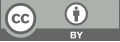
Reinforcement learning methods in board and MOBA games
- 1 University of California, Davis. 1 Shields Ave, Davis, CA 95616
* Author to whom correspondence should be addressed.
Abstract
This article provided an introduction of applying reinforcement learning to games, including board games and video games like Backgammon, Go, and Dota2. The reason for choosing reinforcement learning to solve game problems was analyzed. The article also reviewed the reinforcement learning technique and introduced two optimizing learning methods, Temporal Difference learning and Q learning. Then, three important cases of using reinforcement learning to reach high level game skill, TD-gammon, AlphaGo, and OpenAI Five, were introduced. In the end, the future possibility of applying reinforcement learning in broader way was analyzed.
Keywords
board games, video games, reinforcement learning, Backgammon, Go, Dota2.
[1]. Sutton, Richard S. "Learning to predict by the methods of temporal differences." Machine learning 3.1 (1988): 9-44.
[2]. Tesauro, Gerald. "Temporal difference learning and TD-Gammon." Communications of the ACM 38.3 (1995): 58-68.
[3]. Tesauro, Gerald. "Neurogammon: A neural-network backgammon program." 1990 IJCNN international joint conference on neural networks. IEEE, 1990.
[4]. Sutton, Richard S., and Andrew G. Barto. Reinforcement learning: An introduction. MIT press, 2018.
[5]. Tesauro, Gerald. "TD-Gammon, a self-teaching backgammon program, achieves master-level play." Neural computation 6.2 (1994): 215-219.
[6]. Berner, Christopher, et al. "Dota 2 with large scale deep reinforcement learning." arXiv preprint arXiv:1912.06680(2019).
[7]. Tom M. Mitchell. “Machine Learning.” (1997).
[8]. Justesen, Niels, et al. "Deep learning for video game playing." IEEE Transactions on Games 12.1 (2019): 1-20.
[9]. Wang, Fei-Yue, et al. "Where does AlphaGo go: From church-turing thesis to AlphaGo thesis and beyond." IEEE/CAA Journal of Automatica Sinica 3.2 (2016): 113-120.
[10]. Silver, David, et al. "Mastering the game of go without human knowledge." nature 550.7676 (2017): 354-359.
[11]. Chaslot, Guillaume, et al. "Monte-carlo tree search: A new framework for game ai." Proceedings of the AAAI Conference on Artificial Intelligence and Interactive Digital Entertainment. Vol. 4. No. 1. 2008.
[12]. Schulman, John, et al. "Proximal policy optimization algorithms." arXiv preprint arXiv:1707.06347 (2017).
[13]. Zia, Tehseen, and Usman Zahid. "Long short-term memory recurrent neural network architectures for Urdu acoustic modeling." International Journal of Speech Technology 22.1 (2019): 21-30
Cite this article
Chai,H. (2023). Reinforcement learning methods in board and MOBA games. Applied and Computational Engineering,2,105-112.
Data availability
The datasets used and/or analyzed during the current study will be available from the authors upon reasonable request.
Disclaimer/Publisher's Note
The statements, opinions and data contained in all publications are solely those of the individual author(s) and contributor(s) and not of EWA Publishing and/or the editor(s). EWA Publishing and/or the editor(s) disclaim responsibility for any injury to people or property resulting from any ideas, methods, instructions or products referred to in the content.
About volume
Volume title: Proceedings of the 4th International Conference on Computing and Data Science (CONF-CDS 2022)
© 2024 by the author(s). Licensee EWA Publishing, Oxford, UK. This article is an open access article distributed under the terms and
conditions of the Creative Commons Attribution (CC BY) license. Authors who
publish this series agree to the following terms:
1. Authors retain copyright and grant the series right of first publication with the work simultaneously licensed under a Creative Commons
Attribution License that allows others to share the work with an acknowledgment of the work's authorship and initial publication in this
series.
2. Authors are able to enter into separate, additional contractual arrangements for the non-exclusive distribution of the series's published
version of the work (e.g., post it to an institutional repository or publish it in a book), with an acknowledgment of its initial
publication in this series.
3. Authors are permitted and encouraged to post their work online (e.g., in institutional repositories or on their website) prior to and
during the submission process, as it can lead to productive exchanges, as well as earlier and greater citation of published work (See
Open access policy for details).