 2013 35th Annual International Conference of the IEEE Engineering in Medicine and Biology Society (EMBC), Osaka, Japan, pp. 6627-6630.
[18]. Ganin Y, Ustinova E, Ajakan H. (2016). Domain-Adversarial Training of Neural Networks[J]. Journal of Machine Learning Research.
[19]. Li Y, Zheng W, Wang L, et al. (2019). From Regional to Global Brain: A Novel Hierarchical Spatial-Temporal Neural Network Model for EEG Emotion Recognition[J]. IEEE Transactions on Affective Computing.
[20]. Chen H, Jin M, Li Z, Fan C, et al. (2021). MS-MDA: Multisource Marginal Distribution Adaptation for Cross-Subject and Cross-Session EEG Emotion Recognition[J]. Frontiers in neuroscience, Vol.15: 778488.
[21]. Li Y, Fu B, Li F, et al. (2021). A novel transferability attention neural network model for EEG emotion recognition[J]. Neurocomputing, Vol.447: 92-101
[22]. Li Y, Chen J, Li F, et al. (2023). GMSS: Graph-Based Multi-Task Self-Supervised Learning for EEG Emotion Recognition[J]. IEEE Transactions on Affective Computing, Vol.14(3): 2512-2525
Cite this article
Fu,Z.;Sun,Q. (2024). Label-refined EEG emotion classification based on intelligent media communication. Applied and Computational Engineering,88,72-80.
Data availability
The datasets used and/or analyzed during the current study will be available from the authors upon reasonable request.
Disclaimer/Publisher's Note
The statements, opinions and data contained in all publications are solely those of the individual author(s) and contributor(s) and not of EWA Publishing and/or the editor(s). EWA Publishing and/or the editor(s) disclaim responsibility for any injury to people or property resulting from any ideas, methods, instructions or products referred to in the content.
About volume
Volume title: Proceedings of the 6th International Conference on Computing and Data Science
© 2024 by the author(s). Licensee EWA Publishing, Oxford, UK. This article is an open access article distributed under the terms and
conditions of the Creative Commons Attribution (CC BY) license. Authors who
publish this series agree to the following terms:
1. Authors retain copyright and grant the series right of first publication with the work simultaneously licensed under a Creative Commons
Attribution License that allows others to share the work with an acknowledgment of the work's authorship and initial publication in this
series.
2. Authors are able to enter into separate, additional contractual arrangements for the non-exclusive distribution of the series's published
version of the work (e.g., post it to an institutional repository or publish it in a book), with an acknowledgment of its initial
publication in this series.
3. Authors are permitted and encouraged to post their work online (e.g., in institutional repositories or on their website) prior to and
during the submission process, as it can lead to productive exchanges, as well as earlier and greater citation of published work (See
Open access policy for details).