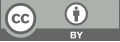
Classifying autism spectrum disorder using machine learning through ABIDE dataset
- 1 College of Information and Control Engineering, Xi’an University of Architecture and Technology, Xi’an, Shaanxi, 710054, China
- 2 College of Artificial Intelligence, Tianjin University of Science & Technology, Tian-jin, 300457, China
- 3 Design and Technology, Parsons School of Design, The New School, New York, 10003, USA
* Author to whom correspondence should be addressed.
Abstract
A neurodevelopmental disorder named autism spectrum disorder (ASD) is challenging to diagnose. The prevailing diagnostic manner is based merely on the behavioral measure-ment with a high tendency of misdiagnosis. People require an advanced method to make more quantitative diagnosis. In this paper, two deep learning architectures were explored with the machine learning methods. The Mixup method was used to augment the original functional Magnetic Resonance Imaging data. Features of the data extracted by two dif-ferent kinds of autoencoders which are Sparse Autoencoder and Variational Autoencoder were used as inputs of two deep neural networks functioning as classifiers respectively. The models can classify patients with ASD from typical control subjects with the accura-cy of 75.5% and 75.2% respectively, which outperformed the other state-of-the-art meth-od by 4.7% and 4.4%. The further significance of this project is to help develop our per-ception of the neurobiological foundation of the ASD.
Keywords
brain disorder, autism spectrum disorder, machine learning, deep neural network, autoen-coder
[1]. Nickel, R. E., & Huang-Storms, L. (2015, September 28). Early Identification of Young Children with Autism Spectrum Disorder. Indian Journal of Pediatrics. https://link.springer.com/article/10.1007/s12098-015-1894-0.
[2]. Baio, J., Wiggins, L., Christensen, D. L., Maenner, M. J., Daniels, J., Warren, Z., Kurzius-Spencer, M., Zahorodny, W., Robinson Rosenberg, C., White, T., Durkin, M. S., Imm, P., Nikolaou, L., Yeargin-Allsopp, M., Lee, L.-C., Harrington, R., Lopez, M., Fitzgerald, R. T., Hewitt, A., … Dowling, N. F. (2018, April 27). Prevalence of Autism Spectrum Disorder Among Children Aged 8 Years - Autism and Developmental Disabilities Monitoring Network, 11 Sites, United States, 2014. Morbidity and mortality weekly report. Surveillance summaries (Washington, D.C. : 2002). https://www.ncbi.nlm.nih.gov/pmc/articles/PMC5919599/.
[3]. Biswal, B., Yetkin, F.Z., Haughton, V.M., Hyde, J.S., (1995). Functional connectivity in the motor cortex of resting human brain using echo-planar MRI. Magn. Reason. Med. 34(4), 537-541.
[4]. Eslami, T., Mirjalili, V., Fong, A., Laird, A. R., & Saeed, F. (2019, January 1). ASD-DiagNet: A Hybrid Learning Approach for Detection of Autism Spectrum Disorder Using fMRI Data. Frontiers. https://www.frontiersin.org/articles/10.3389/fninf.2019.00070/full.
[5]. Yang, J., et al., (2010). Common SNPs explain a large proportion of the heritability for human height. Nat. Genet. 42(7), 565-569.
[6]. Just, M.A., Cherkassky, V.L., Buchweitz, A.,Keller, T.A., Mitchell, T.M., 2014. Identifying autism from neural representations of social interactions: neurocognitive markers of autism. PLoS ONE 9 (12), 1-22.
[7]. Craddock, R.C., Holtzheimer, P.E., Hu, X.P., Mayberg, H.S., (2009). Disease state prediction from resting state functional connectivity. Magn. Reason. Med. 62 (6), 1619-1628.
[8]. Nielsen, J. A., Zielinski, B. A., Fletcher, P. T., Alexander, A. L., Lange, N., Bigler, E. D., ... & Anderson, J. S. (2013). Multisite functional connectivity MRI classification of autism: ABIDE results. Frontiers in human neuroscience, 7, 599.
[9]. Heinsfeld, A. S., Franco, A. R., Craddock, R. C., Buchweitz, A., & Meneguzzi, F. (2018). Identification of autism spectrum disorder using deep learning and the ABIDE dataset. NeuroImage:
[10]. Plis, S.M., et al., (2014). Deep learning for neuroimaging: a validation study. Front. Neurosci. 8 (August), 229.
[11]. Almuqhim, F., & Saeed, F. (2021). ASD-SAENet: A Sparse Autoencoder, and Deep-Neural Network Model for Detecting Autism Spectrum Disorder (ASD) Using fMRI Data. Frontiers in computational neuroscience, 15, 654315. https://doi.org/10.3389/fncom.2021.654315
[12]. Zhan Y, Wei J, Liang J, Xu X, He R, Robbins TW, Wang Z. Diagnostic Classification for Human Autism and Obsessive-Compulsive Disorder Based on Machine Learning From a Primate Genetic Model. Am J Psychiatry. 2021 Jan 1;178(1):65-76. doi: 10.1176/appi.ajp.2020.19101091. Epub 2020 Jun 16. PMID: 32539526.
[13]. Liang X, Wang J, Yan C, Shu N, Xu K, Gong G, He Y. Effects of different correlation metrics and preprocessing factors on small-world brain functional networks: a resting-state functional MRI study. PLoS One. 2012;7(3):e32766. doi: 10.1371/journal.pone.0032766. Epub 2012 Mar 6. PMID: 22412922; PMCID: PMC3295769.
[14]. Baggio HC, Sala-Llonch R, Segura B, Marti MJ, Valldeoriola F, Compta Y, Tolosa E, Junqué C. Functional brain networks and cognitive deficits in Parkinson's disease. Hum Brain Mapp. 2014 Sep;35(9):4620-34. doi: 10.1002/hbm.22499. Epub 2014 Mar 17. PMID: 24639411; PMCID: PMC6869398.
[15]. Zhang, Y., Zhang, H., Chen, X., Lee, S. W., & Shen, D. (2017). Hybrid high-order functional connectivity networks using resting-state functional MRI for mild cognitive impairment diagnosis. Scientific reports, 7(1), 1-15.
[16]. Eslami, T., and Saeed, F. (2019). “Auto-ASD-network: a technique based on deep learning and support vector machines for diagnosing autism spectrum disorder using fMRI data,” in Proceedings of ACM Conference on Bioinformatics,Computational Biology, and Health Informatics (Niagara Falls, NY: ACM).
[17]. Zhang, H., Cisse, M., Dauphin, Y. N., & Lopez-Paz, D. (2017). mixup: Beyond empirical risk minimization. arXiv preprint arXiv:1710.09412.
[18]. Ioffe, S. & Szegedy, C.. (2015). Batch Normalization: Accelerating Deep Network Training by Reducing Internal Covariate Shift. Proceedings of the 32nd International Conference on Machine Learning, in Proceedings of Machine Learning Research 37:448-456 Available from http://proceedings.mlr.press/v37/ioffe15.html .
[19]. D.P. Kingma and J.L. Ba, Adam: A Method for Stochastic Optimization. San Diego: The International Conference on Learning Representations (ICLR), 2015.
[20]. Just, M. A., Cherkassky, V. L., Keller, T. A., Kana, R. K., & Minshew, N. J. (2007). Functional and anatomical cortical underconnectivity in autism: evidence from an FMRI study of an executive function task and corpus callosum morphometry. Cerebral cortex, 17(4), 951-961.
Cite this article
Han,W.;Shao,W.;Wang,Y. (2023). Classifying autism spectrum disorder using machine learning through ABIDE dataset. Applied and Computational Engineering,2,31-44.
Data availability
The datasets used and/or analyzed during the current study will be available from the authors upon reasonable request.
Disclaimer/Publisher's Note
The statements, opinions and data contained in all publications are solely those of the individual author(s) and contributor(s) and not of EWA Publishing and/or the editor(s). EWA Publishing and/or the editor(s) disclaim responsibility for any injury to people or property resulting from any ideas, methods, instructions or products referred to in the content.
About volume
Volume title: Proceedings of the 4th International Conference on Computing and Data Science (CONF-CDS 2022)
© 2024 by the author(s). Licensee EWA Publishing, Oxford, UK. This article is an open access article distributed under the terms and
conditions of the Creative Commons Attribution (CC BY) license. Authors who
publish this series agree to the following terms:
1. Authors retain copyright and grant the series right of first publication with the work simultaneously licensed under a Creative Commons
Attribution License that allows others to share the work with an acknowledgment of the work's authorship and initial publication in this
series.
2. Authors are able to enter into separate, additional contractual arrangements for the non-exclusive distribution of the series's published
version of the work (e.g., post it to an institutional repository or publish it in a book), with an acknowledgment of its initial
publication in this series.
3. Authors are permitted and encouraged to post their work online (e.g., in institutional repositories or on their website) prior to and
during the submission process, as it can lead to productive exchanges, as well as earlier and greater citation of published work (See
Open access policy for details).