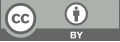
Research on Predicting Survival in Heart Failure Patients of Logistic Regression Models
- 1 School of Mathematics, Statistics and Mechanics, Beijing University of Technology, Beijing 100124, China
* Author to whom correspondence should be addressed.
Abstract
The purpose of this paper is to study a logistic regression model for predicting the survival of patients with heart failure. Heart failure is a serious clinical syndrome that usually develops from multiple heart diseases, and its inadequate pumping function of the heart poses a significant threat to the life and health of the patient and a significant economic burden on the healthcare system. Using medical record data from 299 patients with heart failure in the UCI database, this research utilized logistic regression models to identify the critical factors influencing the survival of heart failure patients and to forecast their survival outcomes. This paper found that age, ejection fraction, serum creatinine concentration and follow-up period are significant factors affecting survival in patients with heart failure. By adopting backward elimination method to optimize the model, the accuracy of prediction is further improved. The optimized logistic regression model yields an area under the Receiver Operating Characteristic (ROC) curve of 0.845, showing high prediction accuracy. The conclusions of this study provide a new perspective for the early diagnosis, risk assessment and personalized treatment of heart failure.
Keywords
Heart failure, survival prediction, logistic regression, backward elimination method.
[1]. Wang, Y., Wei, D., Cao, H., et al. (2024) A review of the application of deep learning in heart failure detection. Computer Science and Exploration, 1-17.
[2]. Zhao, Y. and Liu, H. (2019) Study on the status of symptom burden and its correlation with coping style in patients with chronic heart failure. Journal of Psychological Sciences, 19(15), 56-58+88.
[3]. Yang, X., Sun, J. and An, X. (2018) Efficacy of Daglipzin combined with sacubactril valsartan in the treatment of patients with chronic heart failure. Journal of Clinical Rational Use of Drugs, 17(24), 17-20.
[4]. Fan, M., Chang, L., Liang, L., et al. (2019) Retrospective study on the improvement of cardiac function in patients with viral pneumonia complicated with heart failure. Zhejiang Journal of Traditional Chinese Medicine, 59(08), 681-682.
[5]. Shi, C. and Hu, Z. (2024) A mutual information weighted K-nearest neighbor filling algorithm (MIW-KNN): Application of heart failure with Clostridium difficile infection in mortality prediction. Henan Science, 1-12.
[6]. Zhao Guozhong, et al. (2008) Logistic regression analysis of risk factors for heart failure in hemodialysis patients. Acta Academiae Medicinae Militaris Tertiae, 28(2), 131-144.
[7]. Chicco, D. and Jurman, G. (2020) Machine learning can predict survival of patients with heart failure from serum creatinine and ejection fraction alone. BMC Medical Informatics and Decision Making, 2020,20(1), 16.
[8]. Jiang, K., Li, L., Lai, Z., et al. (2019) Risk factors of heart failure in 80 patients with acute ST segment elevation myocardial infarction in Bazhong City. Chinese Journal of Emergency resuscitation and Disaster Medicine, 19(07), 855-857+866.
[9]. Margosian, S. et al. (2024) Challenges in Prognostication of an Older Adult with Severe Obesity and End-Stage Heart Failure: A Case Study. Journal of palliative medicine.
[10]. Sidey, G.J. and Sidey, G.C. (2019) Machine learning in medicine: a practical introduction. BMC Med Res Methodol, 19, 64.
Cite this article
Man,H. (2024). Research on Predicting Survival in Heart Failure Patients of Logistic Regression Models. Theoretical and Natural Science,53,255-261.
Data availability
The datasets used and/or analyzed during the current study will be available from the authors upon reasonable request.
Disclaimer/Publisher's Note
The statements, opinions and data contained in all publications are solely those of the individual author(s) and contributor(s) and not of EWA Publishing and/or the editor(s). EWA Publishing and/or the editor(s) disclaim responsibility for any injury to people or property resulting from any ideas, methods, instructions or products referred to in the content.
About volume
Volume title: Proceedings of the 2nd International Conference on Applied Physics and Mathematical Modeling
© 2024 by the author(s). Licensee EWA Publishing, Oxford, UK. This article is an open access article distributed under the terms and
conditions of the Creative Commons Attribution (CC BY) license. Authors who
publish this series agree to the following terms:
1. Authors retain copyright and grant the series right of first publication with the work simultaneously licensed under a Creative Commons
Attribution License that allows others to share the work with an acknowledgment of the work's authorship and initial publication in this
series.
2. Authors are able to enter into separate, additional contractual arrangements for the non-exclusive distribution of the series's published
version of the work (e.g., post it to an institutional repository or publish it in a book), with an acknowledgment of its initial
publication in this series.
3. Authors are permitted and encouraged to post their work online (e.g., in institutional repositories or on their website) prior to and
during the submission process, as it can lead to productive exchanges, as well as earlier and greater citation of published work (See
Open access policy for details).