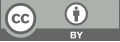
How the US Macroeconomic Factors Affect the Gold Price?
- 1 Boston University, Boston, United States
- 2 Rutgers University New Brunswick, New Brunswick NJ, United States
* Author to whom correspondence should be addressed.
Abstract
Nowadays, investing in gold as a hedge against macroeconomic factors risk has been brought into the spotlight. It is essential for investors to predict gold prices before making their hedging decisions. After a thorough study of past precursors, the machine learning method, especially the gradient boosting technique, has been proven to be a powerful method for predicting gold prices. This study tests macroeconomic factors as well as oil prices and uses them as independent variables to predict gold prices. By using advanced machine learning technique: gradient boosting, this study achieves finding the best four factors model. The research shows that oil prices, the U.S. dollar index, interest rate, and GDP growth are significant factors in predicting gold prices. Among all the significant factors, oil price is the most important one. The explicit reasons of this result is because that transportation cost and operation cost of gold mining is largely influenced by oil price. This study lays the foundations for investors to make insightful decisions.
Keywords
U.S. market, macroeconomic factors, gold price, gradient boosting model
[1]. A, L., Qin, Q., Kamran, H. W., Sawangchai, A., Wisetsri, W., & Raza, M. (2021). How macroeconomic indicators influence gold price management. Business Process Management Journal. https://www.emerald.com/insight/content/doi/10.1108/BPMJ-12-2020-0579/full/html
[2]. Yaqub, K. Q. (2022). The impact of macroeconomic factors on the price of gold. https://digitalcommons.buffalostate.edu/cgi/viewcontent.cgi?article=1036&context=economics_theses
[3]. Folger, J. (2023). What drives the price of gold?. Investopedia. https://www.investopedia.com/financial-edge/0311/what-drives-the-price-of-gold.aspx
[4]. Khan, F., Urooj, A., & Muhammadullah, S. (2021, January). An Arima-Ann Hybrid Model for Time Series forecasting https://www.researchgate.net/publication/263454208_An_ARIMA-ANN_hybrid_model_for_time_series_forecasting
[5]. Lucy, G. (2024). Predicting gold prices with alternative data https://www.neudata.co/intelligence/predicting-gold-prices-with-alternative-data
[6]. Varshini A, Kayal P, Maiti M. (2024). How good are different machine and deep learning models in forecasting the future price of metals? Full sample versus sub-sample, Resources Policy, 92.
[7]. Manjuala, R., & Karthikeyan, S. (2023). Gold Price Prediction using Ensemble based Machine Manjula K A Learning Techniques, https://ieeexplore.ieee.org/document/8862557
[8]. Naser, M.Z., Alavi, A.H. (2023). Error Metrics and Performance Fitness Indicators for Artificial Intelligence and Machine Learning in Engineering and Sciences. Archit. Struct. Constr. 3, 499–517.
Cite this article
Xiong,H.;Zhang,W. (2024). How the US Macroeconomic Factors Affect the Gold Price?. Advances in Economics, Management and Political Sciences,124,32-37.
Data availability
The datasets used and/or analyzed during the current study will be available from the authors upon reasonable request.
Disclaimer/Publisher's Note
The statements, opinions and data contained in all publications are solely those of the individual author(s) and contributor(s) and not of EWA Publishing and/or the editor(s). EWA Publishing and/or the editor(s) disclaim responsibility for any injury to people or property resulting from any ideas, methods, instructions or products referred to in the content.
About volume
Volume title: Proceedings of the 8th International Conference on Economic Management and Green Development
© 2024 by the author(s). Licensee EWA Publishing, Oxford, UK. This article is an open access article distributed under the terms and
conditions of the Creative Commons Attribution (CC BY) license. Authors who
publish this series agree to the following terms:
1. Authors retain copyright and grant the series right of first publication with the work simultaneously licensed under a Creative Commons
Attribution License that allows others to share the work with an acknowledgment of the work's authorship and initial publication in this
series.
2. Authors are able to enter into separate, additional contractual arrangements for the non-exclusive distribution of the series's published
version of the work (e.g., post it to an institutional repository or publish it in a book), with an acknowledgment of its initial
publication in this series.
3. Authors are permitted and encouraged to post their work online (e.g., in institutional repositories or on their website) prior to and
during the submission process, as it can lead to productive exchanges, as well as earlier and greater citation of published work (See
Open access policy for details).