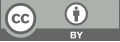
Prediction of building carbon emission based on grey Markov theory
- 1 Tianjin Chengjian University
* Author to whom correspondence should be addressed.
Abstract
The increase in carbon emissions in the construction industry is one of the factors that lead to environmental problems and hinder the sustainable development of society and the construction industry. Therefore, there is an urgent need to rationally control carbon emissions by formulating scientific and effective energy-saving and emission reduction policies so as to safeguard the development of the industry. However, because of the uncertainty and complexity of CO2 emissions, more reliable prediction and assessment tools are needed to comprehensively analyze and predict CO2 emissions. This study combines the gray system theory and Markov principle to give full play to the advantages of the two methods, using Markov theory to determine the state transition probability, find out the characteristics of carbon emissions in the construction industry, and form a suitable gray Markov prediction model. The feasibility of the prediction model is demonstrated by calculating and examining the prediction model using data related to carbon emissions from the U.S. buildings from 2002 to 2022, which greatly improves the accuracy of CO2 emissions prediction.
Keywords
carbon emission, building, construction industry, Grey Markov prediction model
[1]. Javanmard, M. E., Ghaderi, S. F., & Hoseinzadeh, M. (2021). Data mining with 12 machine learning algorithms for predict costs and carbon dioxide emission in integrated energy-water optimization model in buildings. Energy Conversion and Management, 238, 114153.
[2]. Ahmed Ali, K., Ahmad, M. I., & Yusup, Y. (2020). Issues, impacts, and mitigations of carbon dioxide emissions in the building sector. Sustainability, 12(18), 7427.
[3]. Atmaca, A., & Atmaca, N. (2016). Comparative life cycle energy and cost analysis of post-disaster temporary housings. Applied energy, 171, 429-443.
[4]. Atmaca, N., Atmaca, A., & Özçetin, A. İ. (2021). The impacts of restoration and reconstruction of a heritage building on life cycle energy consumption and related carbon dioxide emissions. Energy and Buildings, 253, 111507.
[5]. Zhou, W., Zeng, B., Wang, J., Luo, X., & Liu, X. (2021). Forecasting Chinese carbon emissions using a novel grey rolling prediction model. Chaos, Solitons & Fractals, 147, 110968.
[6]. Fang, Y., Lu, X., & Li, H. (2021). A random forest-based model for the prediction of construction-stage carbon emissions at the early design stage. Journal of Cleaner Production, 328, 129657.
[7]. Xie. Z., Gao. X., Yuan. W., Fang. J., and Jiang. Z, (2020). Decomposition and prediction of direct residential carbon emission indicators in Guangdong Province of China. Ecological Indicators, 115, 106344.
[8]. Wu, R., Wang, J., Wang, S., & Feng, K. (2021). The drivers of declining CO2 emissions trends in developed nations using an extended STIRPAT model: A historical and prospective analysis. Renewable and Sustainable Energy Reviews, 149, 111328..
[9]. Kumar, S., Shukla, A. K., & Muhuri, P. K. (2021). Anomaly based novel multi-source unsupervised transfer learning approach for carbon emission centric GDP prediction. Computers in Industry, 126, 103396.
[10]. Jia, Z. Q., Zhou, Z. F., Zhang, H. J., Li, B., & Zhang, Y. X. (2020). Forecast of coal consumption in Gansu Province based on Grey-Markov chain model. Energy, 199, 117444.
Cite this article
Zhao,C. (2024). Prediction of building carbon emission based on grey Markov theory. Theoretical and Natural Science,48,124-132.
Data availability
The datasets used and/or analyzed during the current study will be available from the authors upon reasonable request.
Disclaimer/Publisher's Note
The statements, opinions and data contained in all publications are solely those of the individual author(s) and contributor(s) and not of EWA Publishing and/or the editor(s). EWA Publishing and/or the editor(s) disclaim responsibility for any injury to people or property resulting from any ideas, methods, instructions or products referred to in the content.
About volume
Volume title: Proceedings of the 2nd International Conference on Environmental Geoscience and Earth Ecology
© 2024 by the author(s). Licensee EWA Publishing, Oxford, UK. This article is an open access article distributed under the terms and
conditions of the Creative Commons Attribution (CC BY) license. Authors who
publish this series agree to the following terms:
1. Authors retain copyright and grant the series right of first publication with the work simultaneously licensed under a Creative Commons
Attribution License that allows others to share the work with an acknowledgment of the work's authorship and initial publication in this
series.
2. Authors are able to enter into separate, additional contractual arrangements for the non-exclusive distribution of the series's published
version of the work (e.g., post it to an institutional repository or publish it in a book), with an acknowledgment of its initial
publication in this series.
3. Authors are permitted and encouraged to post their work online (e.g., in institutional repositories or on their website) prior to and
during the submission process, as it can lead to productive exchanges, as well as earlier and greater citation of published work (See
Open access policy for details).