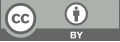
Machine Learning in Finance: A Case Study on Forecasting Google's Stock Price
- 1 Smith School of Business, Queen's University, Kingston, Canada
* Author to whom correspondence should be addressed.
Abstract
Stock price prediction remains an attractive and essential area in financial markets, with researchers constantly working hard to improve existing models or develop new ones to achieve more accurate predictions. Foreseeing the future direction of stock prices allowing people to plan and formulate effective investment strategies. However, predicting stock prices remains a difficult challenge due to many uncontrollable factors. Traditional forecasting methods rely primarily on economic data analysis and formulation. However, these traditional methods often provide limited information and forecast accuracy due to market uncertainty. Many business organizations and individual investors started to utilize the programmed approaches to improve the accuracy of stock price predictions as machine learning and deep learning capabilities continue to advance. Through an actual case study, this essay examines the innovative use of machine learning methods for researching in the field of predicting stock prices. In the case study, an LSTM model is built to find the transforming trend of the stock price, while Google’s stock price is collected to use as the dataset for training the model. The article finally conducts a comparative study on stock price prediction based on LSTM is conducted to clarify its working progress and accuracy of the outcome.
Keywords
Stock price prediction, Machine learning algorithm, LSTM
[1]. Masoud, N. M. (2013). The impact of stock market performance upon economic growth. International Journal of Economics and Financial Issues, 3(4), 788-798.
[2]. Ariyo, A. A., Adewumi, A. O., Ayo, C. K. (2014). Stock price prediction using the ARIMA model. In 2014 UKSim-AMSS 16th international conference on computer modelling and simulation (pp. 106-112). IEEE.
[3]. Nabipour, M., Nayyeri, P., Jabani, H., Mosavi, A., Salwana, E. (2020). Deep learning for stock market prediction. Entropy, 22(8), 840.
[4]. Picasso, A., Merello, S., Ma, Y., Oneto, L., Cambria, E. (2019). Technical analysis and sentiment embeddings for market trend prediction. Expert Systems with Applications, 135, 60-70.
[5]. Vijh, M., Chandola, D., Tikkiwal, V. A., Kumar, A. (2020). Stock closing price prediction using machine learning techniques. Procedia computer science, 167, 599-606.
[6]. Jordan, M. I., Mitchell, T. M. (2015). Machine learning: Trends, perspectives, and prospects. Science, 349(6245), 255-260.
[7]. Yu, Y., Si, X., Hu, C., Zhang, J. (2019). A review of recurrent neural networks: LSTM cells and network architectures. Neural computation, 31(7), 1235-1270.
[8]. Rouf, N., Malik, M. B., Arif, T., Sharma, S., Singh, S., Aich, S., Kim, H. C. (2021). Stock market prediction using machine learning techniques: a decade survey on methodologies, recent developments, and future directions. Electronics, 10(21), 2717.
[9]. Shah, D., Isah, H., Zulkernine, F. (2019). Stock market analysis: A review and taxonomy of prediction techniques. International Journal of Financial Studies, 7(2), 26.
[10]. Singh, D., Singh, B. (2020). Investigating the impact of data normalization on classification performance. Applied Soft Computing, 97, 105524.
Cite this article
Kong,T. (2023). Machine Learning in Finance: A Case Study on Forecasting Google's Stock Price. Advances in Economics, Management and Political Sciences,65,31-35.
Data availability
The datasets used and/or analyzed during the current study will be available from the authors upon reasonable request.
Disclaimer/Publisher's Note
The statements, opinions and data contained in all publications are solely those of the individual author(s) and contributor(s) and not of EWA Publishing and/or the editor(s). EWA Publishing and/or the editor(s) disclaim responsibility for any injury to people or property resulting from any ideas, methods, instructions or products referred to in the content.
About volume
Volume title: Proceedings of the 2nd International Conference on Financial Technology and Business Analysis
© 2024 by the author(s). Licensee EWA Publishing, Oxford, UK. This article is an open access article distributed under the terms and
conditions of the Creative Commons Attribution (CC BY) license. Authors who
publish this series agree to the following terms:
1. Authors retain copyright and grant the series right of first publication with the work simultaneously licensed under a Creative Commons
Attribution License that allows others to share the work with an acknowledgment of the work's authorship and initial publication in this
series.
2. Authors are able to enter into separate, additional contractual arrangements for the non-exclusive distribution of the series's published
version of the work (e.g., post it to an institutional repository or publish it in a book), with an acknowledgment of its initial
publication in this series.
3. Authors are permitted and encouraged to post their work online (e.g., in institutional repositories or on their website) prior to and
during the submission process, as it can lead to productive exchanges, as well as earlier and greater citation of published work (See
Open access policy for details).