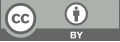
Revolutionizing Inventory Management: The Role of Data Mining in Industry 4.0
- 1 James Cook University, Singapore, Singapore, 387380
- 2 School of Mathematics and Artificial Intelligence, Chongqing University of Arts and Sciences, Chongqing, China, 402160
* Author to whom correspondence should be addressed.
Abstract
In the era of Industry 4.0, with the rapid development and application of technologies such as the Internet of Things (IoT), Big Data and Artificial Intelligence (AI), the manufacturing industry is undergoing unprecedented changes. In this context, data mining technology has become integral to inventory management practices in all industries. This study examines the profound impact of data mining on inventory management efficiency. By leveraging advanced analytics and machine learning algorithms, data mining enables organizations to accurately forecast demand, optimize inventory levels, and improve supply chain transparency. Through real-world case studies and comprehensive analysis, this research highlights how data mining techniques, such as central object-based clustering algorithms, can be successfully applied to optimize material classification, warehouse space utilization and operational efficiency. In addition, this study explores the broader applications of data mining beyond inventory management, including marketing, financial risk management, healthcare, transportation, social media, and cybersecurity. Overall, this study provides valuable insights into how data mining can reshape inventory management practices and drive business growth in the digital age.
Keywords
Industry 4.0, Data mining, Algorithms, Inventory management
[1]. Speshilov, E., & Nesedov, P. (2023). Algorithmization of data mining to optimize the inventory management process at the enterprise in conditions of uncertainty. Otkhody i Resursy, 10(1).
[2]. Granillo-Macías, R. (2020). Inventory Management and Logistics Optimization: A Data Mining Practical Approach. Logforum, 16(4), 535-547.
[3]. Rogić, S., & Kašćelan, L. (2021). Segmentation Approach for Athleisure and Performance Sport Retailers Based on Data Mining Techniques. International Journal of E-Services and Mobile Applications, 13(3), 71-85.
[4]. Jodha, R. (2023). Applications of Data Mining - Scaler Topics. https://www.scaler.com/topics/data-mining-tutorial/application-of-data-mining/
[5]. Kara, M. E., Fırat, S. Ü. O., & Ghadge, A. (2020). A data mining-based framework for supply chain risk management. Computers & Industrial Engineering, 139, 105570.
[6]. Yang, J., & Ying, L. (2021). Application of Data Mining in the Evaluation of Enterprise Lean Management Effect.
[7]. Tkachenko, A., & Chernyshov, M. (2024). Using the Power Query System for Processing and Data Mining SAP ERP. E3S Web of Conferences, 474, 02029.
[8]. Granillo-Macías, R. (2020). Inventory management and logistics optimization: a data mining practical approach. LogForum, 16(4).
[9]. Abby, J. (2021). Inventory Analytics: Best Practices for Smart, Data-Driven Decisions. Netsuite. https://www.netsuite.com/portal/resource/articles/inventory-management/inventory-analytics.shtml
[10]. Conrad, R. (2024). The Future of Warehousing: How AI Is Transforming Inventory Management and Order Fulfillment. https://rtslabs.com/ai-warehouse-automation-picking-optimization
[11]. Zhang, Q., Zheng, L., & Zhang, C. (2006). Application of rough set data mining technology in marketing. Bingong Zidonghua, 25(6), 37-38.
[12]. Hu, L. (2024). Research on risk management of Internet finance under the background of big data. China Economy and Trade, 2024(1), 229-231.
[13]. He, Y. (2024). Research on medical data analysis and mining based on big data. Weixing Jisuanji, 2024(2), 46-48.
[14]. Yang, J. (2024). Application of artificial intelligence technology in road traffic management. Shuzi Tongxin Shijie, 2024(3), 105-107.
[15]. Chi, Z. (2024). Big data network security solutions based on artificial intelligence. Jisuanji Yingyong Wenzhai, 40(2), 93-95.
Cite this article
Huo,Z.;Zhan,X. (2024). Revolutionizing Inventory Management: The Role of Data Mining in Industry 4.0. Advances in Economics, Management and Political Sciences,109,67-72.
Data availability
The datasets used and/or analyzed during the current study will be available from the authors upon reasonable request.
Disclaimer/Publisher's Note
The statements, opinions and data contained in all publications are solely those of the individual author(s) and contributor(s) and not of EWA Publishing and/or the editor(s). EWA Publishing and/or the editor(s) disclaim responsibility for any injury to people or property resulting from any ideas, methods, instructions or products referred to in the content.
About volume
Volume title: Proceedings of ICEMGD 2024 Workshop: Innovative Strategies in Microeconomic Business Management
© 2024 by the author(s). Licensee EWA Publishing, Oxford, UK. This article is an open access article distributed under the terms and
conditions of the Creative Commons Attribution (CC BY) license. Authors who
publish this series agree to the following terms:
1. Authors retain copyright and grant the series right of first publication with the work simultaneously licensed under a Creative Commons
Attribution License that allows others to share the work with an acknowledgment of the work's authorship and initial publication in this
series.
2. Authors are able to enter into separate, additional contractual arrangements for the non-exclusive distribution of the series's published
version of the work (e.g., post it to an institutional repository or publish it in a book), with an acknowledgment of its initial
publication in this series.
3. Authors are permitted and encouraged to post their work online (e.g., in institutional repositories or on their website) prior to and
during the submission process, as it can lead to productive exchanges, as well as earlier and greater citation of published work (See
Open access policy for details).