1. Introduction
With the advancement and breakthrough of science and technology, biometric technology is widely used in people's daily life in today's society. As the most well-known and mature technology, fingerprint recognition has become an indispensable means in the process of identity authentication and identification. This technology is widely used in different scenarios due to its high accuracy, convenience, speed, reliability and difficulty in forging, such as mobile phone unlocking, online payment, identity collection and verification, customs security inspection, on-site evidence collection and case detection.
Experts have found human fingerprints on a large number of artifacts and antiques, but fingerprint technology was not taken seriously before the 16th century [1]. In 1684, Nehemiah Grew first scientifically described the existence of fingerprints [2]. In 1880, Henry Foltz used biological theories and methods to standardize fingerprint research, and concluded that fingerprints are different from each other, and confirmed the theory that fingerprints remain unchanged throughout life [3]. In 1892, Francis Galton, a famous British anthropologist, summarized the achievements of his predecessors and proposed that fingerprints are different for each person and remain unchanged throughout life [4]. Fingerprints can be classified and identified. With the assistance of Sub-Inspectors Azizul Haque and Hemchandra Bose, Henry created a new fingerprint categorization system based on Galton's system between July 1896 and February 1897 [5]. The British Home Office officially recognized that no two individuals possess identical fingerprints. Consequently, law enforcement agencies began to heavily fund scientific research on fingerprint identification and trained numerous experts. For example, the Federal Bureau of Investigation (FBI) fingerprint identification division was set up in 1924. In 1975, the FBI started funding National Institute of Standards & Technology (NIST) to research algorithms, which led to the development of the M40 algorithm. In addition, French and England also start to develop their own Automatic Fingerprint Identification Systems (AFISs). With the rapid advancement of deep learning over the past decade, a paradigm shift in research has become inevitable. While current technology is sufficient for basic biometric recognition, deep learning can play a crucial role in addressing the challenges of identifying partial fingerprints from crime scenes and combating increasingly sophisticated techniques for creating fake fingerprints.
A fingerprint recognition system is a biometric technology that confirms identity by recording and comparing the details of human fingerprints. Through machine learning, the accuracy of this recognition method can be significantly enhanced. The technical procedure consists of two primary components. Extracting fingerprint features comes first. In this step, a pre-trained neural network is used to segment the image and forecast orientation patterns after estimating the fingerprint center using a Region-based Convolutional Neural Network (R-CNN) that has been trained on a large number of samples. This process enables the predictive analysis of the pose, orientation field, and singular points of partial fingerprints, as well as the skeleton image, minutiae points, and inner and outer contours. The second step involves aligning the generated fingerprint with the reference fingerprint within a unified coordinate system. Based on minutiae points, dense registration is performed on the fixed-length matching results, with appropriate deformation correction applied. Additionally, techniques such as Siamese networks, spatial transformer networks, and Generative Adversarial Networks (GAN) are utilized to further improve recognition accuracy. Of course, no technology is perfect. This technology faces significant challenges in practical application, such as obtaining a sufficiently large fingerprint database to support training, ensuring the accuracy of reconstructed 3D simulated fingerprints, and addressing potential ethical and privacy concerns.
This paper systematically explores the fundamental principles of fingerprint recognition technology, delves into algorithm design, examines various application scenarios, and discusses future development trends. The objective is to offer theoretical insights and technical references that can contribute to improving the practicality and security of fingerprint recognition technology.
2. Methodology
2.1. Dataset description and preprocessing
In this study, several datasets were used to train the model and verify the recognition accuracy of the model, such as NIST Special Database 4, NIST Special Database 14 NIST Special Database 301 Nail to Nail (N2N) Fingerprint Challenge Dry Run [6], Fingerprint Verification Competition (FVC). At the same time, due to the cost and privacy issues involved in large-scale fingerprint data collection today, the current public fingerprint database is relatively small, which has certain limitations on the training accuracy of the model. Therefore, thesis also use GAN to generate some pseudo fingerprints to assist in training.
2.2. Proposed approach
2.2.1. Overview. The logical structure of the model used in this study is divided into two main components: fingerprint feature extraction and matching. The fingerprint feature extraction process is particularly critical, as it directly influences the final recognition outcome. This process can be further broken down into several key steps: fingerprint posture estimation, direction field estimation, ridge and minutiae point extraction, and 3D finger reconstruction. Accurately capturing data at each of these stages is essential for achieving reliable recognition results. The pipeline is shown in the Figure 1.
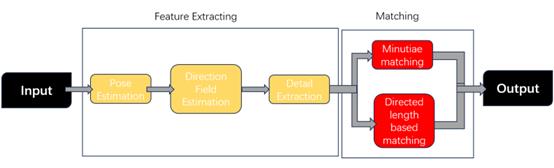
Figure 1. Flowchart of fingerprint recognition system.
The pose estimation part can be well completed by Faster R-CNN technology [7]. The direction field estimation is done by cutting the fingerprint image into blocks and splicing them together through a pre-trained convolutional neural network (ConvNet) to form the entire direction field [8]. Then the minutiae point extraction is completed by the weight-learnable FingerNet [9]. For fingerprint matching, the technical difficulty lies in what kind of feature matching to use. Traditional matching features include minutiae matching [10], fixed-length matching [11], rigid registration, etc. This article mainly discusses the fixed-length matching technology, which is relatively more influenced by deep learning and has made rapid progress.
2.2.2. Faster R-CNN. First, for an input fingerprint image, the pre-trained Faster R-CNN can generate hundreds of possible prediction boxes. The direction types of these prediction boxes are mostly close to the true direction. In order to further filter the prediction results to make them better match the true direction, the researchers use a specific screening method to filter the prediction directions and combine them by weight to get close to the true direction.
Specifically, the model will first classify these prediction boxes into dozens of major categories. Then, for the prediction boxes contained in each major category, by gradually increasing the confidence threshold, the prediction boxes with confidence greater than or equal to the confidence threshold are retained, so that only one bounding box is retained for all major categories except those close to the true direction. For the major categories close to the true direction, the non-maximum suppression (NMS) technology is used to reduce them to one bounding box. Then, in the inter-class combination step, the bounding box with the highest probability is selected first, and the remaining bounding boxes can be divided into two types at this time. First, a weighted method will incorporate the direction prediction result if the probability exceeds the threshold and the angle between the selected direction and the direction is smaller than the threshold. For the bounding boxes that do not meet the above conditions, they are automatically ignored. Compared with traditional methods, this screening combination mechanism can improve the consistency of different prediction directions to a certain extent.
2.2.3. ConvNet. In this step, the researchers proposed a new perspective to solve the problem of directional field estimation. They transformed the problem into a classification problem and used a ConvNet to solve it. Specifically, in the pre-training part, the researchers used the NIST System Dynamics (SD) 4 database to obtain all 2000 16*16-pixel directional fields, including five types of fingerprints, 400 of each type. Then, 10*10 directional fields were extracted again and these new directional fields were classified into 128 categories by the fast K-means clustering method. In addition, the researchers also used the NIST SD14 dataset to extract more training samples and perform noise training. For a given scene image, the model will (1) remove large-scale noise enhancement images, (2) divide the image into a large number of image blocks, and each small detail is divided into several image blocks to pursue high accuracy, (3) send the cut image to ConvNet for processing to predict its directional pattern and output the relevant probability or confidence, (4) combine all prediction methods together and adjust the contradictions appropriately to form the entire directional field.
2.2.4. FingerNet. This method is based on the traditional algorithm and uses deep learning technology to expand and enhance it. The traditional fingerprint processing process can be summarized as (1) direction estimation, (2) segmentation, (3) enhancement, and (4) detail point extraction. The researchers transformed these steps into a CNN with fixed weights. Then, it was further expanded into the FingerNet network. The detail extraction part was significantly enhanced in this process. Specifically, after inputting the enhanced output obtained in the previous steps, the module will generate four different graphs: the first graph is the detail score graph, which is used to indicate whether there is a detail at this location; the second and third graphs represent the probability distribution graphs in the x and y directions, respectively, which are used for accurate positioning through 8 discrete position classification tasks; the fourth graph is used to represent the angular direction of the detail point. After obtaining enough data, the results are filtered by appropriate thresholds and redundant details are cut out by NMS to make the results clear and distinguishable. In order to distinguish the direction of the detail point and the direction of the direction field, the researchers used strong and weak labels for marking, the former is marked as a strong label, and the latter is marked as a weak label.
2.2.5. DeepPrint. DeepPrint technology can be regarded as an important step in the development of fixed-length fingerprint matching. The implementation of this technology relies on three modules: The first module is the alignment module. In this module, the traditional solution is to give some specific reference points to assist the transformation. The researchers used the attention mechanism instead. This means that the positioning network can learn how to transform accurately through autonomous learning, so that it can gradually better distinguish the fingerprint direction. After this step, the fingerprint will be aligned to the same coordinate system for subsequent comparison.
The second and third modules are carried out simultaneously. The main tasks of both modules are to extract features based on the aligned images obtained in the previous step, and classify them to determine the "owner" of the fingerprint image. However, the features they rely on are slightly different. The first one relies on the overall texture features and is highly dependent on the ridge direction and frequency, while the second one relies on the details for resolution. Finally, each of them will give a 96-dimensional vector, and the final result will be the concatenation of the two vectors after normalization. Compared with using two independent models, the advantage of using this method is that it can reduce the complexity of the model.
3. Result and Discussion
3.1. Results of faster R-CNN
Figures 2 and 3 respectively show the results given by different algorithms. The red arrows represent the results from Ouyang [7], while the blue arrows represent the results of the previous state-of-the-art algorithm by Su [12]. Each column in Figure 2 corresponds to the same fingerprint object. It can be observed that in the vast majority of cases, the algorithm by Ouyang [7] produces estimates with smaller directional deviations, generally maintaining the same direction. In contrast, the results from Su algorithm show a higher dependence on the quality of the fingerprint image, with deviations remaining at a relatively large level [12]. This situation is particularly evident in the test results of groups (b) and (f). Figure 3 illustrates the differences in robustness of different algorithms under various noise interferences. It can be seen that the new algorithm clearly has a higher resistance to interference, which is notably evident in images (c), (d), and (e).
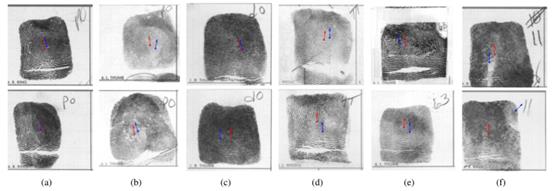
Figure 2. Comparison of the stability of two pose estimation methods.
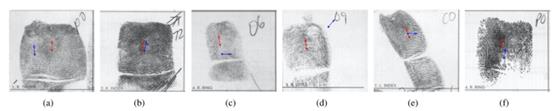
Figure 3. Examples of pose estimation.
3.2. Test results of direction field estimation
Column (a) displays a comparison of orientation fields produced by three distinct algorithms on three distinct clipped latents. (b)–(d) The orientation fields that were acquired using the global orientation patch dictionary, the suggested ConvNet-based technique, and the ridge structure dictionary [13], and (e) the mated rolled prints of (a). To improve visual quality, these latents' contrast has been increased.
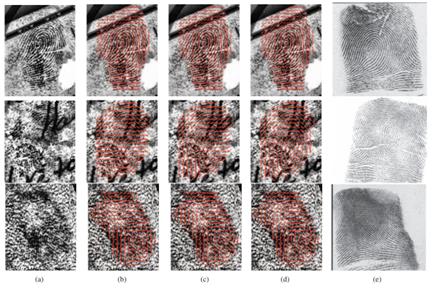
Figure 4. Comparison of orientation fields.
Table 1 and Figure 4 present the test results. Using the NIST SD27 latent fingerprint database, the researchers assessed the root mean square deviation of several algorithms in the experiment. Figure 4 compares the accuracy of several algorithms when applied to images with manually labeled direction fields. The results indicate that the deviation of the proposed algorithm is significantly smaller than that of other algorithms. This means that for the same fingerprint, the direction field produced by this algorithm is more consistent and less influenced by image variations. Furthermore, the algorithm's estimation of the direction field is more accurate than other algorithms and, in some cases, even surpasses the accuracy of manually labeled direction fields. Additionally, the last two rows of data in the table demonstrate that incorporating noise simulation during training can effectively enhance the algorithm's accuracy, making it better suited for real-world images, particularly those with high levels of noise. This improvement increases the algorithm's practicality and robustness in everyday applications.
Table 1. Average Root Mean Square Deviation (RMSD) of different orientation field estimation algorithms on NIST SD27 latent database.
Algorithm |
All |
Good |
Bad |
Ugly |
LocalizedDict[14] (manually marked pose) |
13.76 |
10.87 |
14.12 |
16.40 |
LocalizedDic[14] |
14.35 |
11.15 |
15.15 |
16.85 |
GlobalDic [13] |
18.44 |
14.40 |
19.18 |
21.88 |
RidgeDic [12] |
19.53 |
15.34 |
20.70 |
22.68 |
Proposed (without noise simulation) |
14.44 |
11.42 |
14.61 |
17.41 |
Proposed (with noise simulation) |
13.51 |
10.76 |
13.94 |
16.00 |
3.3. FingeNet results
A variety of metrics can be used to assess minutiae extraction performance; the precision-recall curve was employed in this investigation. Recall is the genuine positive rate, whereas precision is the positive forecast value. The algorithms compared include MINDTCT from NIST, Gabor-based algorithms, Autoencoder-based algorithms, FCN-based algorithms, and VeriFinger, all of which are considered relatively advanced. As shown in Figures 5, 6, and 7, the new algorithm tested on the NIST SD27 database achieved average position and angle errors of 4.4 pixels and 5.0 degrees, respectively, while tests on the FVC 2004 database yielded errors of 3.4 pixels and 6.4 degrees. These results indicate an overall improvement in recognition ability compared to previous algorithms. Notably, the recognition rate, as demonstrated by the Cumulative Match Characteristic (CMC) curve, is nearly double that of the second-best Fully Convolutional Network (FCN) algorithm. These findings clearly demonstrate that the new algorithm outperforms its predecessors in all evaluated aspects.
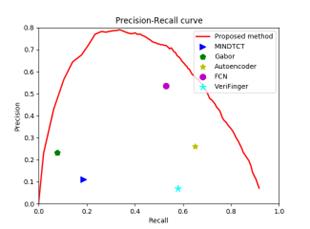
Figure 5. Precision-Recall curves of different minutiae extraction algorithms on NIST SD27.
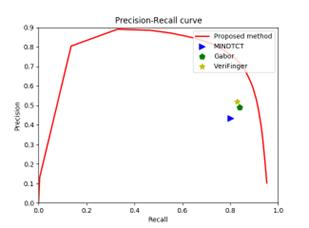
Figure 6. Precision-Recall curves of different minutiae extraction algorithms on FVC 2004 database set A.
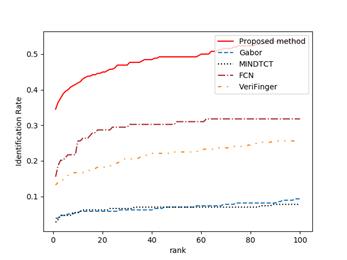
Figure 7. Identification performance (CMC curves) of different minutiae extraction algorithms on NIST SD27.
4. Conclusion
This research leverages advancements in deep learning to address and optimize the limitations of existing fingerprint recognition algorithms. By refining various steps and angles of the recognition process, the pre-trained deep learning models demonstrated several key improvements: they provide more stable estimation results, exhibit enhanced recognition capabilities for high-noise images, significantly accelerate retrieval speed, and maintain the advantage of being fully differentiable. These attributes underscore the potential for deep learning to continue driving progress in the field of fingerprint recognition. The newly introduced algorithms—such as the Faster R-CNN-based pose estimation, ConvNet-based direction field estimation, FingerNet-based minutiae extraction, and DeepPrint-based minutiae matching—have all shown varying degrees of improvement in accuracy and reduction of deviation compared to previous methods. However, there remain areas for further enhancement, particularly in improving recognition accuracy for high-noise images, refining the directional field recognition by further subdividing directional field types, developing more accurate segmentation methods, and strengthening the ability to detect and identify fake fingerprints. Moving forward, ongoing advancements in these areas will be crucial in further advancing the effectiveness and reliability of fingerprint recognition technology based on deep learning.
References
[1]. Jain A K Maltoni D Maio D and Prabhakar S. (2003). Handbook of Fingerprint Recognition. Springer New York
[2]. Grew N. (1984). The description and use of the pores in the skin of the bands and feet, by the learned and ingenious Nehemiah Grew MD. Fellow of the College of physicians and of the Royal Society Philosophical Transactions of the Royal Society of London, 14(159), 566-567 7
[3]. Hazarika P Russell D A. (2012). Advances in fingerprint analysis. Angewandte Chemie International Edition, 51(15), 3524-3531
[4]. Henry E R. (1928). Classification and uses of finger prints. HM Stationery office, 12-18
[5]. Fiumara G Flanagan P Schwarz M et al. (2018). NIST special database. 301 Gaithersburg MD USA, 13
[6]. Ouyang J Feng J Lu J et al. (2017). Fingerprint pose estimation based on faster R-CNN. IEEE International Joint Conference on Biometrics, 268-276
[7]. Cao K Jain A K. (2015). Latent orientation field estimation via convolutional neural network. International Conference on Biometrics, 349-356
[8]. Tang Y Gao F Feng J et al. (2017). FingerNet: An unified deep network for fingerprint minutiae extraction. IEEE International Joint Conference on Biometrics, 108-116
[9]. Pan Z Duan Y Guan X et al. (2024). Latent Fingerprint Matching via Dense Minutia Descriptor. arXiv preprint 2405.01199
[10]. Gu S Feng J Lu J et al. (2022). Latent fingerprint indexing: Robust representation and adaptive candidate list. IEEE Transactions on Information Forensics and Security, 17, 908-923
[11]. Su Y Feng J Zhou J. (2016). Fingerprint indexing with pose constraint. Pattern Recognition, 54, 1-13
[12]. Cao K Liu E Jain A K. (2014). Segmentation and enhancement of latent fingerprints: A coarse to fine ridgestructure dictionary. IEEE transactions on pattern analysis and machine intelligence 36(9), 1847-1859
[13]. Feng J Zhou J Jain A K. (2012). Orientation field estimation for latent fingerprint enhancement. IEEE transactions on pattern analysis and machine intelligence, 35(4), 925-940
[14]. Yang X Feng J Zhou J. (2014). Localized dictionaries based orientation field estimation for latent fingerprints. IEEE transactions on pattern analysis and machine intelligence, 36(5), 955-969
Cite this article
Yang,L. (2024). Advancements in Fingerprint Recognition Through Deep Learning: A Comprehensive Analysis of Novel Algorithms. Applied and Computational Engineering,105,1-8.
Data availability
The datasets used and/or analyzed during the current study will be available from the authors upon reasonable request.
Disclaimer/Publisher's Note
The statements, opinions and data contained in all publications are solely those of the individual author(s) and contributor(s) and not of EWA Publishing and/or the editor(s). EWA Publishing and/or the editor(s) disclaim responsibility for any injury to people or property resulting from any ideas, methods, instructions or products referred to in the content.
About volume
Volume title: Proceedings of CONF-MLA 2024 Workshop: Neural Computing and Applications
© 2024 by the author(s). Licensee EWA Publishing, Oxford, UK. This article is an open access article distributed under the terms and
conditions of the Creative Commons Attribution (CC BY) license. Authors who
publish this series agree to the following terms:
1. Authors retain copyright and grant the series right of first publication with the work simultaneously licensed under a Creative Commons
Attribution License that allows others to share the work with an acknowledgment of the work's authorship and initial publication in this
series.
2. Authors are able to enter into separate, additional contractual arrangements for the non-exclusive distribution of the series's published
version of the work (e.g., post it to an institutional repository or publish it in a book), with an acknowledgment of its initial
publication in this series.
3. Authors are permitted and encouraged to post their work online (e.g., in institutional repositories or on their website) prior to and
during the submission process, as it can lead to productive exchanges, as well as earlier and greater citation of published work (See
Open access policy for details).
References
[1]. Jain A K Maltoni D Maio D and Prabhakar S. (2003). Handbook of Fingerprint Recognition. Springer New York
[2]. Grew N. (1984). The description and use of the pores in the skin of the bands and feet, by the learned and ingenious Nehemiah Grew MD. Fellow of the College of physicians and of the Royal Society Philosophical Transactions of the Royal Society of London, 14(159), 566-567 7
[3]. Hazarika P Russell D A. (2012). Advances in fingerprint analysis. Angewandte Chemie International Edition, 51(15), 3524-3531
[4]. Henry E R. (1928). Classification and uses of finger prints. HM Stationery office, 12-18
[5]. Fiumara G Flanagan P Schwarz M et al. (2018). NIST special database. 301 Gaithersburg MD USA, 13
[6]. Ouyang J Feng J Lu J et al. (2017). Fingerprint pose estimation based on faster R-CNN. IEEE International Joint Conference on Biometrics, 268-276
[7]. Cao K Jain A K. (2015). Latent orientation field estimation via convolutional neural network. International Conference on Biometrics, 349-356
[8]. Tang Y Gao F Feng J et al. (2017). FingerNet: An unified deep network for fingerprint minutiae extraction. IEEE International Joint Conference on Biometrics, 108-116
[9]. Pan Z Duan Y Guan X et al. (2024). Latent Fingerprint Matching via Dense Minutia Descriptor. arXiv preprint 2405.01199
[10]. Gu S Feng J Lu J et al. (2022). Latent fingerprint indexing: Robust representation and adaptive candidate list. IEEE Transactions on Information Forensics and Security, 17, 908-923
[11]. Su Y Feng J Zhou J. (2016). Fingerprint indexing with pose constraint. Pattern Recognition, 54, 1-13
[12]. Cao K Liu E Jain A K. (2014). Segmentation and enhancement of latent fingerprints: A coarse to fine ridgestructure dictionary. IEEE transactions on pattern analysis and machine intelligence 36(9), 1847-1859
[13]. Feng J Zhou J Jain A K. (2012). Orientation field estimation for latent fingerprint enhancement. IEEE transactions on pattern analysis and machine intelligence, 35(4), 925-940
[14]. Yang X Feng J Zhou J. (2014). Localized dictionaries based orientation field estimation for latent fingerprints. IEEE transactions on pattern analysis and machine intelligence, 36(5), 955-969