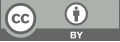
Google’s Stock Price in Covid-19: An Exploration of Machine Learning Techniques for Prediction
- 1 University College London
* Author to whom correspondence should be addressed.
Abstract
The stock market, as well as the global financial and public health systems, were significantly impacted by the abrupt onset of the COVID-19 pandemic. The complicated dynamics caused by the epidemic made it even more difficult to predict stock values. Forecasts from conventional models were less accurate because they have trouble reflecting the psychological characteristics of investors. To increase the accuracy of stock price predictions, researchers investigated machine learning methods like hybrid models and Artificial Neural Networks. In terms of forecasting stock values during crises, there is still a study void. This study study investigates the applicability of decision trees, random forests, and Long Short Term Memory (LSTM) models for analyzing stock market dynamics in the context of an epidemic. Through comparative analysis, it was determined that the LSTM model outperformed the alternative methods, thus establishing its superiority in predictive accuracy. The implications of these findings extend to investors and regulatory bodies, shedding light on the behavior of stock markets during periods of adversity. Subsequent research endeavors should focus on exploring innovative techniques that can further enhance the precision of stock market predictions.
Keywords
pandemic, stock price prediction, machine learning, Long Short-Term Memory
[1]. Mazur, M., Dang, M., & Vega, M. (2021). Covid-19 and the March 2020 stock market crash: Evidence from S&P1500. Finance Research Letters, 38, 101690.
[2]. Ramelli, S., & Wagner, A. (2020). What the stock market tells us about the consequences of covid-19: Mitigating the COVID Economic Crisis: Act Fast and Do Whatever, 63.
[3]. Zhang, D., Hu, M., & Ji, Q. (2020). Financial markets under the global pandemic of covid-19. Finance Research Letters, 36, 101528.
[4]. Khattak, M. A., Ali, M., & Rizvi, S. A. R. (2021). Predicting the European stock market during covid-19: A machine learning approach. MethodsX, 8, 101198.
[5]. Mair, C., Kadoda, G., Lefley, M., Phalp, K., Schofield, C., Shepperd, M., & Webster, S. (2000). An investigation of machine learning based prediction systems. Journal of Systems and Software, 53(1), 23-29.
[6]. Ribeiro, G. T., Santos, A. A. P., Mariani, V. C., & Coelho, L. D. S. (2021). Novel hybrid model based on echo state neural network applied to the prediction of stock price return volatility. Expert Systems with Applications, ISSN 0957-4174.
[7]. Elgammal, M., Salama, K., & Mohieldin, A. (2019). A Comparison of Artificial Neural Network (ANN) and Support Vector Machine (SVM) Classifiers for Neural Seizure Detection, 646-649.
[8]. Lee, Y.-S., & Tong, L.-I. (2011). Forecasting time series using a methodology based on autoregressive integrated moving average and genetic programming. Knowledge-Based Systems, 24(1), ISSN 0950-7051.
[9]. https://www.kaggle.com/datasets/anubhavgoyal10/google-stock-prices-since-the-pandemic-started
[10]. Charbuty, B., & Abdulazeez, A. (2021). Classification based on decision tree algorithm for machine learning. Journal of Applied Science and Technology Trends, 2(01), 20-28.
[11]. Biau, G., & Scornet, E. (2016). A random forest guided tour. Test, 25, 197-227.
[12]. Segal, M. R. (2004). Machine learning benchmarks and random forest regression.
[13]. Reis, I., Baron, D., & Shahaf, S. (2018). Probabilistic random forest: A machine learning algorithm for noisy data sets. The Astronomical Journal, 157(1), 16.
[14]. Yu, Y., Si, X., Hu, C., & Zhang, J. (2019). A review of recurrent neural networks: LSTM cells and network architectures. Neural Computation, 31(7), 1235-1270.
[15]. Staudemeyer, R. C., & Morris, E. R. (2019). Understanding LSTM – a tutorial into long short-term memory recurrent neural networks. arXiv preprint arXiv:1909.09586.
[16]. Sherstinsky, A. (2020). Fundamentals of recurrent neural network (RNN) and long short-term memory (LSTM) network. Physica D: Nonlinear Phenomena, 404, 132306.
[17]. Noorian, A. M., Moradi, I., & Kamali, G. A. (2008). Evaluation of 12 models to estimate hourly diffuse irradiation on inclined surfaces. Renewable Energy, 33(6), 1406-1412.
[18]. Yi, Q., Wang, F., Bao, A., & Jiapaer, G. (2014). Leaf and canopy water content estimation in cotton using hyperspectral indices and radiative transfer models. International Journal of Applied Earth Observation and Geoinformation, 33, 67-75.
[19]. Berrar, D. (2019). Cross-validation.
[20]. Picard, R. R., & Cook, R. D. (1984). Cross-validation of regression models. Journal of the American Statistical Association, 79(387), 575-583.
Cite this article
Wang,Z. (2023). Google’s Stock Price in Covid-19: An Exploration of Machine Learning Techniques for Prediction. Advances in Economics, Management and Political Sciences,46,233-241.
Data availability
The datasets used and/or analyzed during the current study will be available from the authors upon reasonable request.
Disclaimer/Publisher's Note
The statements, opinions and data contained in all publications are solely those of the individual author(s) and contributor(s) and not of EWA Publishing and/or the editor(s). EWA Publishing and/or the editor(s) disclaim responsibility for any injury to people or property resulting from any ideas, methods, instructions or products referred to in the content.
About volume
Volume title: Proceedings of the 2nd International Conference on Financial Technology and Business Analysis
© 2024 by the author(s). Licensee EWA Publishing, Oxford, UK. This article is an open access article distributed under the terms and
conditions of the Creative Commons Attribution (CC BY) license. Authors who
publish this series agree to the following terms:
1. Authors retain copyright and grant the series right of first publication with the work simultaneously licensed under a Creative Commons
Attribution License that allows others to share the work with an acknowledgment of the work's authorship and initial publication in this
series.
2. Authors are able to enter into separate, additional contractual arrangements for the non-exclusive distribution of the series's published
version of the work (e.g., post it to an institutional repository or publish it in a book), with an acknowledgment of its initial
publication in this series.
3. Authors are permitted and encouraged to post their work online (e.g., in institutional repositories or on their website) prior to and
during the submission process, as it can lead to productive exchanges, as well as earlier and greater citation of published work (See
Open access policy for details).