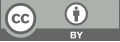
Metal Futures Prices Prediction Based on LSTM-GRU Hybrid Model
- 1 Beijing University of Posts and Telecommunications
* Author to whom correspondence should be addressed.
Abstract
Accurately predicting the prices of metal futures such as aluminum, copper, lead, nickel, tin, and zinc is crucial for investors, manufacturers, and policymakers due to their significant impact on economic activities and industrial processes. This paper presents a comprehensive study on the prediction of futures prices using a hybrid model combining Long Short-Term Memory (LSTM) and Gated Recurrent Unit (GRU) neural networks. The research utilizes historical data from 2014 to 2024, including daily open, high, low, close prices, trading volumes, and return. Data preprocessing involved interpolation for missing values and normalization using MinMaxScaler. The model’s performance was evaluated with various accuracy metrics, such as Root Mean Squared Error (RMSE), Mean Squared Error (MSE), Mean Absolute Error (MAE) ect, showing high accuracy for lead, tin, and zinc futures in both train and test datasets. However, the model performed well only on the train data for aluminum, copper, and nickel, indicating the need for more iterations, additional factors, or the consideration of unexpected events. The results highlight the efficacy of the LSTM-GRU hybrid model in futures price prediction, with significant implications for informed investment decisions. Future research should consider cross-market analysis and additional factors to enhance model accuracy further.
Keywords
Metal Futures, LSTM-GRU Hybrid Model, Price Prediction, Market Analysis
[1]. Ghosh, S., Examining crude oil price - Exchange rate nexus for India during the period of extreme oil price volatility. Applied Energy, 166, 2016, 113-120.
[2]. Shi, P., Song, S., Lang, Q., et al., Futures Price Forecasting Based on LSTM Model Using Public Opinion Sentiment Analysis. In: Technical Committee on Control Theory, Chinese Association of Automation (Eds.), Proceedings of the 42nd Chinese Control Conference (15), 2023, 7,7-12.
[3]. Lei, Z., Chen, Y., Bouri, E., Time-varying jump intensity and volatility forecasting of crude oil returns. Energy Economics, 70, 2018, 40-49.
[4]. Longyue, L., Ying, H., Research on short-term forecasting methods of gold futures prices—Analysis of COMEX gold futures price data based on CEEMDAN and LSTM models. Price Theory and Practice, 2023, 09, 164-168.
[5]. Gong, H., Xing, H., Yu, Y., Liang, Y. A combined model using secondary decomposition for crude oil futures price and volatility forecasting: Analysis based on comparison and ablation experiments. Expert Systems With Applications, 120(2019), 370-379.
[6]. Researchers from University of Oviedo Detail New Studies and Findings in the Area of Support Vector Machines. PM10 concentration forecasting in the metropolitan area of Oviedo (Northern Spain) using models based on SVM, MLP, VARMA and ARIMA: A … Ecology Environment & Conservation, 24(2), 2018, 312-320.
[7]. Wei, W. Research on Multivariate Futures Price Forecasting Based on IMAT-LSTM Model. Academic Journal of Computing & Information Science, 6(1), 2023, 15-26.
[8]. Ceperic, E., et al. Machine Learning; Findings from E. Ceperic et al Has Provided New Information about Machine Learning (Short-term forecasting of natural gas prices using machine learning and feature selection algorithms). Energy Weekly News, 2019, 45-47.
[9]. Lianlian, F., Xinsheng, D., Yuehui, D. Ensemble empirical mode decomposition-based preprocessing method with Multi-LSTM for time series forecasting: a case study for hog prices. Connection Science, 31(4), 2019, 379-393.
[10]. Sun, J.Y., Bing, G.Y. A new method for predicting Shanghai copper futures prices integrating multi-source data information. Operations Research and Management Science, 1-8 [2024-07-08]. Available at: http://kns.cnki.net/kcms/detail/34.1133.G3.20240628.1434.004.html.
[11]. Mostafa, M., Dipu, K.H.M., Farnad, N., Abbas, K. Neural network-based interval forecasting of construction material prices. Journal of Building Engineering, 30, 2020, 101-110.
Cite this article
Liu,B. (2024). Metal Futures Prices Prediction Based on LSTM-GRU Hybrid Model. Advances in Economics, Management and Political Sciences,94,164-174.
Data availability
The datasets used and/or analyzed during the current study will be available from the authors upon reasonable request.
Disclaimer/Publisher's Note
The statements, opinions and data contained in all publications are solely those of the individual author(s) and contributor(s) and not of EWA Publishing and/or the editor(s). EWA Publishing and/or the editor(s) disclaim responsibility for any injury to people or property resulting from any ideas, methods, instructions or products referred to in the content.
About volume
Volume title: Proceedings of ICFTBA 2024 Workshop: Finance in the Age of Environmental Risks and Sustainability
© 2024 by the author(s). Licensee EWA Publishing, Oxford, UK. This article is an open access article distributed under the terms and
conditions of the Creative Commons Attribution (CC BY) license. Authors who
publish this series agree to the following terms:
1. Authors retain copyright and grant the series right of first publication with the work simultaneously licensed under a Creative Commons
Attribution License that allows others to share the work with an acknowledgment of the work's authorship and initial publication in this
series.
2. Authors are able to enter into separate, additional contractual arrangements for the non-exclusive distribution of the series's published
version of the work (e.g., post it to an institutional repository or publish it in a book), with an acknowledgment of its initial
publication in this series.
3. Authors are permitted and encouraged to post their work online (e.g., in institutional repositories or on their website) prior to and
during the submission process, as it can lead to productive exchanges, as well as earlier and greater citation of published work (See
Open access policy for details).