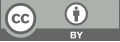
Forecasting of oil and gas stock market in major US
- 1 Faculty of Mathematical and Physical Sciences, University College London, Gower Street, London WC1E 6BT, United Kingdom
* Author to whom correspondence should be addressed.
Abstract
Oil and gas are two crucial resources in modern life and the development of technology. Investors are not only concerned about their own price, but their stock price is also an important part of formulating investment plans. This article will focus on the adjusted closing price since it consists of adjustments for splits, dividends, and capital gain distributions, which can demonstrate the situation of oil and gas in the stock market better. After the occurrence of COVID-19, the economic market of the whole world including the US. encountered great changes in many aspects. Thus, this article considered applying the model with the best performance from ARIMA, exponential smoothing, and Holt-Winters’ method to forecast the future adjusted closing prices of two large and representative companies in oil and gas from the US. According to the predicted results, the US oil and gas market is likely to experience a small but steady increase.
Keywords
Forecasting, ARIMA model, exponential smoothing, Holt-Winters’ method.
[1]. Basuony M A K, Bouaddi M, Ali H and EmadEldeen R 2021 The effect of COVID-19 pandemic on global stock markets: Return, volatility, and bad state probability dynamics. J Public Aff, 2761.
[2]. Wei J, Xu Q and He C 2022 Deep learning of predicting closing price through historical adjustment closing price. Procedia Computer Science, 202, 379-384.
[3]. Chouksey S 2019 Stock Price Prediction Using Time Series Models. MSc Data Analytics dissertation, Dublin Business School, 14.
[4]. Xue Q 2023 Stock Price Forecasting Based on ARIMA Model: An Example of Cheung Kong Hutchison Industrial Co. In: Highlights in Business, Economics and Management, 10, 425-430.
[5]. Vishwakarma A, Singh A, Mahadik A and Pradhan R 2020 Stock Price Prediction Using Sarima and Prophet Machine Learning Model. International Journal of Advanced Research in Science, Communication and Technology (IJARSCT), 9, 1-6.
[6]. Panda M 2020 Application of ARIMA and Holt-Winters forecasting model to predict the spreading of COVID-19 for India and its states. medRxiv.
[7]. Arum K C and Uche P I 2019 Volatility Modelling using Arch and Garch Models (A Case Study of the Nigerian Stock Exchange). International Journal of Mathematics Trends and Technology (IJMTT), 65, 4.
[8]. Almansour B, Alshater M M and Almansour A Y 2021 Performance of ARCH and GARCH Models in Forecasting Cryptocurrency Market Volatility. Industrial Engineering & Management Systems, 20, 130-139.
[9]. Vijh M, Chandola D, Tikkiwal VA and Kumar A 2020 Stock Closing Price Prediction using Machine Learning Techniques. Procedia Computer Science, 167, 599-606.
[10]. [10] Pitta de Jesus D, Souza Bezerra B F L and Da Nóbrega Besarria C 2020 The non-linear relationship between oil prices and stock prices: Evidence from oil-importing and oil-exporting countries. Research in International Business and Finance, 54, 101229.
Cite this article
Zuo,Z. (2024). Forecasting of oil and gas stock market in major US. Theoretical and Natural Science,42,36-45.
Data availability
The datasets used and/or analyzed during the current study will be available from the authors upon reasonable request.
Disclaimer/Publisher's Note
The statements, opinions and data contained in all publications are solely those of the individual author(s) and contributor(s) and not of EWA Publishing and/or the editor(s). EWA Publishing and/or the editor(s) disclaim responsibility for any injury to people or property resulting from any ideas, methods, instructions or products referred to in the content.
About volume
Volume title: Proceedings of the 2nd International Conference on Mathematical Physics and Computational Simulation
© 2024 by the author(s). Licensee EWA Publishing, Oxford, UK. This article is an open access article distributed under the terms and
conditions of the Creative Commons Attribution (CC BY) license. Authors who
publish this series agree to the following terms:
1. Authors retain copyright and grant the series right of first publication with the work simultaneously licensed under a Creative Commons
Attribution License that allows others to share the work with an acknowledgment of the work's authorship and initial publication in this
series.
2. Authors are able to enter into separate, additional contractual arrangements for the non-exclusive distribution of the series's published
version of the work (e.g., post it to an institutional repository or publish it in a book), with an acknowledgment of its initial
publication in this series.
3. Authors are permitted and encouraged to post their work online (e.g., in institutional repositories or on their website) prior to and
during the submission process, as it can lead to productive exchanges, as well as earlier and greater citation of published work (See
Open access policy for details).