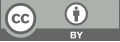
Research on gold price prediction based on ARIMA model
- 1 College of Science, Civil Aviation University of China, Tianjin, 300300, China
* Author to whom correspondence should be addressed.
Abstract
Gold is one of the most prevalent currencies in the world and its price has a very strong influence in the global financial markets. Gold has safe-haven properties, which can have a significant impact on its demand and price, especially in times of social unrest or financial crisis. Now, the demand for gold by investors has increased dramatically. Therefore, being able to accurately predict the direction of the gold price can help investors to effectively develop investment strategies and risk management measures. The overall objective of this study is to forecast the price of gold futures for the next six months. In this study, the Kaggle website was searched to find the price of gold from 2020 to 2024 and finally the CLOSE price was chosen as the final predicted price. This paper uses the ARIMA model for gold price forecasting. By comparing the RSME size of each model, ARIMA (1, 1, 2) is finally chosen. From the prediction results the price of gold remains stable in the first half of the year and then increases significantly. From the results of the residual test, there is no autocorrelation, and then it is white noise.
Keywords
Gold, ARIMA, autocorrelation, prediction
[1]. Yang C 2023 International gold futures forecast based on VMD-LASSO-LSTM. Wuhan University of Science and Technology.
[2]. Abu D I, et al. 2023 Enhancing multilayer perceptron neural network using archive-based harris hawks optimizer to predict gold prices. J. King Saud Univ.-Comput. Inf. Sci.
[3]. Liu C Y 2023 Research on Forecasting of Gold Futures Price Based on LASSO-QRNN. Central South University.
[4]. Yang T T 2023 Research on price discovery and volatility spillover effect in China’s gold market. East China University of Political Science and Law.
[5]. Yang X Y 2023 Gold futures price prediction based on CNN-BiGRU model. Henan University.
[6]. Tully E and Lucey B M 2007 A power GARCH examination of the gold market. Research in International Business & Finance, 21(2), 316-325.
[7]. Lian Z, et al. 2010 Improved gold price prediction based on BP neural network. Computer Integrated Manufacturing Systems, 27(9), 200-203.
[8]. Singh G, Tripathy B and Singh J 2023 An Evaluation of Extreme Learning Machine Algorithm to Forecasting the Gold Price. 2023 7th International Conference on Electronics, Communication and Aerospace Technology (ICECA), Coimbatore, India, 523-529.
[9]. Rapach D E, Strauss J K and Zhou G 2010 Out-of-Sample equity premium prediction: Combination forecasts and links to the real economy. Review of Financial Studies, 23(2), 821–862.
[10]. Jin W 2022 Gold futures price prediction research based on CEEMDAN-GRU. Beijing Jiaotong University.
Cite this article
Bai,Y. (2024). Research on gold price prediction based on ARIMA model. Theoretical and Natural Science,52,41-48.
Data availability
The datasets used and/or analyzed during the current study will be available from the authors upon reasonable request.
Disclaimer/Publisher's Note
The statements, opinions and data contained in all publications are solely those of the individual author(s) and contributor(s) and not of EWA Publishing and/or the editor(s). EWA Publishing and/or the editor(s) disclaim responsibility for any injury to people or property resulting from any ideas, methods, instructions or products referred to in the content.
About volume
Volume title: Proceedings of CONF-MPCS 2024 Workshop: Quantum Machine Learning: Bridging Quantum Physics and Computational Simulations
© 2024 by the author(s). Licensee EWA Publishing, Oxford, UK. This article is an open access article distributed under the terms and
conditions of the Creative Commons Attribution (CC BY) license. Authors who
publish this series agree to the following terms:
1. Authors retain copyright and grant the series right of first publication with the work simultaneously licensed under a Creative Commons
Attribution License that allows others to share the work with an acknowledgment of the work's authorship and initial publication in this
series.
2. Authors are able to enter into separate, additional contractual arrangements for the non-exclusive distribution of the series's published
version of the work (e.g., post it to an institutional repository or publish it in a book), with an acknowledgment of its initial
publication in this series.
3. Authors are permitted and encouraged to post their work online (e.g., in institutional repositories or on their website) prior to and
during the submission process, as it can lead to productive exchanges, as well as earlier and greater citation of published work (See
Open access policy for details).