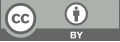
Prediction of atmospheric temperature in Sacramento area based on ARIMA and ETS models
- 1 National University of Singapore, 117546, Singapore
* Author to whom correspondence should be addressed.
Abstract
As global temperatures increased by 1.1 Celsius degrees, there has unprecedented shifts in climate systems. With the rising impact of global warming, exploring the warming trend helps to better understand and maintain the local environment and economy. This study focuses on predicting atmospheric temperature in the Sacramento area using ARIMA and ETS models. The research uses the temperature data from Sacramento Airport's Automated Surface Observing System (ASOS) and explores the prediction performance of ARIMA, ETS, and ARIMAX models in predicting daily average temperatures. The results indicate the ARIMAX (1,1,1) model is the most suitable for forecasting this temperature data with the lowest AIC and RMSE values. However, there are still challenges, in particular the dependence of the ARIMAX model on future exogenous variables, which leads to the forecast outcomes less accurate. To enable this ARIMAX models have better prediction performance, incorporating more exogenous variables is a potential solution. Therefore, the methods discussed in this paper provides ideas for the atmospheric temperature forecasting and points out the direction for further research.
Keywords
ARIMA, ARIMAX, ETS, atmospheric temperature, weather forecasting.
[1]. Calvin K, Dasgupta D, et al. 2023 Climate Change 2023: Synthesis Report. Contribution of Working Groups I, II and III to the Sixth Assessment Report of the Intergovernmental Panel on Climate Change. Intergovernmental Panel on Climate Change (IPCC).
[2]. Fernandez-Bou A S, Ortiz-Partida J P, Pells C, et al. 2021 California’s Fourth Climate Change Assessment: San Joaquin Valley region report. California Governors.
[3]. He M 2022 Assessing Changes in 21st Century Mean and Extreme Climate of the Sacramento–San Joaquin Delta in California. Climate, 10(2), 16.
[4]. De Giorgi M G, Ficarella A and Tarantino M 2011 Assessment of the benefits of numerical weather predictions in wind power forecasting based on statistical methods. Energy, 36(7), 3968-3978.
[5]. Yi J and Prybutok V R 1996 A neural network model forecasting for prediction of daily maximum ozone concentration in an industrialized urban area. Environmental Pollution, 92(3), 349-357.
[6]. Jiang D, Zhang Y, Hu X, Zeng Y, Tan J and Shao D 2004 Progress in developing an ANN model for air pollution index forecast. Atmospheric Environment, 38(40), 7055-7064.
[7]. Pal N R, Pal S, Das J and Majumdar K 2003 Sofm-mlp: a hybrid neural network for atmospheric temperature prediction. IEEE Transactions on Geoscience and Remote Sensing, 41(12), 278-2791.
[8]. Radhika Y and Shashi M 2009 Atmospheric Temperature Prediction using Support Vector Machines. International Journal of Computer Theory and Engineering, 55-58.
[9]. Park I, Kim H S, Lee J, Kim J H, Song C H and Kim H K 2019 Temperature Prediction Using the Missing Data Refinement Model Based on a Long Short-Term Memory Neural Network. Atmosphere, 10(11), 718.
[10]. Chu W T, Ho K C and Borji A 2018 Visual Weather Temperature Prediction. 2018 IEEE Winter Conference on Applications of Computer Vision (WACV).
Cite this article
Li,K. (2024). Prediction of atmospheric temperature in Sacramento area based on ARIMA and ETS models. Theoretical and Natural Science,51,165-171.
Data availability
The datasets used and/or analyzed during the current study will be available from the authors upon reasonable request.
Disclaimer/Publisher's Note
The statements, opinions and data contained in all publications are solely those of the individual author(s) and contributor(s) and not of EWA Publishing and/or the editor(s). EWA Publishing and/or the editor(s) disclaim responsibility for any injury to people or property resulting from any ideas, methods, instructions or products referred to in the content.
About volume
Volume title: Proceedings of CONF-MPCS 2024 Workshop: Quantum Machine Learning: Bridging Quantum Physics and Computational Simulations
© 2024 by the author(s). Licensee EWA Publishing, Oxford, UK. This article is an open access article distributed under the terms and
conditions of the Creative Commons Attribution (CC BY) license. Authors who
publish this series agree to the following terms:
1. Authors retain copyright and grant the series right of first publication with the work simultaneously licensed under a Creative Commons
Attribution License that allows others to share the work with an acknowledgment of the work's authorship and initial publication in this
series.
2. Authors are able to enter into separate, additional contractual arrangements for the non-exclusive distribution of the series's published
version of the work (e.g., post it to an institutional repository or publish it in a book), with an acknowledgment of its initial
publication in this series.
3. Authors are permitted and encouraged to post their work online (e.g., in institutional repositories or on their website) prior to and
during the submission process, as it can lead to productive exchanges, as well as earlier and greater citation of published work (See
Open access policy for details).