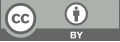
On the Performance of the Minimax Optimal Strategy in the Stochastic Case of Logistic Bandits
- 1 University of Virginia, Charlottesville, VA 22904, USA
* Author to whom correspondence should be addressed.
Abstract
The multi-armed bandit problem is a well-established model for examining the exploration/exploitation trade-offs in sequential decision-making tasks. This study focuses on the logistic bandit, where rewards are derived from two distinct datasets of movie ratings, ranging from 1 to 5, each characterized by different variances. Previous research has shown that regret bounds for multi-armed bandit algorithms can be unstable across varying environments. However, this paper provides new insights by demonstrating the robustness of the Minimax Optimal Strategy in the Stochastic (MOSS) algorithm across environments with differing reward variances. Unlike prior studies, this research shows that MOSS maintains superior performance in both dense and sparse reward settings, consistently outperforming widely used algorithms like UCB and TS, particularly in high variance conditions and over sufficient trials. The findings indicate that MOSS achieves logarithmic expected regret for both types of environments, effectively balancing exploration and exploitation. Specifically, with K arms and T time steps, the regret R(T) of MOSS is bounded by O(√(KT logT )). This work highlights MOSS as a robust solution for handling diverse stochastic conditions, filling a crucial gap in the understanding of its practical adaptability across different reward distributions.
Keywords
bandits, MOSS, sparse environment, regret bounds
[1]. Jedra, Yassir, and Alexandre Proutiere. "Optimal best-arm identification in linear bandits." Advances in Neural Information Processing Systems 33 (2020): 10007-10017.
[2]. Patil, Vishakha, et al. "Achieving fairness in the stochastic multi-armed bandit problem." Journal of Machine Learning Research 22.174 (2021): 1-31.
[3]. Simchi-Levi, David, Zeyu Zheng, and Feng Zhu. "Stochastic multi-armed bandits: optimal trade-off among optimality, consistency, and tail risk." Advances in Neural Information Processing Systems 36 (2023): 35619-35630.
[4]. Bouneffouf, Djallel, Irina Rish, and Charu Aggarwal. "Survey on applications of multi-armed and contextual bandits." 2020 IEEE Congress on Evolutionary Computation (CEC). IEEE, 2020.
[5]. Sébastien Bubeck, Michael Cohen, Yuanzhi Li. Sparsity, variance and curvature in multi-armed bandits. Proceedings of Algorithmic Learning Theory, PMLR 83:111-127, 2018.
[6]. Zimmert, Julian, and Yevgeny Seldin. "An optimal algorithm for stochastic and adversarial bandits." The 22nd International Conference on Artificial Intelligence and Statistics. PMLR, 2019.
[7]. Vural, Nuri Mert, et al. "Minimax optimal algorithms for adversarial bandit problem with multiple plays." IEEE Transactions on Signal Processing 67.16 (2019): 4383-4398.
[8]. Lee, Chung-Wei, et al. "Achieving near instance-optimality and minimax-optimality in stochastic and adversarial linear bandits simultaneously." International Conference on Machine Learning. PMLR, 2021.
[9]. Vial, Daniel, Sanjay Shakkottai, and R. Srikant. "Robust multi-agent multi-armed bandits." Proceedings of the Twenty-second International Symposium on Theory, Algorithmic Foundations, and Protocol Design for Mobile Networks and Mobile Computing. 2021.
[10]. Fouché, Edouard, Junpei Komiyama, and Klemens Böhm. "Scaling multi-armed bandit algorithms." Proceedings of the 25th ACM SIGKDD International Conference on Knowledge Discovery & Data Mining. 2019.
[11]. Liu, Xingchi, et al. "Risk-aware multi-armed bandits with refined upper confidence bounds." IEEE Signal Processing Letters 28 (2020): 269-273.
Cite this article
Liu,Y. (2024). On the Performance of the Minimax Optimal Strategy in the Stochastic Case of Logistic Bandits. Applied and Computational Engineering,83,130-139.
Data availability
The datasets used and/or analyzed during the current study will be available from the authors upon reasonable request.
Disclaimer/Publisher's Note
The statements, opinions and data contained in all publications are solely those of the individual author(s) and contributor(s) and not of EWA Publishing and/or the editor(s). EWA Publishing and/or the editor(s) disclaim responsibility for any injury to people or property resulting from any ideas, methods, instructions or products referred to in the content.
About volume
Volume title: Proceedings of CONF-MLA 2024 Workshop: Semantic Communication Based Complexity Scalable Image Transmission System for Resource Constrained Devices
© 2024 by the author(s). Licensee EWA Publishing, Oxford, UK. This article is an open access article distributed under the terms and
conditions of the Creative Commons Attribution (CC BY) license. Authors who
publish this series agree to the following terms:
1. Authors retain copyright and grant the series right of first publication with the work simultaneously licensed under a Creative Commons
Attribution License that allows others to share the work with an acknowledgment of the work's authorship and initial publication in this
series.
2. Authors are able to enter into separate, additional contractual arrangements for the non-exclusive distribution of the series's published
version of the work (e.g., post it to an institutional repository or publish it in a book), with an acknowledgment of its initial
publication in this series.
3. Authors are permitted and encouraged to post their work online (e.g., in institutional repositories or on their website) prior to and
during the submission process, as it can lead to productive exchanges, as well as earlier and greater citation of published work (See
Open access policy for details).