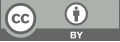
Comparative analysis of machine learning models for particle flow reconstruction
- 1 Ningcui Road and Sanxingzhuang Road Intersection, Haidian, Beijing, 100194, China
- 2 Dalton Academy, The Affiliated High School of Peking University, Beijing, 100086, China
- 3 Wuhan Britain-China, Wuhan, 430000, China
- 4 Jinan Foreign Language School, Jinan 250022, China
- 5 The affiliated Shenzhen School of Guangdong Experimental High School, Shenzhen, 518100, China
* Author to whom correspondence should be addressed.
Abstract
Using the simulation data of different calorimeters, we investigate the use of machine learning and python algorithms for the simulation and reconstruction of particle flow energy in high-energy physics. We train models based on simulated pixel value image instead of common numerical data. We define two models that use different Regressor algorithms. Multi-layer Perceptron (MLP) Regressor offer stable and accurate prediction under less affection situation. Convolutional Neutral Networks (CNN) Regressor provided better and stronger modeling and reconstruction ability; however, it could cause overfitting results in certain situations. Furthermore, we optimize our models with the use of PyTorch. These models can serve as fast method for particle flow reconstruction in future studies and experiments.
Keywords
Machine Learning, Particle Flow Reconstruction, Convolutional Neutral Networks (CNN), Multi-layer Perceptron(MLP).
[1]. F. Carminati et al., The European Physical Journal C 79, 946 (2019).
[2]. C. Jones and I. Kisel, Computing and Software for Big Science 5, 10 (2021).
[3]. T. Sj¨ostrand et al., Computational Physics Communica- tions 191, 159 (2015).
[4]. M. Brummer et al., Physical Review D 104, 054014 (2021).
[5]. G. Aad, X. S. Anduaga, S. Antonelli, M. Bendel, B. Breiler, F. Castrovillari, J. Civera, T. Del Prete, M. T. Dova, S. Duffin, et al., (2008).
[6]. J. Gao et al., The European Physical Journal C 77, 437 (2017).
[7]. J. Dong et al., The European Physical Journal C 82, 539 (2022).
[8]. L. Baulieu, A. Davydychev, R. Delbourgo, and A. Kiselev, Physics Letters B 529, 41 (2013).
[9]. A. Abada et al., The European Physical Journal Special Topics 228, 261 (2019).
[10]. M. Cepeda et al., CERN Yellow Reports: Monographs 7 (2020), 10.23731/CYRM-2020-007.
[11]. H. Li et al., Journal of Physics G: Nuclear and Particle Physics 45, 055002 (2018).
[12]. P. Allport et al., Annual Review of Nuclear and Particle Science 71, 497 (2021).
[13]. G. Aad et al., Journal of Instrumentation 3, S08003 (2014).
[14]. ATLAS Collaboration, Journal of Instrumentation 15, P09022 (2020).
[15]. S. Chatrchyan et al., Journal of Instrumentation 3, S08004 (2017).
[16]. S. Balaji et al., Journal of Instrumentation 15, P04026 (2020).
[17]. CMS Collaboration, Journal of Instrumentation 13, P05015 (2018).
[18]. A. M. Sirunyan et al., Journal of Instrumentation 16, P06016 (2021).
[19]. K. Anderson et al., Nuclear Instruments and Methods in Physics Research Section A 830, 223 (2016).
[20]. R. Tanaka et al., Journal of Instrumentation 13, P07006 (2018).
[21]. C. Patrignani et al., Chinese Physics C 40, 100001 (2016).
[22]. G. Louppe et al., Nature Communications 5, 3982 (2014).
[23]. B. Nachman et al., Annual Review of Nuclear and Par- ticle Science 69, 309 (2019).
[24]. A. Fantoni and O. behalf of the ALICE collaboration), in Journal of Physics: Conference Series, Vol. 293 (IOP Publishing, 2011) p. 012043.
[25]. L. E. Committee et al., Geneva, CERN , 254 (1994).
[26]. F. Hartmann, Nuclear Instruments and Methods in Physics Research Section A: Accelerators, Spectrometers, Detectors and Associated Equipment 666, 25 (2012), advanced Instrumentation.
[27]. X. Xu, S. L. Keoh, C. K. Seow, Q. Cao, and S. K. Bin Abdul Rahim, in 2023 8th International Conference on Business and Industrial Research (ICBIR) (2023) pp. 669–674.
[28]. T. scikit-learn developers, “MLPRegressor – multi-layer perceptron regressor, ” scikit-learn, the machine learning library for Python (2023), [Online; accessed: 2024-08-08].
[29]. Y. LeCun, Y. Bengio, and G. Hinton, nature 521, 436 (2015).
[30]. A. Krizhevsky, I. Sutskever, and G. E. Hinton, Advances in neural information processing systems 25 (2012).
[31]. F. J. Zhou ZH, Proceedings of the Twenty sixInternational Joint conference on Artificial Intelligence: 3553r3559 (2017).
[32]. D. P. Kingma and J. Ba, arXiv preprint arXiv:1412.6980 (2014).
[33]. C. M. Bishop and N. M. Nasrabadi, Pattern recognition and machine learning, Vol. 4 (Springer, 2006).
[34]. K. He, X. Zhang, S. Ren, and J. Sun, in Proceedings of the IEEE conference on computer vision and pattern recognition (2016) pp. 770–778.
Cite this article
Wang,S.;Yang,Z.;Li,Y.;Huo,X.;Yang,R. (2024). Comparative analysis of machine learning models for particle flow reconstruction. Theoretical and Natural Science,53,41-50.
Data availability
The datasets used and/or analyzed during the current study will be available from the authors upon reasonable request.
Disclaimer/Publisher's Note
The statements, opinions and data contained in all publications are solely those of the individual author(s) and contributor(s) and not of EWA Publishing and/or the editor(s). EWA Publishing and/or the editor(s) disclaim responsibility for any injury to people or property resulting from any ideas, methods, instructions or products referred to in the content.
About volume
Volume title: Proceedings of the 2nd International Conference on Applied Physics and Mathematical Modeling
© 2024 by the author(s). Licensee EWA Publishing, Oxford, UK. This article is an open access article distributed under the terms and
conditions of the Creative Commons Attribution (CC BY) license. Authors who
publish this series agree to the following terms:
1. Authors retain copyright and grant the series right of first publication with the work simultaneously licensed under a Creative Commons
Attribution License that allows others to share the work with an acknowledgment of the work's authorship and initial publication in this
series.
2. Authors are able to enter into separate, additional contractual arrangements for the non-exclusive distribution of the series's published
version of the work (e.g., post it to an institutional repository or publish it in a book), with an acknowledgment of its initial
publication in this series.
3. Authors are permitted and encouraged to post their work online (e.g., in institutional repositories or on their website) prior to and
during the submission process, as it can lead to productive exchanges, as well as earlier and greater citation of published work (See
Open access policy for details).