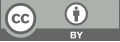
Towards python program repair with generative pre-trained transformer (GPT-3.5)
- 1 University of Michigan
- 2 Dalian University of Technology
* Author to whom correspondence should be addressed.
Abstract
ChatGPT has a great potential using a simple prompt design, which means further studies can be done to investigate the effect of different prompt designs. Complex software often contains hidden bugs in its source code. Recent study suggests that OpenAI’s ChatGPT can perform numerous operations including code-to-code operations like code completion, translation, repair, and summarization, along with language-to-code operations such as code explanation and search. ChatGPT’s dialogue capability can assist in generating more accurate bug fixes. However, it sometimes offers solutions without seeking further information, which can mislead users. To address this issue and enhance user experience, we conducted three design iterations to develop “D-bugger” — a system enabling programmers to fix bugs more effectively. We conducted a survey to gauge the need for refinement, designed a low-fidelity prototype with key features, and then created a high-fidelity prototype evaluated by Python users. Our work aims to enhance the debugging process and user engagement.
Keywords
Program repair, ChatGPT, Misleading rate
[1]. Philipp Hacker, Andreas Engel, and Marco Mauer. 2023. Regulating ChatGPT and other Large Generative AI Models. In Proceedings of the 2023 ACM Conference on Fairness, Accountability, and Transparency (FAccT ‘23). Association for Computing Machinery, New York, NY, USA, 1112–1123. https://doi.org/10.1145/3593013.3594067
[2]. Fan Huang, Haewoon Kwak, and Jisun An. 2023. Is ChatGPT better than Human Annotators? Potential and Limitations of ChatGPT in Explaining Implicit Hate Speech. In Companion Proceedings of the ACM Web Conference 2023 (WWW ‘23 Companion). Association for Computing Machinery, New York, NY, USA, 294–297. https://doi.org/10.1145/ 3543873.3587368
[3]. Majeed Kazemitabaar, Justin Chow, Carl Ka To Ma, Barbara J. Ericson, David Weintrop, and Tovi Grossman. 2023. Studying the effect of AI Code Generators on Supporting Novice Learners in Introductory Programming. In Proceedings of the 2023 CHI Conference on Human Factors in Computing Systems (CHI ‘23). Association for Computing Machinery, New York, NY, USA, Article 455, 1–23. https://doi.org/10.1145/3544548.3580919
[4]. Dominik Sobania, Martin Briesch, Carol Hanna, Justyna Petke. 2023. An Analysis of the Automatic Bug Fixing Performance of ChatGPT
[5]. Yao Xie, Melody Chen, David Kao, Ge Gao, Xiang Anthony Chen. 2020. CheXplain: Enabling Physicians to Explore and Understand Data-driven, AI-Enabled Medical Imaging Analysis (CHI 2020)
Cite this article
Cao,Z.;Ren,Q. (2024). Towards python program repair with generative pre-trained transformer (GPT-3.5). Theoretical and Natural Science,43,102-112.
Data availability
The datasets used and/or analyzed during the current study will be available from the authors upon reasonable request.
Disclaimer/Publisher's Note
The statements, opinions and data contained in all publications are solely those of the individual author(s) and contributor(s) and not of EWA Publishing and/or the editor(s). EWA Publishing and/or the editor(s) disclaim responsibility for any injury to people or property resulting from any ideas, methods, instructions or products referred to in the content.
About volume
Volume title: Proceedings of the 3rd International Conference on Computing Innovation and Applied Physics
© 2024 by the author(s). Licensee EWA Publishing, Oxford, UK. This article is an open access article distributed under the terms and
conditions of the Creative Commons Attribution (CC BY) license. Authors who
publish this series agree to the following terms:
1. Authors retain copyright and grant the series right of first publication with the work simultaneously licensed under a Creative Commons
Attribution License that allows others to share the work with an acknowledgment of the work's authorship and initial publication in this
series.
2. Authors are able to enter into separate, additional contractual arrangements for the non-exclusive distribution of the series's published
version of the work (e.g., post it to an institutional repository or publish it in a book), with an acknowledgment of its initial
publication in this series.
3. Authors are permitted and encouraged to post their work online (e.g., in institutional repositories or on their website) prior to and
during the submission process, as it can lead to productive exchanges, as well as earlier and greater citation of published work (See
Open access policy for details).