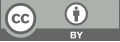
Review on the Use of Data Analysis for Customer Segmentation and Personalization in Marketing Strategies
- 1 Wuhan Britain-China School
- 2 Zhenhai High School
- 3 Jiangsu Taizhou High School
* Author to whom correspondence should be addressed.
Abstract
This paper studies the application of data analysis in the field of customer segmentation and personalization. With the advent of the era of big data, enterprises and organizations have rich data resources that contain important information about customer behavior, preferences, and purchasing habits. Through data analytics, companies can better understand customer needs and provide personalized products and services, thereby improving customer satisfaction and loyalty. This study aims to explore the application of data analytics in the field of customer segmentation and personalization, and provides some common data analysis methods. Data analysis methods include probabilistic methods and Bayesian methods, among others. Through case studies, the application of data analysis in the field of e-commerce is explained. The research results show that data analytics has important value and potential applications in customer segmentation and personalized marketing. However, data analysis still faces some challenges and limitations in practice, including data security and quality issues. Future research can continue to explore methods and techniques for data analysis, solve problems such as data privacy and security, and apply data analysis in more fields.
Keywords
data analysis, customer segmentation, personalized marketing, e-commerce.
[1]. Mahdavinejad, M. S., Rezvan, M., Barekatain, M., Adibi, P., Barnaghi, P., & Sheth, A. P. (2018). Machine learning for internet of things data analysis: A survey. Digital Communications and Networks, 4(3), 161–175. https://doi.org/10.1016/j.dcan.2017.10.002
[2]. Johnston, M. P. (2014). Secondary Data Analysis: A Method of which the Time Has Come. Qualitative and Quantitative Methods in Libraries.
[3]. Wilk, M. B., & Gnanadesikan, R. (1968). Probability Plotting Methods for the Analysis of Data. Biometrika, 55(1), 1. https://doi.org/10.2307/2334448
[4]. Cooil, B., Aksoy, L., & Keiningham, T. L. (2008). Approaches to Customer Segmentation. Journal of Relationship Marketing, 6(3–4), 9–39. https://doi.org/10.1300/J366v06n03_02
[5]. Anshari, M., Almunawar, M. N., Lim, S. A., & Al-Mudimigh, A. (2019). Customer relationship management and big data enabled: Personalization & customization of services. Applied Computing and Informatics, 15(2), 94–101. https://doi.org/10.1016/j.aci.2018.05.004
[6]. Kim, S.-Y., Jung, T.-S., Suh, E.-H., & Hwang, H.-S. (2006). Customer segmentation and strategy development based on customer lifetime value: A case study. Expert Systems with Applications, 31(1), 101–107. https://doi.org/10.1016/j.eswa.2005.09.004
[7]. Fan, H., & Poole, M. S. (2006). What Is Personalization? Perspectives on the Design and Implementation of Personalization in Information Systems. Journal of Organizational Computing and Electronic Commerce, 16(3–4), 179–202. https://doi.org/10.1080/10919392.2006.9681199
[8]. Sundar, S. S., & Marathe, S. S. (2010). Personalization versus Customization: The Importance of Agency, Privacy, and Power Usage. Human Communication Research, 36(3), 298–322. https://doi.org/10.1111/j.1468-2958.2010.01377.x
[9]. Essalmi, F., Ayed, L. J. B., Jemni, M., Kinshuk, & Graf, S. (2010). A fully personalization strategy of E-learning scenarios. Computers in Human Behavior, 26(4), 581–591. https://doi.org/10.1016/j.chb.2009.12.010
[10]. Kruschke, J. K. (2010). What to believe: Bayesian methods for data analysis. Trends in Cognitive Sciences, 14(7), 293–300. https://doi.org/10.1016/j.tics.2010.05.001
[11]. Kruschke, J. K., Aguinis, H., & Joo, H. (2012). The Time Has Come: Bayesian Methods for Data Analysis in the Organizational Sciences. Organizational Research Methods, 15(4), 722–752. https://doi.org/10.1177/1094428112457829
[12]. Alrumiah, S. S., & Hadwan, M. (2021). Implementing Big Data Analytics in E-Commerce: Vendor and Customer View. IEEE Access, 9, 37281–37286. https://doi.org/10.1109/ACCESS.2021.3063615
[13]. Poprawe, M. (2015). A panel data analysis of the effect of corruption on tourism. Applied Economics, 47(23), 2399–2412. https://doi.org/10.1080/00036846.2015.1005874
[14]. Chasapopoulos, P., Butter, F. A. G. D., & Mihaylov, E. (2014). Demand for tourism in Greece: A panel data analysis using the gravity model. International Journal of Tourism Policy, 5(3), 173. https://doi.org/10.1504/IJTP.2014.063105
Cite this article
Xiao,M.;Xu,Y.;Gao,Z. (2024). Review on the Use of Data Analysis for Customer Segmentation and Personalization in Marketing Strategies. Advances in Economics, Management and Political Sciences,106,10-16.
Data availability
The datasets used and/or analyzed during the current study will be available from the authors upon reasonable request.
Disclaimer/Publisher's Note
The statements, opinions and data contained in all publications are solely those of the individual author(s) and contributor(s) and not of EWA Publishing and/or the editor(s). EWA Publishing and/or the editor(s) disclaim responsibility for any injury to people or property resulting from any ideas, methods, instructions or products referred to in the content.
About volume
Volume title: Proceedings of the 3rd International Conference on Business and Policy Studies
© 2024 by the author(s). Licensee EWA Publishing, Oxford, UK. This article is an open access article distributed under the terms and
conditions of the Creative Commons Attribution (CC BY) license. Authors who
publish this series agree to the following terms:
1. Authors retain copyright and grant the series right of first publication with the work simultaneously licensed under a Creative Commons
Attribution License that allows others to share the work with an acknowledgment of the work's authorship and initial publication in this
series.
2. Authors are able to enter into separate, additional contractual arrangements for the non-exclusive distribution of the series's published
version of the work (e.g., post it to an institutional repository or publish it in a book), with an acknowledgment of its initial
publication in this series.
3. Authors are permitted and encouraged to post their work online (e.g., in institutional repositories or on their website) prior to and
during the submission process, as it can lead to productive exchanges, as well as earlier and greater citation of published work (See
Open access policy for details).