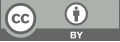
RETRACTED ARTICLE:Research Progress on 2D Human Pose Estimation Based on Deep Learning
- 1 School of Information and Communication Engineering, University of Electronic Science and Technology of China, Chengdu, China
* Author to whom correspondence should be addressed.
Abstract
Human pose estimation (HPE) is a significant area of study within computer vision and artificial intelligence, which locates the position and pose of human joints through images or videos. HPE has advanced progress in computer vision and deep learning fields at the theoretical level, improved the efficiency and accuracy of neural networks, and supported the development of natural and efficient human-computer interaction systems. At the application level, HPE has extensive value in many fields, including security monitoring, motion analysis and health management, VR&AR, robotics technology, film and television production and animation, intelligent driving and assisted driving. This paper divides 2D HPE tasks into three categories: two-stage (top-down and bottom-up), single-stage, and other improvement strategies (Transformer-based methods and post-processing optimization methods). Although deep learning-based pose estimation algorithms have achieved remarkable results, they still face challenges, such as prediction in complex scenes, crowd and joint occlusion problems, and lightweight design of models. Future work should pay more attention to these challenges and extend pose estimation technology from 2D to 3D contexts.
Keywords
Human Pose Estimation, Deep Learning, Multi-person pose estimation, Body part detectors
[1]. Wang, L., Wu, J., Zhou, Z. M., Zhao, X., Liu, Y. C. (2015). Human action recognition through part-configured human detection response feature maps. Journal of Software, 26, 128−136.
[2]. Zheng, C., Wu, W. H., Chen, C., et al. (2023). Deep learning-based human pose estimation: A survey. ACM Computing Surveys, 56(1), 1-37.
[3]. Geng, Z. G., Wang, C. Y., Wei, Y. X., et al. (2023). Human pose as compositional tokens. Proceedings of the IEEE/CVF Conference on Computer Vision and Pattern Recognition, 660-671.
[4]. Yang, J., Zeng, A. L., Liu, S., et al. (2023). Explicit box detection unifies end-to-end multi-person pose estimation. arXiv preprint arXiv, 2302.01593.
[5]. Jiang, T., Lu, P., Zhang, L., et al. (2023). Rtmpose: Real-time multi-person pose estimation based on mmpose. arXiv preprint arXiv, 2303.07399.
[6]. Fang, H. S., Xie, S. Q., Tai, Y. W., et al. (2017). Rmpe: Regional multi-person pose estimation. Proceedings of the IEEE international conference on computer vision, 2334-2343.
[7]. Sun, K., Xiao, B., Liu, D., et al. (2019). Deep high-resolution representation learning for human pose estimation. Proceedings of the IEEE/CVF conference on computer vision and pattern recognition, 5693-5703.
[8]. Zhang, F., Zhu, X. T., Dai, H. B., et al. (2020). Distribution-aware coordinate representation for human pose estimation. Proceedings of the IEEE/CVF conference on computer vision and pattern recognition, 7093-7102.
[9]. Nibali, A., He, Z., Morgan, S., et al. (2018). Numerical coordinate regression with convolutional neural networks. arXiv preprint arXiv, 1801.07372.
[10]. Nibali, A., He, Z., Morgan, S., et al. (2018). Numerical coordinate regression with convolutional neural networks. arXiv preprint arXiv, 1801.07372.
[11]. Cheng, B. W., Xiao, B., Wang, J. D., et al. (2020). Higherhrnet: Scale-aware representation learning for bottom-up human pose estimation. Proceedings of the IEEE/CVF conference on computer vision and pattern recognition, 5386-5395.
[12]. Luo, Z. X., Wang, Z. C., Huang, Y., et al. (2021). Rethinking the heatmap regression for bottom-up human pose estimation. Proceedings of the IEEE/CVF conference on computer vision and pattern recognition, 13264-13273.
[13]. Yu, H., Du, C. J., & Yu, L. (2022). Scale-aware heatmap representation for human pose estimation. Pattern Recognition Letters, 154, 1-6.
[14]. Nie, X. C., Feng, J. S, Zhang, J. F., et al. (2019). Single-stage multi-person pose machines. Proceedings of the IEEE/CVF international conference on computer vision, 6951-6960.
[15]. McNally, W., Vats, K., Wong, A., et al. (2022). Rethinking keypoint representations: Modeling keypoints and poses as objects for multi-person human pose estimation. European Conf. on Computer Vision. Cham: Springer Nature Switzerland, 37-54.
[16]. Lu, P., Jiang, T., Li, Y. N., et al. (2024). RTMO: Towards High-Performance One-Stage Real-Time Multi-Person Pose Estimation. Proceedings of the IEEE/CVF Conference on Computer Vision and Pattern Recognition, 1491-1500.
[17]. Yang, S., Quan, Z. B., Nie, M., & Yang, W. K. (2021). TransPose: Keypoint localization via transformer. Proceedings of the IEEE/CVF international conference on computer vision, 11802-11812.
[18]. Xu, Y. F., Zhang, J., Zhang, Q. M., et al. (2022). Vitpose: Simple vision transformer baselines for human pose estimation. Advances in Neural Information Processing Systems, 35, 38571-38584.
[19]. Fieraru, M., Khoreva, A., Pishchulin, L., et al. (2018). Learning to refine human pose estimation. Proceedings of the IEEE conference on computer vision and pattern recognition workshops, 205-214.
[20]. Moon, G., Chang, J. Y., Lee, K. M. (2019). Posefix: Model-agnostic general human pose refinement network. Proceedings of the IEEE/CVF Conference on Computer Vision and Pattern Recognition, 7773-7781.
[21]. Bin, Y., Chen, Z. M., Wei, X. S., et al. (2020). Structure-aware human pose estimation with graph convolutional networks. Pattern Recognition, 106, 107410.
Cite this article
Liu,H. (2024). RETRACTED ARTICLE:Research Progress on 2D Human Pose Estimation Based on Deep Learning. Theoretical and Natural Science,41,193-195.
Data availability
The datasets used and/or analyzed during the current study will be available from the authors upon reasonable request.
Disclaimer/Publisher's Note
The statements, opinions and data contained in all publications are solely those of the individual author(s) and contributor(s) and not of EWA Publishing and/or the editor(s). EWA Publishing and/or the editor(s) disclaim responsibility for any injury to people or property resulting from any ideas, methods, instructions or products referred to in the content.
About volume
Volume title: Proceedings of the 2nd International Conference on Mathematical Physics and Computational Simulation
© 2024 by the author(s). Licensee EWA Publishing, Oxford, UK. This article is an open access article distributed under the terms and
conditions of the Creative Commons Attribution (CC BY) license. Authors who
publish this series agree to the following terms:
1. Authors retain copyright and grant the series right of first publication with the work simultaneously licensed under a Creative Commons
Attribution License that allows others to share the work with an acknowledgment of the work's authorship and initial publication in this
series.
2. Authors are able to enter into separate, additional contractual arrangements for the non-exclusive distribution of the series's published
version of the work (e.g., post it to an institutional repository or publish it in a book), with an acknowledgment of its initial
publication in this series.
3. Authors are permitted and encouraged to post their work online (e.g., in institutional repositories or on their website) prior to and
during the submission process, as it can lead to productive exchanges, as well as earlier and greater citation of published work (See
Open access policy for details).