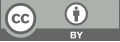
Physics Models and Machine Learning in Molecular Dynamics
- 1 Beijing 21st Century School
* Author to whom correspondence should be addressed.
Abstract
Tackling the complexity of particle interactions, this investigation introduces a unified computational methodology employing Moldy, Gnuplot, and Visual Molecular Dynamics (VMD). Inspired by the n-body problem's persistent intrigue, specifically the three-body dynamics within molecular systems, we leverage Molecular Dynamics (MD) simulations to forecast and scrutinize the nuanced behavior of atomic and molecular entities. Moldy, a flexible MD software, facilitated the simulation of particle trajectories and interactions across diverse scenarios, with meticulous documentation of the resulting data. For visual analytics and insight extraction, Gnuplot crafted detailed plots depicting kinetic and potential energies alongside other thermodynamic metrics over temporal scales. The integration of VMD culminated in our analysis by vividly portraying the molecular motions, enhancing comprehension of emergent patterns. This study not only endorses Moldy's precision in MD simulations but also highlights the potent alliance among simulation, analysis, and visualization in elucidating intricate particle interactions. In summary, our work underscores the efficacy of the Moldy-Gnuplot-VMD triad as a formidable resource for scientists engaged in molecular motion prediction and analysis, paving fresh paths in computational physics and chemistry research.
Keywords
physics models and machine learning, physics simulation, analysis of experiment graph in computer vision, basic molecular dynamics, three-body problem.
[1]. Alder, B. J., & Wainwright, T. E. (1959). Studies in Molecular Dynamics. I. General Method. The Journal of Chemical Physics, 31(2), 459–466. https://doi.org/10.1063/1.1730376
[2]. Schlick, T. (2013). Molecular Modeling and Simulation. Springer Science & Business Media.
[3]. Refson, K. (2000). Moldy: a portable molecular dynamics simulation program for serial and parallel computers. Computer Physics Communications, 126(3), 310–329. https://doi.org/10.1016/s0010-4655(99)00496-8
[4]. Racine, J. (2006). gnuplot 4.0: a portable interactive plotting utility. Journal of Applied Econometrics, 21(1), 133–141. https://doi.org/10.1002/jae.885
[5]. Humphrey, W., Dalke, A., & Schulten, K. (1996). VMD: Visual molecular dynamics. Journal of Molecular Graphics, 14(1), 33–38. https://doi.org/10.1016/0263-7855(96)00018-5
[6]. Valtonen, M. J., & Hannu Karttunen. (2006). The three-body problem. Cambridge University Press.
[7]. Trenti, M., & Hut, P. (2008). N-body simulations (gravitational). Scholarpedia, 3(5), 3930. https://doi.org/10.4249/scholarpedia.3930
[8]. Goldberger, A. L. (1996). Non-linear dynamics for clinicians: chaos theory, fractals, and complexity at the bedside. The Lancet, 347(9011), 1312–1314. https://doi.org/10.1016/s0140-6736(96)90948-4
[9]. Rössler, O. E. (1976). Chaotic Behavior in Simple Reaction Systems. Zeitschrift Für Naturforschung A, 31(3-4), 259–264. https://doi.org/10.1515/zna-1976-3-408
[10]. P.C. Hohenberg, & Shraiman, B. I. (1989). Chaotic behavior of an extended system. Physica D Nonlinear Phenomena, 37(1-3), 109–115. https://doi.org/10.1016/0167-2789(89)90121-8
[11]. Barrow-Green, J. (1997). Poincaré and the three body problem. American Mathematical Society ; London.
[12]. van Gunsteren, W. F., & Berendsen, H. J. C. (1977). Algorithms for macromolecular dynamics and constraint dynamics. Molecular Physics, 34(5), 1311–1327. https://doi.org/10.1080/00268977700102571
[13]. Watson, C. (2023, September 23). We Just Got 12,000 New Solutions to The Infamous Three-Body Problem. ScienceAlert.
[14]. Breen, P. G., Foley, C. N., Boekholt, T., & Zwart, S. P. (2020). Newton versus the machine: solving the chaotic three-body problem using deep neural networks. Monthly Notices of the Royal Astronomical Society, 494(2), 2465–2470. https://doi.org/10.1093/mnras/staa713
[15]. Mendoza, M. L. Sanchez., Aguilar, M. A., & del Valle, F. J. Olivares. (1998). A mean field approach that combines quantum mechanics and molecular dynamics simulation: the water molecule in liquid water. Journal of Molecular Structure: THEOCHEM, 426(1-3), 181–190. https://doi.org/10.1016/s0166-1280(97)00319-9
[16]. Kaminski, G. A., Friesner, R. A., Tirado-Rives, J., & Jorgensen, W. L. (2001). Evaluation and Reparametrization of the OPLS-AA Force Field for Proteins via Comparison with Accurate Quantum Chemical Calculations on Peptides. The Journal of Physical Chemistry B, 105(28), 6474–6487. https://doi.org/10.1021/jp003919d
[17]. Fujita, T., Nakano, T., & Tanaka, S. (2011). Fragment molecular orbital calculations under periodic boundary condition. Chemical Physics Letters, 506(1-3), 112–116. https://doi.org/10.1016/j.cplett.2011.02.055
Cite this article
Zhang,S. (2024). Physics Models and Machine Learning in Molecular Dynamics. Theoretical and Natural Science,55,34-40.
Data availability
The datasets used and/or analyzed during the current study will be available from the authors upon reasonable request.
Disclaimer/Publisher's Note
The statements, opinions and data contained in all publications are solely those of the individual author(s) and contributor(s) and not of EWA Publishing and/or the editor(s). EWA Publishing and/or the editor(s) disclaim responsibility for any injury to people or property resulting from any ideas, methods, instructions or products referred to in the content.
About volume
Volume title: Proceedings of the 2nd International Conference on Applied Physics and Mathematical Modeling
© 2024 by the author(s). Licensee EWA Publishing, Oxford, UK. This article is an open access article distributed under the terms and
conditions of the Creative Commons Attribution (CC BY) license. Authors who
publish this series agree to the following terms:
1. Authors retain copyright and grant the series right of first publication with the work simultaneously licensed under a Creative Commons
Attribution License that allows others to share the work with an acknowledgment of the work's authorship and initial publication in this
series.
2. Authors are able to enter into separate, additional contractual arrangements for the non-exclusive distribution of the series's published
version of the work (e.g., post it to an institutional repository or publish it in a book), with an acknowledgment of its initial
publication in this series.
3. Authors are permitted and encouraged to post their work online (e.g., in institutional repositories or on their website) prior to and
during the submission process, as it can lead to productive exchanges, as well as earlier and greater citation of published work (See
Open access policy for details).