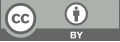
MOUTAI Stock Price Prediction Based on ARIMA Model
- 1 Beijing National Day School
* Author to whom correspondence should be addressed.
Abstract
Autoregressive Integrated Moving Average (ARIMA) models are effectively utilized in time series analysis and forecasts. With the focus on MOUTAI, one of the brands in liquor industry that has absorbed great public interest and wide acceptance, this paper studies the pattern of the closing price of MOUTAI stock, fits the data using ARIMA model, and eventually forecasts over the future horizon. Based on daily closing price of the specific stock, a few transformations of the data are carried out, making the fitting process of ARIMA model more accurate. The best-fit ARIMA model is studied and the forecast re-sults are analyzed. According to the increasing trend throughout the interval studied and the unforeseen peak at the beginning of 2021, the causation is discussed: the consumer trend. That is, people are inclined to consume more liquor products during mood swings, which is likely to happen during lock downs and the deteriorated epidemic worldwide. This study provides more insights to the potential investors and buyers of MOUTAI stock and products, and furthermore the liquor industry, giving an overview of the patterns shown in the stock’s closing price and the causation of the forecast results.
Keywords
MOUTAI, ARIMA, prediction
[1]. Calina, Daniela, et al. "COVID-19 pandemic and alcohol consumption: Impacts and interconnections." Toxicology reports 8: 529-535. (2021)
[2]. Colbert, Stephanie, et al. "COVID‐19 and alcohol in Australia: industry changes and public health impacts." Drug and alcohol review (2020).
[3]. Stockwell, Tim, et al. "Alcohol sales in Canadian liquor outlets as a predictor of subsequent COVID-19 infection rates: a time-series analysis." Addiction (2022).
[4]. Ramalho, Rodrigo. "Alcohol consumption and alcohol-related problems during the COVID-19 pandemic: a narrative review." Australasian Psychiatry 28.5: 524-526. (2020)
[5]. Xu, Y., and K. Ji. "Moutai (Maotai): production and sensory properties." Alcoholic Beverages: 315-330. (2012)
[6]. P. Chen, H. Yuan and X. Shu, "Forecasting Crime Using the ARIMA Model," 2008 Fifth International Conference on Fuzzy Systems and Knowledge Discovery, 627-630, (2008).
[7]. Fattah, Jamal, et al. "Forecasting of demand using ARIMA model." International Journal of Engineering Business Management 10: 1847979018808673 (2018).
[8]. Nochai, Rangsan, and Titida Nochai. "ARIMA model for forecasting oil palm price." Proceedings of the 2nd IMT-GT Regional Conference on Mathematics, Statistics and applications (2006).
[9]. Hyndman, Rob J., and George Athanasopoulos. "Autoregressive models." de Forecasting: Principles and Practice, otexts (2018).
[10]. Eom, K. B. . "Analysis of Acoustic Signatures from Moving Vehicles Using Time-Varying Autoregressive Models." Multidimensional Systems and Signal Processing 10(4), 357-378(1999).
Cite this article
Duan,S. (2023). MOUTAI Stock Price Prediction Based on ARIMA Model. Advances in Economics, Management and Political Sciences,14,34-41.
Data availability
The datasets used and/or analyzed during the current study will be available from the authors upon reasonable request.
Disclaimer/Publisher's Note
The statements, opinions and data contained in all publications are solely those of the individual author(s) and contributor(s) and not of EWA Publishing and/or the editor(s). EWA Publishing and/or the editor(s) disclaim responsibility for any injury to people or property resulting from any ideas, methods, instructions or products referred to in the content.
About volume
Volume title: Proceedings of the 2nd International Conference on Business and Policy Studies
© 2024 by the author(s). Licensee EWA Publishing, Oxford, UK. This article is an open access article distributed under the terms and
conditions of the Creative Commons Attribution (CC BY) license. Authors who
publish this series agree to the following terms:
1. Authors retain copyright and grant the series right of first publication with the work simultaneously licensed under a Creative Commons
Attribution License that allows others to share the work with an acknowledgment of the work's authorship and initial publication in this
series.
2. Authors are able to enter into separate, additional contractual arrangements for the non-exclusive distribution of the series's published
version of the work (e.g., post it to an institutional repository or publish it in a book), with an acknowledgment of its initial
publication in this series.
3. Authors are permitted and encouraged to post their work online (e.g., in institutional repositories or on their website) prior to and
during the submission process, as it can lead to productive exchanges, as well as earlier and greater citation of published work (See
Open access policy for details).