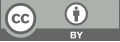
Development and Comparison of Regression Models Predicting Wine Quality using Excel - From a Profit Maximization Perspective
- 1 Economics and Management School, Wuhan University, Wuhan, 430071, China
- 2 Ningbo University of Technology, Ningbo, 315211, China
- 3 College of Liberal Arts, University of Minnesota twin cities, Minneapolis,55455, United States
- 4 School of Science, University of Rensselaer Polytechnic Institute, Troy, 12180, US
* Author to whom correspondence should be addressed.
Abstract
Wine reviewers and the general public usually determine the quality of the wine. There is a great deal of subjec-tivity in such reviews and differences in the reviewers' preferences, making it difficult for the winery to obtain favorable information. This paper will use Excel to build a related model of chemical substances in wine and wine quality. Both linear and logistic regression is used in this article to predict wine quality. In addition, differ-ent from past literature that indicates the quality of red wine based on chemical substances, this paper creatively constructs a profit model based on predicting wine quality. It thus helps wine sellers to make model selections. All these can help producers understand how to make good wine and get higher profits.
Keywords
linear regression, logistic regression, wine quality, profit maximization
[1]. Trei, Lisa, and Lisa Trei. Price Changes Way People Experience Wine, Study Finds. Stanford University, January 16, 2008. https://news.stanford.edu/news/2008/january16/wine-011608.html.
[2]. Horowitz I, Lockshin L. What Price Quality? An Investigation into the Prediction of Wine-quality Ratings. Journal of Wine Research. 2002; 13: 7-29
[3]. Antonio, Capurso. The Six Attributes of Quality in Wine. Wine And Other Stories. 24 Jan. 2020
[4]. Kwak, Young-Sik, Yoon-Jung Nam, Jae-Won Hong. Effect of Online Collective Intelligence in Wine Industry: Focus on Correlation between Wine Quality Ratings and on-Premise Prices
[5]. Badole, Mayur. Wine Quality Prediction Using Machine Learning: Predicting Wine Quality. Analytics Vidhya, 25 July 2022
[6]. Yogesh Gupta. Selection of important features and predicting wine quality using machine learning tech-niques. Procedia Computer Science. 2018; 125: 305-312
[7]. Niggl, Dennis. Predict Red Wine Quality. Kaggle
[8]. Tingwei, Zhou. Red Wine Quality Prediction through Active Learning. Journal of Physics: Conference Series 1966, no. 1 (2021): 012021.
[9]. Shaw, B., Suman, A.K., Chakraborty, B. (2020). Wine Quality Analysis Using Machine Learning. In: Mandal, J., Bhattacharya, D. (eds) Emerging Technology in Modelling and Graphics. Advances in Intelligent Systems and Computing, vol 937
[10]. Kothawade, Rohan Dilip. Wine Quality Prediction Model Using Machine Learning Techniques, n.d.
Cite this article
Xie,W.;Hu,S.;Ji,J.;Chen,Q. (2023). Development and Comparison of Regression Models Predicting Wine Quality using Excel - From a Profit Maximization Perspective. Advances in Economics, Management and Political Sciences,6,33-42.
Data availability
The datasets used and/or analyzed during the current study will be available from the authors upon reasonable request.
Disclaimer/Publisher's Note
The statements, opinions and data contained in all publications are solely those of the individual author(s) and contributor(s) and not of EWA Publishing and/or the editor(s). EWA Publishing and/or the editor(s) disclaim responsibility for any injury to people or property resulting from any ideas, methods, instructions or products referred to in the content.
About volume
Volume title: Proceedings of the 2022 International Conference on Financial Technology and Business Analysis (ICFTBA 2022), Part 2
© 2024 by the author(s). Licensee EWA Publishing, Oxford, UK. This article is an open access article distributed under the terms and
conditions of the Creative Commons Attribution (CC BY) license. Authors who
publish this series agree to the following terms:
1. Authors retain copyright and grant the series right of first publication with the work simultaneously licensed under a Creative Commons
Attribution License that allows others to share the work with an acknowledgment of the work's authorship and initial publication in this
series.
2. Authors are able to enter into separate, additional contractual arrangements for the non-exclusive distribution of the series's published
version of the work (e.g., post it to an institutional repository or publish it in a book), with an acknowledgment of its initial
publication in this series.
3. Authors are permitted and encouraged to post their work online (e.g., in institutional repositories or on their website) prior to and
during the submission process, as it can lead to productive exchanges, as well as earlier and greater citation of published work (See
Open access policy for details).