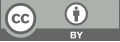
A Comparative Analysis of Machine Learning Algorithms for Predicting the Telemarketing Campaigns of Portuguese Banking Institutions
- 1 School of Computer Science, South China Normal University, Guangzhou, 510631, China
* Author to whom correspondence should be addressed.
Abstract
A financial institution is an entity of business that handles deposits, money circulation, saving, and other corresponding operations. Banks supply a broad spectrum of services. This differs depending on the capacity of each bank. The bank is going to provide further amenities, which is one of the many industries that frequently engage in direct product launches. Thus, banks might employ technological innovations to do market investigation before directly releasing products, which may assist with decision-making. Marketing initiatives that offer prospective consumers the option of embracing or rejecting an offered product can be carried out using direct email, phone calls, and email campaigns. The volume of incoming data continues to expand as time goes by. One of the bank institutions in Portugal faced difficulties judging whether or not its customer base would choose to sign up for a term deposit because of the growing amount of data. Consequently, to ascertain if the consumer will subscribe to a term deposit, the data mining processes utilized here will incorporate Ensemble Learning (Gradient Boosting (GB), Xg Boost (XGB), Random Forest (RF), Linear Models (Logistic Forest (RF), Linear Models (Logistic Regression (LR), Non-Linear Models (K-Near Neighbors (KNN), Support Vector Machine (SVM), Decision Tree Classifier (DT)), and Probabilistic Models (Naive Bayes (NB))included in classification techniques. Among these, Xg Boost yields the most impressive results, with the best performance in terms of AUC, model accuracy, and cross-validation accuracy in a minimum amount of computation time.
Keywords
Direct Marketing, Telemarketing Campaigns, Machine Learning
[1]. Hagen, L., Uetake, K., Yang, N., Bollinger, B., Chaney, A. J. B., Dzyabura, D., Etkin, J., Goldfarb, A., Liu, L., Sudhir, K., Wang, Y., Wright, J. R., & Zhu, Y. (2020). How can machine learning aid behavioral marketing research? Marketing Letters, 31(4), 361–370.
[2]. Mitić, V. (2019). Benefits of artificial intelligence and machine learning in marketing. In Sinteza 2019 - International Scientific Conference on Information Technology and Data Related Research (pp. 472-477).
[3]. Borugadda, P., Nandru, P., & Madhavaiah, C. (2021). Predicting the success of bank telemarketing for selling long-term deposits: An application of machine learning algorithms. St. Theresa Journal of Humanities and Social Sciences, 7(1), 91-108.
[4]. Umam, F. S. A., & R. A. (2021). Determinants of Mudharabah term deposit: A case of Indonesia Islamic banks. Journal of Economics Research and Social Sciences, 5(2), 167-180.
[5]. Mari, A. (2019). The rise of machine learning in marketing: Goal, process, and benefit of AI-driven marketing. Swiss Cognitive.
[6]. Gupta, A., & Gupta, G. (2018). Comparative study of random forest and neural network for prediction in direct marketing. In Advances in Intelligent Systems and Computing (Vol. 697, pp. 401-410). Springer.
[7]. Zaki, A. M., Khodadadi, N., Lim, W. H., & Towfek, S. K. (2024). Predictive analytics and machine learning in direct marketing for anticipating bank term deposit subscriptions. American Journal of Business and Operations Research, 11(1), 79-88.
[8]. Li, Z., Xu, Z., & Zhou, Y. (2024). Application of machine learning on client prediction in bank marketing. In Economic Management and Big Data Application: Proceedings of the 3rd International Conference (pp. 1064-1076).
[9]. Tang, X., & Zhu, Y. (2024). Enhancing bank marketing strategies with ensemble learning: Empirical analysis. PLOS ONE, 19(1), e0294759.
[10]. Koripi, M. (2021). A review on secure communications and wireless personal area networks (WPAN). Wutan Huatan Jisuan Jishu, XVII(VII), 168-174.
Cite this article
Huang,S. (2024). A Comparative Analysis of Machine Learning Algorithms for Predicting the Telemarketing Campaigns of Portuguese Banking Institutions. Advances in Economics, Management and Political Sciences,94,44-53.
Data availability
The datasets used and/or analyzed during the current study will be available from the authors upon reasonable request.
Disclaimer/Publisher's Note
The statements, opinions and data contained in all publications are solely those of the individual author(s) and contributor(s) and not of EWA Publishing and/or the editor(s). EWA Publishing and/or the editor(s) disclaim responsibility for any injury to people or property resulting from any ideas, methods, instructions or products referred to in the content.
About volume
Volume title: Proceedings of ICFTBA 2024 Workshop: Finance in the Age of Environmental Risks and Sustainability
© 2024 by the author(s). Licensee EWA Publishing, Oxford, UK. This article is an open access article distributed under the terms and
conditions of the Creative Commons Attribution (CC BY) license. Authors who
publish this series agree to the following terms:
1. Authors retain copyright and grant the series right of first publication with the work simultaneously licensed under a Creative Commons
Attribution License that allows others to share the work with an acknowledgment of the work's authorship and initial publication in this
series.
2. Authors are able to enter into separate, additional contractual arrangements for the non-exclusive distribution of the series's published
version of the work (e.g., post it to an institutional repository or publish it in a book), with an acknowledgment of its initial
publication in this series.
3. Authors are permitted and encouraged to post their work online (e.g., in institutional repositories or on their website) prior to and
during the submission process, as it can lead to productive exchanges, as well as earlier and greater citation of published work (See
Open access policy for details).