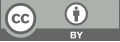
Research on Detection Methods for Text Generated by Large Language Models Based on Multi-Model Ensemble
- 1 Business-intelligence of Oriental Nations Corporation Ltd, Beijing, China
- 2 Goodwill E-Health Info Corporation Ltd, Beijing, China
* Author to whom correspondence should be addressed.
Abstract
The rapid development of Large Language Models (LLMs) has made their generated text almost indistinguishable from human writing, posing significant challenges to traditional human-machine recognition techniques. This paper proposes a detection method based on multi-model ensemble to accurately identify text generated by LLMs. Firstly, a large-scale, diverse, and heterogeneous dataset is constructed, covering student writings and texts generated by models such as GPT-3, GPT-2, CTRL, and XLM. Then, a multifaceted detection framework integrating linear models, deep learning models, and pre-trained language models is designed. The linear model utilizes an argumentative essay dataset (DAIGT V2 Train Dataset) similar in distribution to the competition dataset, combined with adaptive BPE tokenization, N-Gram, and TF-IDF features. It employs Multinomial Naive Bayes and SGDClassifier to train classifiers that capture shallow statistical features of the text. The deep learning model fine-tunes the DeBERTa-v3-small model on large-scale datasets (Pile, Ultra, Human vs. LLM Text Corpus) to learn deep semantic representations of the text. The pre-trained language model introduces a fine-tuned DistilRoBERTa model, enhancing detection capabilities using third-party datasets. Finally, the above models are integrated through a weighted average strategy, significantly improving the generalization and robustness of the detection results. Experimental results show that this method achieved a score of 0.967466 in the Kaggle competition, earning a silver medal and outperforming any single model. The study demonstrates the effectiveness of multi-source data and multi-model ensemble in detecting LLM-generated text, providing new ideas and practical references for research in this field.
Keywords
Large Language Models, text detection, heterogeneous dataset, deep learning.
[1]. Brown, T. B., Mann, B., Ryder, N., Subbiah, M., Kaplan, J., Dhariwal, P., ... & Amodei, D. (2020). Language Models are Few-Shot Learners. In Advances in Neural Information Processing Systems (Vol. 33, pp. 1877-1901). Curran Associates, Inc.
[2]. Uchendu, A., Suresh, H., Xu, W., & Lee, S. (2020). Authorship Attribution for Neural Text Generation. In Proceedings of the 2020 Conference on Empirical Methods in Natural Language Processing (EMNLP) (pp. 8384-8395). Association for Computational Linguistics.
[3]. Zellers, R., Holtzman, A., Bisk, Y., Farhadi, A., & Choi, Y. (2019). Defending Against Neural Fake News. In Advances in Neural Information Processing Systems (Vol. 32, pp. 9051-9062). Curran Associates, Inc.
[4]. DAIGT V2 Train Dataset. (2022). Kaggle. Available at: https://www.kaggle.com/datasets/thedrcat/daigt-v2-train-dataset
[5]. Gao, L., Biderman, S., Black, S., Golding, L., Hoppe, T., Foster, C., ... & Leahy, C. (2020). The Pile: An 800GB Dataset of Diverse Text for Language Modeling. arXiv preprint arXiv:2101.00027. Available at: https://www.kaggle.com/datasets/canming/piles-and-ultra-data
[6]. Human vs. LLM Text Corpus. (2023). Kaggle. Available at: https://www.kaggle.com/datasets/starblasters8/human-vs-llm-text-corpus
[7]. Kudo, T., & Richardson, J. (2018). SentencePiece: A simple and language independent subword tokenizer and detokenizer for Neural Text Processing. In Proceedings of the 2018 Conference on Empirical Methods in Natural Language Processing: System Demonstrations (pp. 66-71). Association for Computational Linguistics.
[8]. He, P., Liu, X., Gao, J., & Chen, W. (2021). DeBERTa: Decoding-enhanced BERT with Disentangled Attention. In International Conference on Learning Representations (ICLR) 2021. Available at: https://openreview.net/forum?id=XPZIaotutsD
[9]. Sanh, V., Debut, L., Chaumond, J., & Wolf, T. (2019). DistilBERT, a distilled version of BERT: smaller, faster, cheaper and lighter. arXiv preprint arXiv:1910.01108.
Cite this article
Tian,L.;Jiang,N. (2024). Research on Detection Methods for Text Generated by Large Language Models Based on Multi-Model Ensemble. Applied and Computational Engineering,106,59-67.
Data availability
The datasets used and/or analyzed during the current study will be available from the authors upon reasonable request.
Disclaimer/Publisher's Note
The statements, opinions and data contained in all publications are solely those of the individual author(s) and contributor(s) and not of EWA Publishing and/or the editor(s). EWA Publishing and/or the editor(s) disclaim responsibility for any injury to people or property resulting from any ideas, methods, instructions or products referred to in the content.
About volume
Volume title: Proceedings of the 2nd International Conference on Machine Learning and Automation
© 2024 by the author(s). Licensee EWA Publishing, Oxford, UK. This article is an open access article distributed under the terms and
conditions of the Creative Commons Attribution (CC BY) license. Authors who
publish this series agree to the following terms:
1. Authors retain copyright and grant the series right of first publication with the work simultaneously licensed under a Creative Commons
Attribution License that allows others to share the work with an acknowledgment of the work's authorship and initial publication in this
series.
2. Authors are able to enter into separate, additional contractual arrangements for the non-exclusive distribution of the series's published
version of the work (e.g., post it to an institutional repository or publish it in a book), with an acknowledgment of its initial
publication in this series.
3. Authors are permitted and encouraged to post their work online (e.g., in institutional repositories or on their website) prior to and
during the submission process, as it can lead to productive exchanges, as well as earlier and greater citation of published work (See
Open access policy for details).