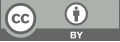
Heart disease prediction using machine learning models
- 1 ShanDong University
* Author to whom correspondence should be addressed.
Abstract
Heart disease remains a global health threat, making rapid identification crucial. Using the Heart Disease Dataset from Kaggle, this research employs a Random Forest model to analyze 13 clinical variables from 1,025 samples. To improve accuracy and address class imbalance, the dataset was split into training and test sets, utilizing methods such as Z-scores, SMOTE, and feature selection. The Random Forest model, which combines multiple decision trees, achieved high performance with an accuracy of 98.54%, identifying key predictors such as chest pain type, maximum heart rate, and thalassemia. Compared to a single decision tree, the Random Forest model reduces overfitting, improves generalization, and increases predictive accuracy. Factors like cholesterol levels, resting blood pressure, and exercise-induced angina were also considered. By averaging results from multiple trees, the model offers reliable and stable predictions, highlighting its potential in clinical settings for early detection and personalized treatment strategies. This study aims to assist healthcare providers in better allocating resources, planning preventive measures, and tailoring treatment plans to individual patients.
Keywords
Heart disease, random forest, pathogenic factors.
[1]. Salari N, Faryadras F, Shohaimi S, et al. 2024 Global prevalence of congenital heart diseases in infants: A systematic review and meta-analysis. Journal of Neonatal Nursing.
[2]. Abughazaleh S, Obeidat O, Tarawneh M, et al. 2024 Trends of Hypertensive Heart Disease Prevalence and Mortality in the United States between the period 1990-2019, Global Burden of disease Database. Current Problems in Cardiology, 102621.
[3]. Chen Y, Wei Y and Tang W 2024 The role of hydrogen in the prevention and treatment of coronary atherosclerotic heart disease. European Journal of Pharmacology, 176586.
[4]. Vora N, Patel P, Gajjar A, et al. 2023 Gene therapy for heart failure: A novel treatment for the age old disease. Disease-a-Month, 101636.
[5]. Islam M A, Majumder M Z H, Miah M S, et al. 2024 Precision healthcare: A deep dive into machine learning algorithms and feature selection strategies for accurate heart disease prediction. Computers in Biology and Medicine, 176, 108432.
[6]. Mesquita F and Marques G 2024 An explainable machine learning approach for automated medical decision support of heart disease. Data & Knowledge Engineering, 102339.
[7]. Chen L, Ji P, Ma Y, et al. 2023 Custom machine learning algorithm for large-scale disease screening-taking heart disease data as an example. Artificial Intelligence in Medicine, 146, 102688.
[8]. Ghasemieh A, Lloyed A, Bahrami P, et al. 2023 A novel machine learning model with Stacking Ensemble Learner for predicting emergency readmission of heart-disease patients. Decision Analytics Journal, 7, 100242.
[9]. Chang V, Bhavani V R, Xu A Q, et al. 2022 An artificial intelligence model for heart disease detection using machine learning algorithms. Healthcare Analytics, 2, 100016.
[10]. Sun H and Pan J 2023 Heart disease prediction using machine learning algorithms with self-measurable physical condition indicators. Journal of data analysis and information processing, 11(1), 1-10.
Cite this article
Wang,W. (2024). Heart disease prediction using machine learning models . Theoretical and Natural Science,51,9-17.
Data availability
The datasets used and/or analyzed during the current study will be available from the authors upon reasonable request.
Disclaimer/Publisher's Note
The statements, opinions and data contained in all publications are solely those of the individual author(s) and contributor(s) and not of EWA Publishing and/or the editor(s). EWA Publishing and/or the editor(s) disclaim responsibility for any injury to people or property resulting from any ideas, methods, instructions or products referred to in the content.
About volume
Volume title: Proceedings of CONF-MPCS 2024 Workshop: Quantum Machine Learning: Bridging Quantum Physics and Computational Simulations
© 2024 by the author(s). Licensee EWA Publishing, Oxford, UK. This article is an open access article distributed under the terms and
conditions of the Creative Commons Attribution (CC BY) license. Authors who
publish this series agree to the following terms:
1. Authors retain copyright and grant the series right of first publication with the work simultaneously licensed under a Creative Commons
Attribution License that allows others to share the work with an acknowledgment of the work's authorship and initial publication in this
series.
2. Authors are able to enter into separate, additional contractual arrangements for the non-exclusive distribution of the series's published
version of the work (e.g., post it to an institutional repository or publish it in a book), with an acknowledgment of its initial
publication in this series.
3. Authors are permitted and encouraged to post their work online (e.g., in institutional repositories or on their website) prior to and
during the submission process, as it can lead to productive exchanges, as well as earlier and greater citation of published work (See
Open access policy for details).