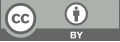
Analysis of the Effectiveness of Multi-Armed Bandit Algorithms in Crop Pricing
- 1 Anhui University, 3 Feixi Road, Hefei, Anhui, 230039, China
* Author to whom correspondence should be addressed.
Abstract
This study explores the application of multi-armed bandit (MAB) algorithms in dynamic pricing of crops, with a focus on evaluating the adaptive upper confidence bound (asUCB) and Thompson Sampling (TS) algorithms. Through simulation experiments on historical data, the study analyzed the performance of these algorithms in fitting actual market price trends and responding to future price fluctuations. The results indicate that the asUCB algorithm performed particularly well in both the training dataset and simulation tests, demonstrating low mean squared error (MSE) and minimal cumulative regret, reflecting its rapid convergence and stable pricing capabilities. In contrast, although the TS algorithm initially performed slightly less effectively, it demonstrated unique advantages in dealing with market volatility due to its strong adaptability. This study demonstrates the potential application of MAB algorithms in dynamic pricing, providing valuable insights for pricing strategies in the agricultural product market.
Keywords
Multi-Armed Bandit Algorithms, Dynamic Pricing, Market Adaptability
[1]. Bi Wenjie, Chen Gong. High-speed Rail passenger ticket dynamic Pricing Algorithm based on deep reinforcement Learning [J]. Computer applications and software,2024,41(04):228-235+261.
[2]. Zhang Yifan. Dynamic Pricing Algorithm for Niche Products based on MAB model [D]. Nanjing University,2021.
[3]. Bi Wenjie, Zhou Yubing. Research on joint inventory control and dynamic pricing of fresh products based on deep reinforcement learning [J]. Computer application research, 2022, 39(09):2660-2664.
[4]. Fang Chao, Hu Yajing, Zheng Weibo, et al. Dynamic selection strategy for new product development project portfolio based on income information uncertainty of online learning [J]. Chinese management science,2024,32(06):151-162.
[5]. Bi Wenjie, Chen Meifang. Gaussian Process Regression Dynamic Pricing Algorithm considering strategic consumers [J]. Computer Applications and Software,2024,41(02):250-256.
[6]. Tang Jue, LIU Meilian, CHENG Chengqi. A first-order Markov dynamic pricing model considering consumer loyalty [J]. Social Scientist,2023,(10):77-84
[7]. Zhang Chen, Tian Qiong. Research on online airline ticket pricing strategy considering passenger loyalty [J]. Journal of management science,2019,22(12):31-39+55.
[8]. Ma Shuangfeng, Guo Wei. Dynamic pricing of series queuing system with limited resources and its application in vehicle detection field [J]. Systems engineering theory and practice, 2023, 43(12):3653-3674.
[9]. Xu Minghui, Shen Hui, Zheng Yiwei. Research on Dynamic Pricing based on consumer time Preference and Product quality information update [J]. System Engineering Theory and Practice, 2023, 43(10):2989-3017.
[10]. Feng Zibai. Dynamic Pricing Problem with Reference Price [D]. University of Science and Technology of China, 2023.
[11]. Cui Zibin. Research on Dynamic Pricing of Competitive products in the Context of Consumer learning [D]. Guangdong University of Technology, 2022
[12]. Liu Xu. Based on the demand for learning the perishable product dynamic pricing algorithm research [D]. Southwest university of finance and economics, 2022.
Cite this article
Wang,X. (2024). Analysis of the Effectiveness of Multi-Armed Bandit Algorithms in Crop Pricing. Applied and Computational Engineering,82,1-11.
Data availability
The datasets used and/or analyzed during the current study will be available from the authors upon reasonable request.
Disclaimer/Publisher's Note
The statements, opinions and data contained in all publications are solely those of the individual author(s) and contributor(s) and not of EWA Publishing and/or the editor(s). EWA Publishing and/or the editor(s) disclaim responsibility for any injury to people or property resulting from any ideas, methods, instructions or products referred to in the content.
About volume
Volume title: Proceedings of the 2nd International Conference on Machine Learning and Automation
© 2024 by the author(s). Licensee EWA Publishing, Oxford, UK. This article is an open access article distributed under the terms and
conditions of the Creative Commons Attribution (CC BY) license. Authors who
publish this series agree to the following terms:
1. Authors retain copyright and grant the series right of first publication with the work simultaneously licensed under a Creative Commons
Attribution License that allows others to share the work with an acknowledgment of the work's authorship and initial publication in this
series.
2. Authors are able to enter into separate, additional contractual arrangements for the non-exclusive distribution of the series's published
version of the work (e.g., post it to an institutional repository or publish it in a book), with an acknowledgment of its initial
publication in this series.
3. Authors are permitted and encouraged to post their work online (e.g., in institutional repositories or on their website) prior to and
during the submission process, as it can lead to productive exchanges, as well as earlier and greater citation of published work (See
Open access policy for details).