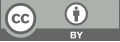
Implied Volatility Forecasting for American Options Based on Random Forest Regressor, Linear Regression Model
- 1 Department of Economics and Management, Changchun University of Science and Technology, Changchun, China
* Author to whom correspondence should be addressed.
Abstract
The research investigates the effectiveness of the Random Forest Model in accurately capturing the volatility of American options, a critical aspect of financial market analysis. Making use of a comprehensive dataset consisting of various parameters such as option prices, strike values, volume, and open interest, the research conducts thorough pre-processing tasks. This involves intricate procedures including feature engineering to extract meaningful predictors, handling of missing values, and ensuring uniform data standardization to facilitate model training. The study proceeds to train random forest models on the accurately processed dataset. Subsequently, the performance of these models is evaluated on a distinct test set to gauge their predictive capabilities accurately. The evaluation involves a comparative analysis between the Random Forest Model and a benchmark Linear Regression Model, employing widely accepted metrics like R^2 and MAPE. The findings underscore the outstanding performance of the Random Forest Model, showing enhanced accuracy and significantly reduced errors compared to the linear regression counterpart, which means Random Forest Model performs better. Furthermore, the study explores deeper into dissecting the strengths and weaknesses inherent in the Random Forest Model, shedding light on its potential applications and limitations in real-world financial scenes. By elucidating these aspects, the research provides valuable insights for practitioners in the field of financial trading and risk management. These findings serve as a significant contribution towards addressing the myriad challenges encountered in financial markets, empowering stakeholders with enhanced decision-making capabilities and more robust risk management strategies.
Keywords
Machine learning, Random Forest, option, implied volatility
[1]. Merton, R.C. (1973) Theory of rational option pricing. The Bell Journal of economics and management science, 141-183.
[2]. Cox, J. C., Ross, S. A. and Rubinstein, M. (1979) Option pricing: A simplified approach. Journal of financial Economics, 7(3), 229-263.
[3]. Ij, H. (2018) Statistics versus machine learning. Nat Methods, 15(4), 233.
[4]. Culkin, R. and Das, S.R. (2017). Machine learning in finance: the case of deep learning for option pricing. Journal of Investment Management, 15(4), 92-100.
[5]. Menger, K. and Menger, K. (2003) Statistical metrics. Selecta Mathematica: Volume 2, 433-435.
[6]. Capiński, M. and Kopp, E. (2012. The Black–Scholes Model. Cambridge University Press.
[7]. Janková, Z. (2018) Drawbacks and limitations of Black-Scholes model for options pricing. Journal of Financial Studies and Research, 2018, 1-7.
[8]. Teneng, D. (2011) Limitations of the Black-Scholes model. Collection of Papers, 1, 143.
[9]. Biau, G. and Scornet, E. (2016) A random forest guided tour. Test, 25, 197-227.
[10]. Liu, C., Chan, Y., Alam Kazmi, S.H. and Fu, H. (2015) Financial fraud detection model: Based on random forest. International journal of economics and finance, 7(7).
Cite this article
Liu,C. (2024). Implied Volatility Forecasting for American Options Based on Random Forest Regressor, Linear Regression Model. Advances in Economics, Management and Political Sciences,85,154-160.
Data availability
The datasets used and/or analyzed during the current study will be available from the authors upon reasonable request.
Disclaimer/Publisher's Note
The statements, opinions and data contained in all publications are solely those of the individual author(s) and contributor(s) and not of EWA Publishing and/or the editor(s). EWA Publishing and/or the editor(s) disclaim responsibility for any injury to people or property resulting from any ideas, methods, instructions or products referred to in the content.
About volume
Volume title: Proceedings of the 2nd International Conference on Management Research and Economic Development
© 2024 by the author(s). Licensee EWA Publishing, Oxford, UK. This article is an open access article distributed under the terms and
conditions of the Creative Commons Attribution (CC BY) license. Authors who
publish this series agree to the following terms:
1. Authors retain copyright and grant the series right of first publication with the work simultaneously licensed under a Creative Commons
Attribution License that allows others to share the work with an acknowledgment of the work's authorship and initial publication in this
series.
2. Authors are able to enter into separate, additional contractual arrangements for the non-exclusive distribution of the series's published
version of the work (e.g., post it to an institutional repository or publish it in a book), with an acknowledgment of its initial
publication in this series.
3. Authors are permitted and encouraged to post their work online (e.g., in institutional repositories or on their website) prior to and
during the submission process, as it can lead to productive exchanges, as well as earlier and greater citation of published work (See
Open access policy for details).