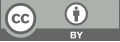
Wind Power Prediction Based on LSTM and Self-Attention Mechanism
- 1 Harbin Institute of Technology, Shenzhen, HIT Campus of University Town of Shenzhen, Shenzhen, China
* Author to whom correspondence should be addressed.
Abstract
With the intensification of global climate change and energy crises, wind energy, as a clean and renewable energy source, has gradually become a crucial component in the energy sector. However, the intermittent and unstable nature of wind power generation poses significant challenges to accurately predicting the power output of wind turbines. This study proposes a wind power prediction model combining Long Short-Term Memory (LSTM) networks and Self-Attention mechanisms. LSTM net- works effectively capture long-term dependencies in time series through their gat- ing mechanisms, while the Self-Attention mechanism dynamically adjusts attention to critical time steps, further enhancing prediction accuracy. Experimental validation on real-world wind power datasets demonstrates that the LSTM + Attention model outperforms traditional RNN and LSTM models in terms of training loss, validation loss, and prediction accuracy, particularly in reducing prediction errors and improving accuracy. The results indicate that the LSTM model integrated with Self-Attention ef- fectively addresses complex nonlinear features in wind power prediction, enhancing both generalization capability and prediction precision. This model provides an ef- fective solution for wind power prediction and holds significant application value for optimizing grid dispatch and management, as well as improving the competitiveness of wind energy in the energy market.
Keywords
wind power generation, power prediction, long short-term memory (lstm), self-attention mechanism, deep learning
[1]. Bri-Mathias Hodge, Austin Zeiler, Duncan Brooks, Gary Blau, Joseph Pekny, and Gintaras Reklatis. Improved wind power forecasting with arima models. In Computer aided chemical engineering, volume 29, pages 1789–1793. Elsevier, 2011.
[2]. Guoqiang Zhang, B Eddy Patuwo, and Michael Y Hu. Forecasting with artificial neural net- works:: The state of the art. International journal of forecasting, 14(1):35–62, 1998.
[3]. Ian Goodfellow. Deep learning, volume 196. MIT press, 2016.
[4]. Gregor Giebel and George Kariniotakis. Wind power forecasting—a review of the state of the art. Renewable energy forecasting, pages 59–109, 2017.
[5]. Xiaochen Wang, Peng Guo, and Xiaobin Huang. A review of wind power forecasting models. Energy Procedia, 12:770–778, 2011.
[6]. Shahram Hanifi, Xiaolei Liu, Zi Lin, and Saeid Lotfian. A critical review of wind power fore- casting methods—past, present and future. Energies, 13(15):3764, 2020.
[7]. Ulrich Focken, Matthias Lange, and Hans-Peter Waldl. Previento-a wind power prediction system with an innovative upscaling algorithm. In Proceedings of the European Wind Energy Confer- ence, Copenhagen, Denmark, volume 276, 2001.
[8]. Umut Firat, Seref Naci Engin, Murat Saraclar, and Aysin Baytan Ertuzun. Wind speed forecasting based on second order blind identification and autoregressive model. In 2010 Ninth International Conference on Machine Learning and Applications, pages 686–691. IEEE, 2010.
[9]. Zi Lin, Xiaolei Liu, and Maurizio Collu. Wind power prediction based on high-frequency scada data along with isolation forest and deep learning neural networks. International Journal of Electrical Power & Energy Systems, 118:105835, 2020.
[10]. Pankaj Malhotra, Anusha Ramakrishnan, Gaurangi Anand, Lovekesh Vig, Puneet Agarwal, and Gautam Shroff. Lstm-based encoder-decoder for multi-sensor anomaly detection. arXiv preprint arXiv:1607.00148, 2016.
[11]. Jinhua Zhang, Jie Yan, David Infield, Yongqian Liu, and Fue-sang Lien. Short-term forecasting and uncertainty analysis of wind turbine power based on long short-term memory network and gaussian mixture model. Applied Energy, 241:229–244, 2019.
[12]. S Hochreiter. Long short-term memory. Neural Computation MIT-Press, 1997.
[13]. Kyunghyun Cho. On the properties of neural machine translation: Encoder-decoder approaches. arXiv preprint arXiv:1409.1259, 2014.
[14]. Xin Liu, Luoxiao Yang, and Zijun Zhang. Short-term multi-step ahead wind power predictions based on a novel deep convolutional recurrent network method. IEEE Transactions on Sustain- able Energy, 12(3):1820–1833, 2021.
[15]. Yoshua Bengio, Patrice Simard, and Paolo Frasconi. Learning long-term dependencies with gradient descent is difficult. IEEE transactions on neural networks, 5(2):157–166, 1994.
[16]. Junyoung Chung, Caglar Gulcehre, KyungHyun Cho, and Yoshua Bengio. Empirical evaluation of gated recurrent neural networks on sequence modeling. arXiv preprint arXiv:1412.3555, 2014.
[17]. Felix A Gers, Jürgen Schmidhuber, and Fred Cummins. Learning to forget: Continual prediction with lstm. Neural computation, 12(10):2451–2471, 2000.
[18]. I Sutskever. Sequence to sequence learning with neural networks. arXiv preprint arXiv:1409.3215, 2014.
[19]. George Zerveas, Srideepika Jayaraman, Dhaval Patel, Anuradha Bhamidipaty, and Carsten Eick- hoff. A transformer-based framework for multivariate time series representation learning. In Pro- ceedings of the 27th ACM SIGKDD conference on knowledge discovery & data mining, pages 2114–2124, 2021.
[20]. A Vaswani. Attention is all you need. Advances in Neural Information Processing Systems, 2017.
[21]. Md Rasel Sarkar, Sreenatha G Anavatti, Tanmoy Dam, Mahardhika Pratama, and Berlian Al Kindhi. Enhancing wind power forecast precision via multi-head attention transformer: An investigation on single-step and multi-step forecasting. In 2023 International Joint Conference on Neural Networks (IJCNN), pages 1–8. IEEE, 2023.
[22]. Hao Zhang, Jie Yan, Yongqian Liu, Yongqi Gao, Shuang Han, and Li Li. Multi-source and temporal attention network for probabilistic wind power prediction. IEEE Transactions on Sus- tainable Energy, 12(4):2205–2218, 2021.
[23]. Bryan Lim, Sercan Ö Arık, Nicolas Loeff, and Tomas Pfister. Temporal fusion transformers for interpretable multi-horizon time series forecasting. International Journal of Forecasting, 37(4):1748–1764, 2021.
Cite this article
Yang,Y. (2025). Wind Power Prediction Based on LSTM and Self-Attention Mechanism. Applied and Computational Engineering,141,30-38.
Data availability
The datasets used and/or analyzed during the current study will be available from the authors upon reasonable request.
Disclaimer/Publisher's Note
The statements, opinions and data contained in all publications are solely those of the individual author(s) and contributor(s) and not of EWA Publishing and/or the editor(s). EWA Publishing and/or the editor(s) disclaim responsibility for any injury to people or property resulting from any ideas, methods, instructions or products referred to in the content.
About volume
Volume title: Proceedings of the 3rd International Conference on Mechatronics and Smart Systems
© 2024 by the author(s). Licensee EWA Publishing, Oxford, UK. This article is an open access article distributed under the terms and
conditions of the Creative Commons Attribution (CC BY) license. Authors who
publish this series agree to the following terms:
1. Authors retain copyright and grant the series right of first publication with the work simultaneously licensed under a Creative Commons
Attribution License that allows others to share the work with an acknowledgment of the work's authorship and initial publication in this
series.
2. Authors are able to enter into separate, additional contractual arrangements for the non-exclusive distribution of the series's published
version of the work (e.g., post it to an institutional repository or publish it in a book), with an acknowledgment of its initial
publication in this series.
3. Authors are permitted and encouraged to post their work online (e.g., in institutional repositories or on their website) prior to and
during the submission process, as it can lead to productive exchanges, as well as earlier and greater citation of published work (See
Open access policy for details).