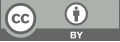
Predictive Analytics Techniques in Consumer Behaviour: A Literature Review
- 1 Financial Management, Donghua University, Shanghai, 200051, China
- 2 Applied statistics, Nanjing University of Posts and Telecommunications, Nanjing, 210023, China
- 3 Civil engineering management, Hongkong Chuhai college, Hongkong, China
- 4 Concord College, Shrewsbury, England
* Author to whom correspondence should be addressed.
Abstract
Under fierce market competition, there is a need for enterprises to analyze and predict the behavior of consumers in order to improve the market competitiveness. We find that in the previous literature, there is little specific systematic summary and research on the methods to predict customer behavior. Thus, in this paper, we discuss relevant concepts of customer behavior and predict consumer behavior by studying the operation mechanism of Big Data Analysis (BDA), Decision Tree (DT) and Consumer Relationship Management (CRM). We also study the application of each technology to enterprises in different fields and its impact on consumer behavior. BDA can efficiently organize a large amount of data into several variables that is related to the prediction and provide a reference prediction for the business. DT can effectively improve the accuracy of market segmentation, classify customers into sub-customer groups with distinct consumption characteristics, and help enterprises make targeted decisions. CRM can collect customer information through data sampling to build a comprehensive view of customers, and create customer analysis and prediction models according to the different needs of the enterprise. Studying the past consumer behavior can help enterprises to better develop products and make personalized decision plans for specific customer groups.
Keywords
Predictive analytics techniques, Consumer behavior, Big data, Decision tree, Consumer relationship management
[1]. Jisana, T. K. (2014). Consumer behaviour models: an overview. Sai Om Journal of Commerce & Management, 1(5), 34-43.
[2]. Peter, J. P., Olson, J. C., & Grunert, K. G. (1999). Consumer behavior and marketing strategy.
[3]. Su, X. (2017). Introduction to big data. IFUD1123.
[4]. Lee, C. S., Cheang, P. Y. S., & Moslehpour, M. (2022). Predictive analytics in business analytics: decision tree. Advances in Decision Sciences, 26(1), 1-29.
[5]. Tirenni, G., Kaiser, C., & Herrmann, A. (2007). Applying decision trees for value-based customer relations management: Predicting airline customers' future values. Journal of Database Marketing & Customer Strategy Management, 14, 130-142.
[6]. Duan, L., Bo, W., Wu, C., Ning, H., & Zhang, C. (2019). Customer Classification-Based Pre-sale Multi-value-chain Collaborative Mechanism Verification. In Human Centered Computing: 5th International Conference, HCC 2019, Čačak, Serbia, August 5–7, 2019, Revised Selected Papers 5 (pp. 86-97). Springer International Publishing.
[7]. Foxall, G. R. (1998). Radical Behaviorist Interpretation: Generating and evaluating an account of consumer behavior. Behavior Analyst, 21(2), 321-354.
[8]. Achar, C., So, J., Agrawal, N., & Duhachek, A. (2016). What we feel and why we buy: the influence of emotions on consumer decision-making. Current Opinion in Psychology, 10, 166-170.
[9]. Stankevich, A. (2017). Explaining the consumer decision-making process: Critical literature review. Journal of international business research and marketing, 2(6).
[10]. Lunenburg, F. C. (2010, September). The decision making process. In National Forum of Educational Administration & Supervision Journal (Vol. 27, No. 4).
[11]. ]McGill, A. L., & Anand, P. (1989). The effect of vivid attributes on the evaluation of alternatives: The role of differential attention and cognitive elaboration. Journal of consumer Research, 16(2), 188-196.
[12]. Bucko, J., Kakalejčík, L., & Ferencová, M. (2018). Online shopping: Factors that affect consumer purchasing behaviour. Cogent Business & Management, 5(1), 1535751.
[13]. Gajjar, N. B. (2013). Factors affecting consumer behavior. International Journal of Research in Humanities and Social Sciences, 1(2), 10-15.
[14]. Rehman, F. U., Ilyas, M., Nawaz, T., & Hyder, S. (2014). How Facebook advertising affects buying behavior of young consumers: The moderating role of gender. Academic Research International, 5(4), 395-404.
[15]. Pentz, C. D., Du Preez, R., & Swiegers, L. (2020). The online shopping behaviour of technologically enabled consumers: a South African Generation Y study. African Journal of Business and Economic Research, 15(3), 227.
[16]. Ratchford, B., Soysal, G., Zentner, A., & Gauri, D. K. (2022). Online and offline retailing: What we know and directions for future research. Journal of Retailing, 98(1), 152-177.
[17]. Brusdal, R., & Lavik, R. (2008). Just shopping? A closer look at youth and shopping in Norway. Young, 16(4), 393-408.
[18]. Cui, L., Yu, F. R., & Yan, Q. (2016). When big data meets software-defined networking: SDN for big data and big data for SDN. IEEE network, 30(1), 58-65.
[19]. Kumar, V., & Garg, M. L. (2018). Predictive analytics: a review of trends and techniques. International Journal of Computer Applications, 182(1), 31-37.
[20]. Gandomi, A., & Haider, M. (2015). Beyond the hype: Big data concepts, methods, and analytics.International journal of information management, 35(2), 137-144.
[21]. Sharma, H., & Kumar, S. (2016). A survey on decision tree algorithms of classification in data mining. International Journal of Science and Research (IJSR), 5(4), 2094-2097.
[22]. Kotsiantis, S. B. (2013). Decision trees: a recent overview. Artificial Intelligence Review, 39, 261-283.
[23]. Song, Y. Y., & Ying, L. U. (2015). Decision tree methods: applications for classification and prediction. Shanghai archives of psychiatry, 27(2), 130.
[24]. Suthaharan, S., & Suthaharan, S. (2016). Decision tree learning. Machine Learning Models and Algorithms for Big Data Classification: Thinking with Examples for Effective Learning, 237-269.
[25]. Tangirala, S. (2020). Evaluating the impact of GINI index and information gain on classification using decision tree classifier algorithm. International Journal of Advanced Computer Science and Applications, 11(2), 612-619.
[26]. Wellmann, J. F., & Regenauer-Lieb, K. (2012). Uncertainties have a meaning: Information entropy as a quality measure for 3-D geological models. Tectonophysics, 526, 207-216.
[27]. Sigala, M. (2004). Customer relationship management (CRM) evaluation: diffusing CRM benefits into business processes. ECIS 2004 Proceedings, 172.
[28]. Kracklauer, A. H., Mills, D. Q., & Seifert, D. (2004). Customer management as the origin of collaborative customer relationship management. In Collaborative customer relationship management: taking CRM to the next level (pp. 3-6). Berlin, Heidelberg: Springer Berlin Heidelberg.
[29]. Apte, C., Liu, B., Pednault, E. P., & Smyth, P. (2002). Business applications of data mining. Communications of the ACM, 45(8), 49-53.
[30]. Prasad, J., & Venkatesham, T. (2021). Big Data Analytics- in retail sector. International journal of computer science and mobile computing, 10(7), 34-38.
[31]. Vaca, C., RiofrÍo, D., Pérez, N., & BenÍtez, D. (2020, August). Buy & Sell Trends Analysis Using Decision Trees. In 2020 IEEE Colombian Conference on Applications of Computational Intelligence (IEEE ColCACI 2020) (pp. 1-6). IEEE.
[32]. Valecha, H., Varma, A., Khare, I., Sachdeva, A., & Goyal, M. (2018, November). Prediction of consumer behaviour using random forest algorithm. In 2018 5th IEEE Uttar Pradesh section international conference on electrical, electronics and computer engineering (UPCON) (pp. 1-6). IEEE.
[33]. Anderson, J. L., Jolly, L. D., & Fairhurst, A. E. (2007). Customer relationship management in retailing: A content analysis of retail trade journals. Journal of Retailing and Consumer services, 14(6), 394-399.
[34]. Minami, C., & Dawson, J. (2008). The CRM process in retail and service sector firms in Japan: Loyalty development and financial return. Journal of Retailing and Consumer Services, 15(5), 375-385.
[35]. Akter, S., & Wamba, S. F. (2016). Big Data Analytics in e-commerce: A Systematic Review and Agenda for Future research. Electronic Markets, 26(2), 173/185
[36]. Safara, F. (2022). A computational model to predict consumer behaviour during COVID-19 pandemic. Computational Economics, 59(4), 1525-1538.
[37]. Lee, S., Lee, S., & Park, Y. (2007). A prediction model for success of services in e-commerce using decision tree: E-customer’s attitude towards online service. Expert Systems with Applications, 33(3), 572-581.
[38]. Chan, C. C. H., Cheng, C. B., & Hsu, C. H. (2007). Bargaining strategy formulation with CRM for an e-commerce agent. Electronic Commerce Research and Applications, 6(4), 490-498.
[39]. Fan, Y. W., & Ku, E. (2010). Customer focus, service process fit and customer relationship management profitability: the effect of knowledge sharing. The Service Industries Journal, 30(2), 203-223.
[40]. Fang, B., & Zhang, P. (2016). Big data in finance. En Springer eBooks (pp. 391-412). 400-403
[41]. Wang, S. N., & Yang, J. G. (2007, August). A money laundering risk evaluation method based on decision tree. In 2007 international conference on machine learning and cybernetics (Vol. 1, pp. 283-286). IEEE.
[42]. Krasnikov, A., Jayachandran, S., & Kumar, V. (2009). The impact of customer relationship management implementation on cost and profit efficiencies: evidence from the US commercial banking industry. Journal of marketing, 73(6), 61-76.
[43]. Eid, R. (2007). Towards a successful CRM implementation in banks: An integrated model. The service industries journal, 27(8), 1021-1039.
Cite this article
Xu,Y.;Ma,Y.;Hu,R.;Wang,H. (2024). Predictive Analytics Techniques in Consumer Behaviour: A Literature Review. Advances in Economics, Management and Political Sciences,97,20-31.
Data availability
The datasets used and/or analyzed during the current study will be available from the authors upon reasonable request.
Disclaimer/Publisher's Note
The statements, opinions and data contained in all publications are solely those of the individual author(s) and contributor(s) and not of EWA Publishing and/or the editor(s). EWA Publishing and/or the editor(s) disclaim responsibility for any injury to people or property resulting from any ideas, methods, instructions or products referred to in the content.
About volume
Volume title: Proceedings of the 2nd International Conference on Financial Technology and Business Analysis
© 2024 by the author(s). Licensee EWA Publishing, Oxford, UK. This article is an open access article distributed under the terms and
conditions of the Creative Commons Attribution (CC BY) license. Authors who
publish this series agree to the following terms:
1. Authors retain copyright and grant the series right of first publication with the work simultaneously licensed under a Creative Commons
Attribution License that allows others to share the work with an acknowledgment of the work's authorship and initial publication in this
series.
2. Authors are able to enter into separate, additional contractual arrangements for the non-exclusive distribution of the series's published
version of the work (e.g., post it to an institutional repository or publish it in a book), with an acknowledgment of its initial
publication in this series.
3. Authors are permitted and encouraged to post their work online (e.g., in institutional repositories or on their website) prior to and
during the submission process, as it can lead to productive exchanges, as well as earlier and greater citation of published work (See
Open access policy for details).