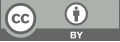
Analysis of Spam Classification Based on Naive Bayes and Random Forest Model
- 1 Department of Computer Science, Guangxi University of Science and Technology, Liuzhou, China
* Author to whom correspondence should be addressed.
Abstract
Spam classification has become more and more significant in email filtering and content auditing systems nowadays. Despite the development of many ways for filtering spam, spammers continue to adopt new methods for spam detection, which has left us overwhelmed with spam. Furthermore, robust, and flexible categorization algorithms are necessary to keep up with the constant evolution of spam tactics. The best method for categorizing and filtering spam now is to use machine learning techniques. In this study, a large spam dataset containing 5572 email instances is used in simulations for the spam classification task. This study comparatively analyzes two prevalent machine learning algorithms, namely, Random Forest and Naive Bayes. A detailed description of both algorithms, including their theoretical foundations and practical implementations in spam detection, is provided. In addition, the data was characterized in the study for training the models as well as making predictions. Finally, the effectiveness and performance of each algorithm is shown in the experimental evaluation using four commonly used performance evaluation metrics. Overall, these results providing insights into their strengths and limitations in practical spam filtering applications.
Keywords
Spam classification, Naive Bayes, Random Forest, Performance evaluation indicators, feature engineering
[1]. Pu, C. and Webb, S. (2006). Observed Trends in Spam Construction Techniques: A Case Study of Spam Evolution. In CEAS pp. 104-112)
[2]. Mishra, R. and Thakur, R.S. (2013). Analysis of random forest and Naive Bayes for spam mail using feature selection categorization. International Journal of Computer Applications, 80(3), 42-47.
[3]. Helfman, J. and Isbell, C. (1995). Ishmail: Immediate identification of important information. Technical report, AT&T Bell Laboratories, MIT Artificial Intelligence Laboratory.
[4]. Rennie, J. (2000). An application of machine learning to e-mail filtering. In Proc. KDD-2000 Text Mining Workshop pp. 75-80.
[5]. Yu, B. and Xu, Z.B. (2008). A comparative study for content-based dynamic spam classification using four machine learning algorithms. Knowledge-Based Systems, 21(4), 355-362.
[6]. Ramos, J. (2003). Using tf-idf to determine word relevance in document queries. Proceedings of the first instructional conference on machine learning, 242(1), 29-48.
[7]. Ramachandran, A., Dagon, D. and Feamster, N. (2006). Can DNS-based blacklists keep up with bots?. In CEAS.
[8]. Mishra, R. and Thakur, R.S. (2013). Analysis of random forest and Naive Bayes for spam mail using feature selection categorization. International Journal of Computer Applications, 80(3), 42-47.
[9]. Hall, M.A. and Holmes, G. (2003). Benchmarking attribute selection techniques for discrete class data mining. IEEE Transactions on Knowledge and Data engineering, 15(6), 1437-1447.
[10]. Schonlau, M. (2023). The Naive Bayes classifier. In Applied Statistical Learning: With Case Studies in Stata, Cham: Springer International Publishing, pp. 143-160.
Cite this article
Li,K. (2024). Analysis of Spam Classification Based on Naive Bayes and Random Forest Model. Advances in Economics, Management and Political Sciences,84,250-257.
Data availability
The datasets used and/or analyzed during the current study will be available from the authors upon reasonable request.
Disclaimer/Publisher's Note
The statements, opinions and data contained in all publications are solely those of the individual author(s) and contributor(s) and not of EWA Publishing and/or the editor(s). EWA Publishing and/or the editor(s) disclaim responsibility for any injury to people or property resulting from any ideas, methods, instructions or products referred to in the content.
About volume
Volume title: Proceedings of the 2nd International Conference on Management Research and Economic Development
© 2024 by the author(s). Licensee EWA Publishing, Oxford, UK. This article is an open access article distributed under the terms and
conditions of the Creative Commons Attribution (CC BY) license. Authors who
publish this series agree to the following terms:
1. Authors retain copyright and grant the series right of first publication with the work simultaneously licensed under a Creative Commons
Attribution License that allows others to share the work with an acknowledgment of the work's authorship and initial publication in this
series.
2. Authors are able to enter into separate, additional contractual arrangements for the non-exclusive distribution of the series's published
version of the work (e.g., post it to an institutional repository or publish it in a book), with an acknowledgment of its initial
publication in this series.
3. Authors are permitted and encouraged to post their work online (e.g., in institutional repositories or on their website) prior to and
during the submission process, as it can lead to productive exchanges, as well as earlier and greater citation of published work (See
Open access policy for details).