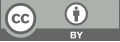
Factors influencing housing prices: A comparative study using multiple linear regression and random forest
- 1 University of Waterloo, Waterloo, N2L 3G1, Canada
* Author to whom correspondence should be addressed.
Abstract
The method is to construct a multiple linear regression model to examine the variables that significantly affect US home prices. The dataset has 13 variables and 545 observations, and it was obtained via Kaggle. This paper also considers comparing the multiple linear regression models and random forest model for predicting house prices and thus concludes which model can most accurately predict house prices. The result indicates that the factors including area, quantity of bedrooms and bathrooms, the existence of a basement, and state of the furnishings, etc. significantly impact housing prices. By comparing Root Mean Squared Error (RMSE) and Mean Absolute Percentage Error (MAPE), the conclusion suggests that the multiple linear regression model marginally surpasses the random forest regarding the accuracy of the predictions, despite the fact that both models function similarly. This research offers practical applications for enhancing real estate market strategies and provides valuable insights into the factors influencing housing prices.
Keywords
Multiple linear regression, random forest, housing prices.
[1]. Jiang Y and Qiu L 2022 Empirical study on the influencing factors of housing price-based on cross-section data of 31 provinces and cities in China. Procedia Computer Science, 199, 1498-1504.
[2]. Dong D 2021 Factors Influencing Housing Prices: An Empirical Analysis From New York City. Proceedings of the 2020 3rd International Conference on E-Business, Information Management and Computer Science, 106–113.
[3]. Zhao C and Liu F 2023 Impact of housing policies on the real estate market-Systematic literature review. Heliyon, 9(10).
[4]. Sagala N T M and Cendriawan L H 2022 House Price Prediction Using Linier Regression. 2022 IEEE 8th International Conference on Computing, Engineering and Design (ICCED), Sukabumi, Indonesia, 1-5.
[5]. Wang P Y, et al. 2021 Deep learning model for house price prediction using heterogeneous data analysis along with joint self-attention mechanism. IEEE Access, 9, 55244-55259.
[6]. Thamarai M and Malarvizhi S P 2020 House Price Prediction Modeling Using Machine Learning. International Journal of Information Engineering and Electronic Business, 12(2), 15–20.
[7]. Wang L, Wang G, Yu H and Wang F 2022 Prediction and analysis of residential house price using a flexible spatiotemporal model. Journal of Applied Economics, 25(1), 503-522.
[8]. Park B and Bae J K 2015 Using machine learning algorithms for housing price prediction: The case of Fairfax County, Virginia Housing Data. Expert Systems with Applications, 42(6), 2928-2934.
[9]. Ahtesham M, Bawany N Z and Fatima K 2020 House Price Prediction using Machine Learning Algorithm - The Case of Karachi City, Pakistan. 2020 21st International Arab Conference on Information Technology (ACIT), Giza, Egypt, 1-5.
[10]. Brannlund J, et al. 2023 Predicting changes in Canadian housing markets with Machine Learning. Bank of Canada.
[11]. Marill K A 2004 Advanced Statistics: Linear Regression, Part II: Multiple Linear Regression. Academic Emergency Medicine, 11(1), 94–102.
Cite this article
Zhong,S. (2024). Factors influencing housing prices: A comparative study using multiple linear regression and random forest. Theoretical and Natural Science,51,73-79.
Data availability
The datasets used and/or analyzed during the current study will be available from the authors upon reasonable request.
Disclaimer/Publisher's Note
The statements, opinions and data contained in all publications are solely those of the individual author(s) and contributor(s) and not of EWA Publishing and/or the editor(s). EWA Publishing and/or the editor(s) disclaim responsibility for any injury to people or property resulting from any ideas, methods, instructions or products referred to in the content.
About volume
Volume title: Proceedings of CONF-MPCS 2024 Workshop: Quantum Machine Learning: Bridging Quantum Physics and Computational Simulations
© 2024 by the author(s). Licensee EWA Publishing, Oxford, UK. This article is an open access article distributed under the terms and
conditions of the Creative Commons Attribution (CC BY) license. Authors who
publish this series agree to the following terms:
1. Authors retain copyright and grant the series right of first publication with the work simultaneously licensed under a Creative Commons
Attribution License that allows others to share the work with an acknowledgment of the work's authorship and initial publication in this
series.
2. Authors are able to enter into separate, additional contractual arrangements for the non-exclusive distribution of the series's published
version of the work (e.g., post it to an institutional repository or publish it in a book), with an acknowledgment of its initial
publication in this series.
3. Authors are permitted and encouraged to post their work online (e.g., in institutional repositories or on their website) prior to and
during the submission process, as it can lead to productive exchanges, as well as earlier and greater citation of published work (See
Open access policy for details).