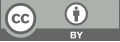
Data Visualization and Machine Learning Trend Analysis Forecasting Based on Roblox Stock Data
- 1 Shanghai Lixin University of Accounting and Finance
- 2 Shanghai Lixin University of Accounting and Finance
* Author to whom correspondence should be addressed.
Abstract
The stock market, as a highly complex, unstable and dynamically changing system, is affected by a wide range of factors, such as political, economic, social and technological, in terms of price changes. Therefore, stock price prediction is a challenging problem, but also a problem of great importance. In order to provide a more accurate and reliable method for stock closing price prediction, this paper aims to reduce investment risk and improve investment returns by comparing the prediction effects of different regression models for investors to make investment strategies and decisions. In this paper, Open, High, Low, and Adj Close are used as input parameters, Close is used as the target parameter, the final closing price of the stock is determined from the various stock data, the training set, validation set, and test set are divided in accordance with 6:2:2, and the predictive effect of the model is evaluated using the parameters of MSE, RMSE, MAE, MAPE, and R2. After the comparison of multiple machine learning algorithms, XGBoost regression model and Random Forest Regression model perform the best in the prediction of stock closing price, and their MSEs are 0.061 and 0.072 respectively; followed by LightGBM regression model and decision tree regression model, the MSEs of the two models are 0.113 and 0.299 respectively, but the prediction results of BP neural network regression are poor. By comparing the prediction results of different regression models, it can provide investors with a more accurate and reliable method to predict the closing price of stocks, help investors better formulate investment strategies and decisions, reduce investment risks and improve investment returns. This is of great significance to both individual and institutional investors.
Keywords
Roblox stock, Machine learning algorithms, MSE
[1]. Lin W ,Xie L ,Xu H .Deep-Reinforcement-Learning-Based Dynamic Ensemble Model for Stock Prediction[J].Electronics,2023,12(21):
[2]. Haodong H ,Liang X ,Shengshuang C , et al.Stock trend prediction based on industry relationships driven hypergraph attention networks[J].Applied Intelligence,2023,53(23): 29448-29464.
[3]. Jafar H S ,Akhtar S ,Chaarani E H , et al.Forecasting of NIFTY 50 Index Price by Using Backward Elimination with an LSTM Model[J].Journal of Risk and Financial Management,2023,16(10):
[4]. Fangzhao Z .RETRACTED: Extreme learning machine for stock price prediction[J].International Journal of Electrical Engineering Education,2023,60(1_suppl): 3972-3985.
[5]. Bhatta A ,Poudyal P ,Maharjan K D , et al.Assessing Machine Learning's Accuracy in Stock Price Prediction[J].International Journal of Computer (IJC),2023,49(1):46-63.
[6]. Haque S M ,Amin S M ,Miah J , et al.Boosting Stock Price Prediction with Anticipated Macro Policy Changes[J].Journal of Mathematics and Statistics Studies,2023,4(3):29-34.
[7]. Dongbo M ,Da Y ,Maojun H , et al.VGC-GAN: A multi-graph convolution adversarial network for stock price prediction[J].Expert Systems With Applications,2024,236.
[8]. Li C Y ,Huang Y H ,Yang P N , et al.Stock Market Forecasting Based on Spatiotemporal Deep Learning[J].Entropy,2023,25(9):
[9]. Sichong C .High-frequency stock return prediction using state-of-the-art deep learning models[J].International Journal of Financial Engineering,2023,10(03):
[10]. Barlybayev A ,Zhetkenbay L ,Karimov D , et al.Development neuro-fuzzy model to predict the stocks of companies in the electric vehicle industry[J].Eastern-European Journal of Enterprise Technologies,2023,4(4):72-87.
Cite this article
Wang,C.;Zhang,W. (2024). Data Visualization and Machine Learning Trend Analysis Forecasting Based on Roblox Stock Data. Advances in Economics, Management and Political Sciences,75,279-285.
Data availability
The datasets used and/or analyzed during the current study will be available from the authors upon reasonable request.
Disclaimer/Publisher's Note
The statements, opinions and data contained in all publications are solely those of the individual author(s) and contributor(s) and not of EWA Publishing and/or the editor(s). EWA Publishing and/or the editor(s) disclaim responsibility for any injury to people or property resulting from any ideas, methods, instructions or products referred to in the content.
About volume
Volume title: Proceedings of the 3rd International Conference on Business and Policy Studies
© 2024 by the author(s). Licensee EWA Publishing, Oxford, UK. This article is an open access article distributed under the terms and
conditions of the Creative Commons Attribution (CC BY) license. Authors who
publish this series agree to the following terms:
1. Authors retain copyright and grant the series right of first publication with the work simultaneously licensed under a Creative Commons
Attribution License that allows others to share the work with an acknowledgment of the work's authorship and initial publication in this
series.
2. Authors are able to enter into separate, additional contractual arrangements for the non-exclusive distribution of the series's published
version of the work (e.g., post it to an institutional repository or publish it in a book), with an acknowledgment of its initial
publication in this series.
3. Authors are permitted and encouraged to post their work online (e.g., in institutional repositories or on their website) prior to and
during the submission process, as it can lead to productive exchanges, as well as earlier and greater citation of published work (See
Open access policy for details).