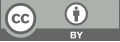
Spatial analysis and machine learning: Towards integrated predictive modeling advancements
- 1 The University of Adelaide
- 2 The University of Newcastle
* Author to whom correspondence should be addressed.
Abstract
This paper presents a comprehensive exploration of the integration of spatial analysis with machine learning techniques, aiming to enhance predictive modeling capabilities across various domains. Spatial analysis, a methodological approach for understanding geographic patterns and relationships, when combined with the computational power of machine learning, offers unprecedented opportunities for analyzing complex spatial datasets. Through quantitative analysis and the application of mathematical models, this study demonstrates the effectiveness of this integration in improving the accuracy and efficiency of predictive models. The research encompasses a range of applications, from environmental monitoring to urban planning and public health, showcasing the versatility and potential of combining spatial analysis with machine learning.
Keywords
Spatial Analysis, Machine Learning, Predictive Modeling, Geographic Information Systems (GIS), Environmental Monitoring
[1]. Dumelle, Michael, et al. "spsurvey: Spatial Sampling Design and Analysis in R." Journal of Statistical Software 105 (2023): 1-29.
[2]. Pebesma, Edzer, and Roger Bivand. Spatial data science: With applications in R. CRC Press, 2023.
[3]. Mandel, Lauren Heather, Bradley Wade Bishop, and Ashley Marie Orehek. "A new decade of uses for geographic information systems (GIS) as a tool to research, measure and analyze library services." Library Hi Tech 41.4 (2023): 1022-1038.
[4]. Ukoba, M. O., et al. "Optimal sites for agricultural and forest residues energy conversion plant using geographic information system." Heliyon 9.9 (2023).
[5]. Moein, Mohammad Mohtasham, et al. "Predictive models for concrete properties using machine learning and deep learning approaches: A review." Journal of Building Engineering 63 (2023): 105444.
[6]. Nazar, Sohaib, et al. "Machine learning interpretable-prediction models to evaluate the slump and strength of fly ash-based geopolymer." Journal of Materials Research and Technology 24 (2023): 100-124.
[7]. Mirzakarimova, G. M. "REMOTE SENSING DATA: INTERNATIONAL EXРERIENCES AND AРРLICATIONS." ITALY" ACTUAL PROBLEMS OF SCIENCE AND EDUCATION IN THE FACE OF MODERN CHALLENGES". 14.1 (2023).
[8]. Sherif, Khaled, et al. "Deep Learning in IoT: An LSTM Approach for NDVI Forecasting." 2023 3rd International Conference on Electronic Engineering (ICEEM). IEEE, 2023.
Cite this article
Lu,X.;Li,Z. (2024). Spatial analysis and machine learning: Towards integrated predictive modeling advancements. Theoretical and Natural Science,36,152-157.
Data availability
The datasets used and/or analyzed during the current study will be available from the authors upon reasonable request.
Disclaimer/Publisher's Note
The statements, opinions and data contained in all publications are solely those of the individual author(s) and contributor(s) and not of EWA Publishing and/or the editor(s). EWA Publishing and/or the editor(s) disclaim responsibility for any injury to people or property resulting from any ideas, methods, instructions or products referred to in the content.
About volume
Volume title: Proceedings of the 2nd International Conference on Mathematical Physics and Computational Simulation
© 2024 by the author(s). Licensee EWA Publishing, Oxford, UK. This article is an open access article distributed under the terms and
conditions of the Creative Commons Attribution (CC BY) license. Authors who
publish this series agree to the following terms:
1. Authors retain copyright and grant the series right of first publication with the work simultaneously licensed under a Creative Commons
Attribution License that allows others to share the work with an acknowledgment of the work's authorship and initial publication in this
series.
2. Authors are able to enter into separate, additional contractual arrangements for the non-exclusive distribution of the series's published
version of the work (e.g., post it to an institutional repository or publish it in a book), with an acknowledgment of its initial
publication in this series.
3. Authors are permitted and encouraged to post their work online (e.g., in institutional repositories or on their website) prior to and
during the submission process, as it can lead to productive exchanges, as well as earlier and greater citation of published work (See
Open access policy for details).