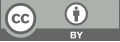
Correlation between obesity and income: A statistical analysis using linear regression models
- 1 Shanghai American School, Shanghai, 200000, China
* Author to whom correspondence should be addressed.
Abstract
The rate of obesity across the world has seen a steady increase in the past several decades. This disease can lead to significant harm associated with one’s body functioning and mental health. The potential causes of obesity incorporate social, environmental, and personal factors. This article attempts to cover the correlation between obesity and income level using a linear regression model. Samples were extracted from the National Health and Nutrition Examination Survey about 545 individuals from 2017 to 2020. The dataset was subdivided into four cohorts, which include non-Hispanic White male, non-Hispanic White female, non-Hispanic Black male, and non-Hispanic Black female, respectively, to avoid variables that might confound with the explanatory variable. The study found out that the correlation for White male, White female, and Black female can be either positive, negative, or non-existent, and positive on the other hand for the Black male cohort. However, the study concluded that the linear regression model is ineffective for the topic of analysis as the metrics indicate. The incompatibility of the model can be attributed to the fact that other variables that can possibly confound with the explanatory variable are neglected and not filtered out.
Keywords
Obesity, BMI, income-to-poverty ratio, linear regression, confounding variables.
[1]. Pi-Sunyer X 2009 The medical risks of obesity. Postgraduate Medicine, 121(6), 21-33.
[2]. Kelly T, et al. 2008 Global burden of obesity in 2005 and projections to 2030. International Journal of Obesity, 32(9), 1431-1437.
[3]. Safaei M, Sundararajan E A, Driss M, Boulila W and Shapi A 2021 A systematic literature review on obesity: Understanding the causes & Consequences of obesity and reviewing various machine learning approaches used to predict obesity. Computers in Biology and Medicine, 136.
[4]. Hu L, et al. (2017). Prevalence of overweight, obesity, abdominal obesity and obesity-related risk factors in southern China. PLOS ONE, 12(9).
[5]. Chooi Y C, Ding C and Magkos F 2019 The epidemiology of obesity. Metabolism, 92, 6-10.
[6]. Westbury S, Oyebode O, Rens T V and Barber T M 2023 Obesity Stigma: Causes, Consequences, and Potential Solutions. Current Obesity Reports, 12, 10-23.
[7]. Nuttall F Q 2015 Body Mass Index Obesity, BMI, and Health: A Critical Review. Nutrition Today, 50(3), 117-128.
[8]. Lin X and Li H 2021 Obesity: Epidemiology, Pathophysiology, and Therapeutics. Frontiers in Endocrinology, 12.
[9]. Mahmoud R, Kimonis V and Butler M G 2022 Genetics of Obesity in Humans: A Clinical Review. International Journal of Molecular Science, 23(19).
[10]. Mathieu-Bolh N 2022 The elusive link between income and obesity. Journal of Economic Surveys, 36(4), 935-968.
[11]. Kim T J and Knesebeck O D 2017 Income and obesity: what is the direction of the relationship? A systematic review and meta-analysis. BMJ Open, 8(1).
[12]. Bruce M A, et al. 2007 One size fits all? Race, gender and body mass index among U.S. adults. Journal of the National Medical Association, 99(10), 1152-1158.
[13]. Colby S L and Ortman J M 2015 Projections of the Size and Composition of the U.S. Population: 2014 to 2060. US Cencus Bureau.
[14]. Levine J A 2011 Poverty and obesity in the U.S. Diabetes, 60(11), 2667-2668.
Cite this article
Jiang,Y. (2024). Correlation between obesity and income: A statistical analysis using linear regression models. Theoretical and Natural Science,51,1-8.
Data availability
The datasets used and/or analyzed during the current study will be available from the authors upon reasonable request.
Disclaimer/Publisher's Note
The statements, opinions and data contained in all publications are solely those of the individual author(s) and contributor(s) and not of EWA Publishing and/or the editor(s). EWA Publishing and/or the editor(s) disclaim responsibility for any injury to people or property resulting from any ideas, methods, instructions or products referred to in the content.
About volume
Volume title: Proceedings of CONF-MPCS 2024 Workshop: Quantum Machine Learning: Bridging Quantum Physics and Computational Simulations
© 2024 by the author(s). Licensee EWA Publishing, Oxford, UK. This article is an open access article distributed under the terms and
conditions of the Creative Commons Attribution (CC BY) license. Authors who
publish this series agree to the following terms:
1. Authors retain copyright and grant the series right of first publication with the work simultaneously licensed under a Creative Commons
Attribution License that allows others to share the work with an acknowledgment of the work's authorship and initial publication in this
series.
2. Authors are able to enter into separate, additional contractual arrangements for the non-exclusive distribution of the series's published
version of the work (e.g., post it to an institutional repository or publish it in a book), with an acknowledgment of its initial
publication in this series.
3. Authors are permitted and encouraged to post their work online (e.g., in institutional repositories or on their website) prior to and
during the submission process, as it can lead to productive exchanges, as well as earlier and greater citation of published work (See
Open access policy for details).