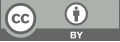
Design of Reward Functions for Autonomous Driving Based on Reinforcement Learning: Balancing Safety and Efficiency
- 1 International School, Beijing University of Posts and Telecommunications
* Author to whom correspondence should be addressed.
Abstract
Autonomous driving, leveraging artificial intelligence and reinforcement learning (RL), has made significant strides in improving traffic efficiency and safety. However, current RL-based approaches often focus on single-objective optimization, such as maximizing either efficiency or safety. In real-world driving, multiple conflicting objectives—such as safety, efficiency, and comfort—must be balanced simultaneously, which remains underexplored. This paper proposes a multi-objective reward function design to balance safety and efficiency in autonomous driving. Using the Proximal Policy Optimization (PPO) algorithm, we train seven autonomous driving models with varying collision penalty strategies in the MetaDrive simulation environment. The results show that dynamic collision penalties outperform fixed penalties in balancing safety and efficiency, with Model 5 achieving the best overall performance. Despite this, all models underperform in left-turn scenarios, highlighting the need for further optimization in lateral control. This work provides insights into effective reward design for multi-objective reinforcement learning in autonomous driving.
Keywords
Reinforcement Learning, autonomous driving, reward function, safety, efficiency
[1]. Russell, S. J., & Norvig, P. (2021). Artificial intelligence: a modern approach, 4th US ed. University of California, Berkeley.
[2]. Bansal, M., Krizhevsky, A., & Ogale, A. (2018). Chauffeurnet: Learning to drive by imitating the best and synthesizing the worst. arXiv preprint arXiv:1812.03079.
[3]. Geiger, A., Lenz, P., Stiller, C., & Urtasun, R. (2013). Vision meets robotics: The kitti dataset. The International Journal of Robotics Research, 32(11), 1231-1237.
[4]. Schwarting, W., Pierson, A., Alonso-Mora, J., Karaman, S., & Rus, D. (2019). Social behavior for autonomous vehicles. Proceedings of the National Academy of Sciences, 116(50), 24972-24978.
[5]. Kong, J., Pfeiffer, M., Schildbach, G., & Borrelli, F. (2015, June). Kinematic and dynamic vehicle models for autonomous driving control design. In 2015 IEEE intelligent vehicles symposium (IV) (pp. 1094-1099). IEEE.
[6]. Kiran, B. R., Sobh, I., Talpaert, V., Mannion, P., Al Sallab, A. A., Yogamani, S., & Pérez, P. (2021). Deep reinforcement learning for autonomous driving: A survey. IEEE Transactions on Intelligent Transportation Systems, 23(6), 4909-4926.
[7]. HAMMA, R., & BOUMARAF, M. (2023). Applying Deep Reinforcement Learning for Autonomous Driving in CARLA Simulator (Doctoral dissertation).
[8]. Lowe, R., Wu, Y. I., Tamar, A., Harb, J., Pieter Abbeel, O., & Mordatch, I. (2017). Multi-agent actor-critic for mixed cooperative-competitive environments. Advances in neural information processing systems, 30.
[9]. Haarnoja, T., Zhou, A., Abbeel, P., & Levine, S. (2018, July). Soft actor-critic: Off-policy maximum entropy deep reinforcement learning with a stochastic actor. In International conference on machine learning (pp. 1861-1870). PMLR.
[10]. Andrychowicz, M., Wolski, F., Ray, A., Schneider, J., Fong, R., Welinder, P., ... & Zaremba, W. (2017). Hindsight experience replay. Advances in neural information processing systems, 30.
[11]. Ng, A. Y., Harada, D., & Russell, S. (1999, June). Policy invariance under reward transformations: Theory and application to reward shaping. In Icml (Vol. 99, pp. 278-287).
[12]. Shalev-Shwartz, S. (2017). On a formal model of safe and scalable self-driving cars. arXiv preprint arXiv:1708.06374.
[13]. Taubman-Ben-Ari, O., Mikulincer, M., & Gillath, O. (2004). The multidimensional driving style inventory—scale construct and validation. Accident Analysis & Prevention, 36(3), 323-332.
[14]. Ziegler, J., Bender, P., Schreiber, M., Lategahn, H., Strauss, T., Stiller, C., ... & Zeeb, E. (2014). Making bertha drive—an autonomous journey on a historic route. IEEE Intelligent transportation systems magazine, 6(2), 8-20.
[15]. Bojarski, M. (2016). End to end learning for self-driving cars. arXiv preprint arXiv:1604.07316.
[16]. Ohn-Bar, E., Prakash, A., Behl, A., Chitta, K., & Geiger, A. (2020). Learning situational driving. In Proceedings of the IEEE/CVF Conference on Computer Vision and Pattern Recognition (pp. 11296-11305).
Cite this article
Zhang,W. (2025). Design of Reward Functions for Autonomous Driving Based on Reinforcement Learning: Balancing Safety and Efficiency. Applied and Computational Engineering,146,9-22.
Data availability
The datasets used and/or analyzed during the current study will be available from the authors upon reasonable request.
Disclaimer/Publisher's Note
The statements, opinions and data contained in all publications are solely those of the individual author(s) and contributor(s) and not of EWA Publishing and/or the editor(s). EWA Publishing and/or the editor(s) disclaim responsibility for any injury to people or property resulting from any ideas, methods, instructions or products referred to in the content.
About volume
Volume title: Proceedings of SEML 2025 Symposium: Machine Learning Theory and Applications
© 2024 by the author(s). Licensee EWA Publishing, Oxford, UK. This article is an open access article distributed under the terms and
conditions of the Creative Commons Attribution (CC BY) license. Authors who
publish this series agree to the following terms:
1. Authors retain copyright and grant the series right of first publication with the work simultaneously licensed under a Creative Commons
Attribution License that allows others to share the work with an acknowledgment of the work's authorship and initial publication in this
series.
2. Authors are able to enter into separate, additional contractual arrangements for the non-exclusive distribution of the series's published
version of the work (e.g., post it to an institutional repository or publish it in a book), with an acknowledgment of its initial
publication in this series.
3. Authors are permitted and encouraged to post their work online (e.g., in institutional repositories or on their website) prior to and
during the submission process, as it can lead to productive exchanges, as well as earlier and greater citation of published work (See
Open access policy for details).