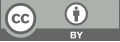
Forecasting urban unemployment rate in China using ARIMA model
- 1 Faculty of Arts and Science, University of Toronto St. George, Ontario, M5S 1A1, Canada
* Author to whom correspondence should be addressed.
Abstract
The urban unemployment rate is a significant economic indicator that has long drawn researchers’ interest. Monitoring and predicting changes in the unemployment rate can help in understanding economic trends and implementing appropriate measures. This article aims to forecast urban unemployment rates in China. By collecting previous surveyed urban unemployment rates in China, this article will generate and compare various ARIMA models in order to identify the one with the best forecasting accuracy. The forecast results of the selected model state that the unemployment rate will remain almost unchanged, around 5%, in the second half of 2024 and throughout 2025. Fluctuations are expected to be between 0.01% and 0.03%. The number is much lower than the peak during the pandemic, but it is still above the historical average. This article argues that China’s economy is gradually stabilizing, and the post-pandemic measures have been effective but are still insufficient. The government still needs to implement additional actions.
Keywords
Unemployment rate, forecast, ARIMA model.
[1]. Hjazeen H, Seraj M and Ozdeser H 2021 The nexus between the economic growth and unemployment in Jordan. Futur. Bus. J. 7 1-8
[2]. Soylu Ö B, Çakmak İ and Okur F 2018 Economic growth and unemployment issue: panel data analysis in Eastern European Countries. J. Int. Bus. Stud. 11 93-107
[3]. Didiharyono D and Syukri M 2020 Forecasting with ARIMA model in anticipating open unemployment rates in South Sulawesi. Int. J. Sci. Technol. Res. 9 3838-3841
[4]. Gostkowski M and Rokicki T 2021 Forecasting the unemployment rate: application of selected prediction methods. Eur. Res. Stud. 24 985-1000
[5]. Chakraborty T, Chakraborty A K, Biswas M, Banerjee S and Bhattacharya S 2021 Unemployment rate forecasting: a hybrid approach. Comput. Econ. 57 183-201
[6]. Ahmad M, Khan Y A, Jiang C, Kazmi S J H and Abbas S Z 2023 The impact of COVID-19 on unemployment rate: an intelligent based unemployment rate prediction in selected countries of Europe. Int J Fin Econ. 28 528-543
[7]. Będowska-Sójka B 2017 Unemployment rates forecasts: unobserved component models versus SARIMA models in Central and Eastern European countries. Comp. Econ. Res. 20 91-107
[8]. Dritsaki C 2016 Forecast of SARIMA models: an application to unemployment rates of Greece. Am. J. Appl. Math. Stat. 4 136-148
[9]. Dritsakis N and Klazoglou P 2018 Forecasting unemployment rates in USA using Box-Jenkins methodology. Int. J. Econ. Financ. Issues. 8 9
[10]. Katris C 2020 Prediction of unemployment rates with time series and machine learning techniques. Comput. Econ. 55 673-706
Cite this article
Jiang,X. (2024). Forecasting urban unemployment rate in China using ARIMA model. Theoretical and Natural Science,51,142-148.
Data availability
The datasets used and/or analyzed during the current study will be available from the authors upon reasonable request.
Disclaimer/Publisher's Note
The statements, opinions and data contained in all publications are solely those of the individual author(s) and contributor(s) and not of EWA Publishing and/or the editor(s). EWA Publishing and/or the editor(s) disclaim responsibility for any injury to people or property resulting from any ideas, methods, instructions or products referred to in the content.
About volume
Volume title: Proceedings of CONF-MPCS 2024 Workshop: Quantum Machine Learning: Bridging Quantum Physics and Computational Simulations
© 2024 by the author(s). Licensee EWA Publishing, Oxford, UK. This article is an open access article distributed under the terms and
conditions of the Creative Commons Attribution (CC BY) license. Authors who
publish this series agree to the following terms:
1. Authors retain copyright and grant the series right of first publication with the work simultaneously licensed under a Creative Commons
Attribution License that allows others to share the work with an acknowledgment of the work's authorship and initial publication in this
series.
2. Authors are able to enter into separate, additional contractual arrangements for the non-exclusive distribution of the series's published
version of the work (e.g., post it to an institutional repository or publish it in a book), with an acknowledgment of its initial
publication in this series.
3. Authors are permitted and encouraged to post their work online (e.g., in institutional repositories or on their website) prior to and
during the submission process, as it can lead to productive exchanges, as well as earlier and greater citation of published work (See
Open access policy for details).