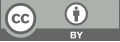
Influential Factors on California Regional Housing Price Analysed by Multiple Linear Regression
- 1 Victoria College, University of Toronto, Toronto, Ontario, Canada, M5S 1A1
* Author to whom correspondence should be addressed.
Abstract
While metropolises keep expanding with the increasing population in recent decades, the need for housing rises inevitably. This paper aims to find the most explanatory factors for the housing price in California; with 20433 entries of data found in Kaggle, the method of multiple linear regression (MLR) is applied to find the most influential factors. 500 entries in the dataset are chosen randomly and are divided into 2 datasets for training and testing purposes. Models have been developed in R by using the training dataset. After comparing the adjusted R square and variability of the models, the most convincible model will be selected to find out the result of this investigation on the test dataset. After model diagnostics, the result of this analysis is that the regional median income level has a strong positive correlation with the housing price, and it is the most influential factor. Other influential factors will be introduced in the conclusion.
Keywords
housing price, multiple linear regression, model construction
[1]. Pace, R. Kelley, and Ronald Barry. "Sparse spatial autoregressions." Statistics & Probability Letters 33.3 (1997): 291-297. https://www.kaggle.com/datasets/camnugent/california-housing-prices
[2]. S. Sisman, A.C. Aydinoglu “A modeling approach with geographically weighted regression methods for determining geographic variation and influencing factors in housing price: A case in Istanbul” https://doi.org/10.1016/j.landusepol.2022.106183
[3]. Sean Hollya, M. Hashem Pesarana, Takashi Yamagata “A spatio-temporal model of house prices in the USA” doi: 10.1016/j.jeconom.2010.03.040Yanchao Feng “Examining the determinants of housing prices and the influence of the spatial–temporal interaction effect: The case of China during 2003–2016” https://doi.org/10.1016/j.cjpre.2021.04.013
[4]. Yanchao Feng “Examining the determinants of housing prices and the influence of the spatial–temporal interaction effect: The case of China during 2003–2016” https://doi.org/10.1016/j.cjpre.2021.04.013
[5]. Noora Shrestha, Detecting Multicollinearity in Regression Analysis, American Journal of Applied Mathematics and Statistics. 2020, 8(2), 39-42. DOI: 10.12691/ajams-8-2-1
[6]. Simon J. Sheather. A Modern Approach to Regression with R. ISBN:978-0-387-09608-7. page 151.
Cite this article
Cao,Y. (2024). Influential Factors on California Regional Housing Price Analysed by Multiple Linear Regression. Advances in Economics, Management and Political Sciences,77,48-53.
Data availability
The datasets used and/or analyzed during the current study will be available from the authors upon reasonable request.
Disclaimer/Publisher's Note
The statements, opinions and data contained in all publications are solely those of the individual author(s) and contributor(s) and not of EWA Publishing and/or the editor(s). EWA Publishing and/or the editor(s) disclaim responsibility for any injury to people or property resulting from any ideas, methods, instructions or products referred to in the content.
About volume
Volume title: Proceedings of the 3rd International Conference on Business and Policy Studies
© 2024 by the author(s). Licensee EWA Publishing, Oxford, UK. This article is an open access article distributed under the terms and
conditions of the Creative Commons Attribution (CC BY) license. Authors who
publish this series agree to the following terms:
1. Authors retain copyright and grant the series right of first publication with the work simultaneously licensed under a Creative Commons
Attribution License that allows others to share the work with an acknowledgment of the work's authorship and initial publication in this
series.
2. Authors are able to enter into separate, additional contractual arrangements for the non-exclusive distribution of the series's published
version of the work (e.g., post it to an institutional repository or publish it in a book), with an acknowledgment of its initial
publication in this series.
3. Authors are permitted and encouraged to post their work online (e.g., in institutional repositories or on their website) prior to and
during the submission process, as it can lead to productive exchanges, as well as earlier and greater citation of published work (See
Open access policy for details).