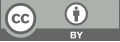
Sentiment Prediction by a Classifier
- 1 University of Melbourne
* Author to whom correspondence should be addressed.
Abstract
In real life, there is far more unprocessed data than labeled data, which brings a large amount of data that cannot be directly used for machine learning training. Based on the tweet dataset processed by Natural Language Processing (NLP), this paper uses a variety of machine learning models for training and comparison. Moreover, different performances are analyzed and discussed. Since labeled datasets are difficult to obtain, the use of supervised learning will be limited. However, the number of unlabeled datasets is very large, which can provide a continuous training set for machine learning. This paper conducted a comparative experiment on the effect of semi-supervised learning and obtained better results than supervised learning and unsupervised learning. The experiments in this paper prove that semi-supervised learning can effectively use unlabeled data and train machine learning models.
Keywords
machine learning, sentiment prediction, KNN, Gaussian Bayes, logistic regression
[1]. Zhang, S., Li, X., Zong, M., Zhu, X. and Wang, R. Efficient kNN classification with different numbers of nearest neighbors. IEEE transactions on neural networks and learning systems 29(5), 1774–1785 (2017).
[2]. Ontivero-Ortega, M., Lage-Castellanos, A., Valente, G., Goebel, R. and Valdes-Sosa, M. Fast Gaussian Naïve Bayes for searchlight classification analysis. Neuroimage 163, 471–479 (2017).
[3]. Komarek, P. Logistic regression for data mining and high-dimensional classification. Carnegie Mellon University (2004).
[4]. Eisenstein, J., O’Connor, B., Smith, N. A. and Xing, E. P. A latent variable model for geographic lexical variation. In Proceedings of the 2010 Conference on Empirical Methods in Natural Language Processing (EMNLP 2010), Cambridge, USA, 1277–1287 (2010).
[5]. Blodgett, S. L., Green, L., and O’Connor, B. Demographic dialectal variation in social media: A case study of African-American English. In Proceedings of the 2016 Conference on Empirical Methods in Natural Language Processing, Austin, Texas, Association for Computational Linguistics, 1119–1130 (2016).
[6]. Deri, A. and Knight, K. How to make a frenemy: Multitape FSTs for portmanteau generation. In Human Language Technologies: The2015 Annual Conference of the North American Conference of the North American Chapter of the ACL, Denver, USA, 206–210 (2015).
[7]. Das, K. and Neuramanteau, S. A neural network ensemble model for lexical blends. In Proceedings of The 8th International Joint Conference on Natural Language Processing, Taipei, Table 8. Test for overfitting. Semi-supervised, K=40 Accuracy F1 Prediction of development set 0.69125 0.69083 Prediction of training set 0.85259 0.85248 Taiwan, 576–583 (2017).
[8]. Cook, P. and Stevenson, S. Automatically identifying the source words of lexical blends in English. Computational Linguistics 36(1), 129–149 (2010).
[9]. Choudhary, R. and Gianey, H. K. Comprehensive review on supervised machine learning algorithms. In 2017 International Conference on Machine Learning and Data Science (MLDS), IEEE, 37-43 (2017).
[10]. Guo, G., Wang, H., Bell, D., Bi, Y. and Greer, K. KNN model-based approach in classification. In OTM Confederated International Conferences. On the Move to Meaningful Internet Systems. Springer, Berlin, Heidelberg, 986-996 (2003).
[11]. Singh, A., Thakur, N. and Sharma, A. A review of supervised machine learning algorithms. In 2016 3rd International Conference on Computing for Sustainable Global Development (INDIACom), IEEE, 1310-1315 (2016).
[12]. Van Engelen, J. E. and Hoos, H. H. A survey on semi-supervised learning. Machine Learning 109(2), 373-440 (2020).
[13]. Rosenberg, C., Hebert, M. and Schneiderman, H. Semi-supervised self-training of object detection models (2005).
[14]. Lee, D. H. Pseudo-label: The simple and efficient semi-supervised learning method for deep neural networks. In Workshop on challenges in representation learning, ICML 3(2), 896 (2013).
[15]. De Sousa Ribeiro, F., Calivá, F., Swainson, M., Gudmundsson, K., Leontidis, G. and Kollias, S. Deep bayesian self-training. Neural Computing and Applications 32(9), 4275-4291 (2020).
Cite this article
Ren,Y. (2023). Sentiment Prediction by a Classifier. Applied and Computational Engineering,8,18-25.
Data availability
The datasets used and/or analyzed during the current study will be available from the authors upon reasonable request.
Disclaimer/Publisher's Note
The statements, opinions and data contained in all publications are solely those of the individual author(s) and contributor(s) and not of EWA Publishing and/or the editor(s). EWA Publishing and/or the editor(s) disclaim responsibility for any injury to people or property resulting from any ideas, methods, instructions or products referred to in the content.
About volume
Volume title: Proceedings of the 2023 International Conference on Software Engineering and Machine Learning
© 2024 by the author(s). Licensee EWA Publishing, Oxford, UK. This article is an open access article distributed under the terms and
conditions of the Creative Commons Attribution (CC BY) license. Authors who
publish this series agree to the following terms:
1. Authors retain copyright and grant the series right of first publication with the work simultaneously licensed under a Creative Commons
Attribution License that allows others to share the work with an acknowledgment of the work's authorship and initial publication in this
series.
2. Authors are able to enter into separate, additional contractual arrangements for the non-exclusive distribution of the series's published
version of the work (e.g., post it to an institutional repository or publish it in a book), with an acknowledgment of its initial
publication in this series.
3. Authors are permitted and encouraged to post their work online (e.g., in institutional repositories or on their website) prior to and
during the submission process, as it can lead to productive exchanges, as well as earlier and greater citation of published work (See
Open access policy for details).