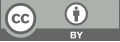
Simulation Assisted Improvement of Plastic Degradation Enzyme PETase based Machine Learning Tools
- 1 Shanghai American School Puxi Campus, Shanghai, China
* Author to whom correspondence should be addressed.
Abstract
Polyethylene terephthalate (PET) plastic is one of the most widely used plastic primarily due to its flexibility, endurance, and low cost. However, the plastic’s one-time use nature and long degradation time have led to massive waste accumulation, damaging our ecosystem, health, and biodiversity. While previous degradation methods are ineffective due to their high cost and low efficiency, the discovery of two enzymes PETase and MHETase in the bacteria Ideonella sakaiensis to degrade PET and mono(2-hydroxyethyl), a reaction intermediate in PET degradation, respectively, sparked the idea of a sustainable approach to degradation. Ever since, many approaches, including directed evolution, rational protein engineering, and computational redesign strategies, have optimized PETase in terms of its thermostability, catalytic activity, and more. This study proposes the incorporation of newly developed machine learning-based computational tools, including MutCompute, AlphaFold, and DiffDock, into a holistic protein engineering process to predict optimal PETase mutations. Here, in-silico experiments using machine learning tools as well as molecular dynamics simulation and interactions analysis screened for large amounts of PETase mutants in a time and cost-saving manner. Degradation assay coupled with mass analysis and high-performance liquid chromatography techniques then experimentally characterized PETase and its chosen mutants; thus, further screening found the most viable PETase mutant. Using various strategies, the project directly tackles one of the major global issues – sustainability – by bio-recycling PET. The research also aims to pave the way for introducing a new, imitable process for the more effective and resource-efficient engineering of all proteins.
Keywords
Machine learning, protein engineering, sustainability, PETase, plastic degradation
[1]. Joo, S., Cho, I. J., Seo, H., Son, H. F., Sagong, H.-Y., Shin, T. J., Choi, S. Y., Lee, S. Y., & Kim, K.-J. (2018). Structural insight into molecular mechanism of poly(ethylene terephthalate) degradation. Nature Communications, 9(1). https://doi.org/10.1038/s41467-018-02881-1
[2]. Yang, H., Chen, G., & Wang, J. (2021). Microplastics in the marine environment: Sources, Fates, impacts and microbial degradation. Toxics, 9(2), 41. https://doi.org/10.3390/toxics9020041
[3]. Han, X., Liu, W., Huang, J.-W., Ma, J., Zheng, Y., Ko, T.-P., Xu, L., Cheng, Y.-S., Chen, C.-C., & Guo, R.-T. (2017). Structural insight into catalytic mechanism of pet hydrolase. Nature Communications, 8(1). https://doi.org/10.1038/s41467-017-02255-z
[4]. Austin, H. P., Allen, M. D., Donohoe, B. S., Rorrer, N. A., Kearns, F. L., Silveira, R. L., Pollard, B. C., Dominick, G., Duman, R., El Omari, K., Mykhaylyk, V., Wagner, A., Michener, W. E., Amore, A., Skaf, M. S., Crowley, M. F., Thorne, A. W., Johnson, C. W., Woodcock, H. L., ... Beckham, G. T. (2018). Characterization and engineering of a plastic-degrading aromatic polyesterase. Proceedings of the National Academy of Sciences, 115(19). https://doi.org/10.1073/ pnas.1718804115
[5]. Jerves, C., Neves, R. P. P., Ramos, M. J., Silva, S. de, & Fernandes, P. A. (2021). Reaction mechanism of the PET degrading enzyme PETase studied with DFT/mm molecular dynamics simulations. ACS Catalysis, 11, 11626-11638. https://doi.org/10.1021/acscatal.1c03700.s002
[6]. Singh, A., Rorrer, N. A., Nicholson, S. R., Erickson, E., DesVeaux, J. S., Avelino, A. F. T., Lamers, P., Bhatt, A., Zhang, Y., Avery, G., Tao, L., Pickford, A. R., Carpenter, A. C., McGeehan, J. E., & Beckham, G. T. (2021). Techno-economic, life-cycle, and socioeconomic impact analysis of enzymatic recycling of poly(ethylene terephthalate). Joule, 5(9), 2479–2503. https://doi.org/10.1016/j.joule.2021.06.015
[7]. Yoshida, S., Hiraga, K., Takehana, T., Taniguchi, I., Yamaji, H., Maeda, Y., Toyohara, K., Miyamoto, K., Kimura, Y., & Oda, K. (2016). A bacterium that degrades and assimilates poly(ethylene terephthalate). Science, 351(6278), 1196–1199. https://doi.org/10.1126/ science.aad6359
[8]. Xu, Y., Cui, R., Han, Y., Jiang, J., Hu, D., Zhao, L., & Xi, Z. (2024). Efficient alcoholysis of poly(ethylene terephthalate) by using supercritical carbon dioxide as a green solvent. Polymers, 16(11), 1564. https://doi.org/10.3390/polym16111564
[9]. Bell, E. L., Smithson, R., Kilbride, S., Foster, J., Hardy, F. J., Ramachandran, S., Tedstone, A. A., Haigh, S. J., Garforth, A. A., Day, P. J., Levy, C., Shaver, M. P., & Green, A. P. (2022). Directed evolution of an efficient and thermostable PET depolymerase. Nature Catalysis, 5(8), 673–681. https://doi.org/10.1038/s41929-022-00821-3
[10]. Lu, H., Diaz, D. J., Czarnecki, N. J., Zhu, C., Kim, W., Shroff, R., Acosta, D. J., Alexander, B. R., Cole, H. O., Zhang, Y., Lynd, N. A., Ellington, A. D., & Alper, H. S. (2022). Machine learning- aided engineering of hydrolases for PET depolymerization. Nature, 604(7907), 662–667. https:// doi.org/10.1038/s41586-022-04599-z
[11]. Son, H. F., Cho, I. J., Joo, S., Seo, H., Sagong, H.-Y., Choi, S. Y., Lee, S. Y., & Kim, K.-J. (2019). Rational Protein Engineering of Thermo-Stable PETase from Ideonella sakaiensis for Highly Efficient PET Degradation. ACS Catalysis, 9(4), 3519–3526. https://doi.org/10.1021/acscatal.9b00568
[12]. Shroff, R., Cole, A. W., Diaz, D. J., Morrow, B. R., Donnell, I., Annapareddy, A., Gollihar, J., Ellington, A. D., & Thyer, R. (2020). Discovery of novel gain-of-function mutations guided by structure-based Deep Learning. ACS Synthetic Biology, 9(11), 2927–2935. https://doi.org/10.1021/acssynbio.0c00345
[13]. Jumper, J., Evans, R., Pritzel, A., Green, T., Figurnov, M., Ronneberger, O., Tunyasuvunakool, K., Bates, R., Žídek, A., Potapenko, A., Bridgland, A., Meyer, C., Kohl, S. A., Ballard, A. J., Cowie, A., Romera-Paredes, B., Nikolov, S., Jain, R., Adler, J., … Hassabis, D. (2021). Highly accurate protein structure prediction with AlphaFold. Nature, 596(7873), 583–589. https://doi.org/10.1038/s41586-021-03819-2
[14]. Forli, S., Huey, R., Pique, M. E., Sanner, M. F., Goodsell, D. S., & Olson, A. J. (2016). Computational protein–ligand docking and virtual drug screening with the autodock suite. Nature Protocols, 11(5), 905–919. https://doi.org/10.1038/nprot.2016.051
[15]. Corso, G., Stärk, H., Jing, B., Barzilay, R., & Jaakkola, T. (2022). DiffDock: Diffusion steps, twists, and turns for molecular docking. arXiv preprint arXiv:2210.01776.
[16]. Eastman, P., Swails, J., Chodera, J. D., McGibbon, R. T., Zhao, Y., Beauchamp, K. A., Wang, L.-P., Simmonett, A. C., Harrigan, M. P., Stern, C. D., Wiewiora, R. P., Brooks, B. R., & Pande, V. S. (2017). OpenMM 7: Rapid development of high performance algorithms for Molecular Dynamics. PLOS Computational Biology, 13(7). https://doi.org/10.1371/journal.pcbi.1005659
[17]. Meng, E. C., Goddard, T. D., Pettersen, E. F., Couch, G. S., Pearson, Z. J., Morris, J. H., & Ferrin, T. E. (2023). UCSF ChimeraX: Tools for structure building and analysis. Protein Science, 32(11). https://doi.org/10.1002/pro.4792
[18]. Bisong, E. (2019). Google Colaboratory. Building Machine Learning and Deep Learning Models on Google Cloud Platform, 59–64. https://doi.org/10.1007/978-1-4842-4470-8_7
[19]. Hanwell, M. D., Curtis, D. E., Lonie, D. C., Vandermeersch, T., Zurek, E., & Hutchison, G. R. (2012a). Avogadro: An advanced semantic chemical editor, visualization, and analysis Platform. Journal of Cheminformatics, 4(17). https://doi.org/10.1186/1758-2946-4-17
[20]. McNutt, A. T., Francoeur, P., Aggarwal, R., Masuda, T., Meli, R., Ragoza, M., Sunseri, J., & Koes, D. R. (2021). GNINA 1.0: Molecular docking with deep learning. Journal of Cheminformatics, 13(1). https://doi.org/10.1186/s13321-021-00522-2
[21]. Moruzzi, G. (2020). Plotting with matplotlib. Essential Python for the Physicist, 53–69. https://doi.org/10.1007/978-3-030-45027-4_3
[22]. Kufareva, I., & Abagyan, R. (2012). Methods of protein structure comparison. Methods in molecular biology, 857, 231–257. https://doi.org/10.1007/978-1-61779-588-6_10
[23]. Deng, J.-H., Luo, J., Mao, Y.-L., Lai, S., Gong, Y.-N., Zhong, D.-C., & Lu, T.-B. (2020). π-π stacking interactions: Non-negligible forces for stabilizing porous supramolecular frameworks. Science Advances, 6(2). https://doi.org/10.1126/sciadv.aax9976
[24]. Jia, Y., Samak, N. A., Hao, X., Chen, Z., Wen, Q., & Xing, J. (2022). Hydrophobic cell surface display system of PETase as a sustainable biocatalyst for PET degradation. Frontiers in Microbiology, 13, 1005480. https://doi.org/10.3389/fmicb.2022.1005480
[25]. Tournier, V., Topham, C. M., Gilles, A., David, B., Folgoas, C., Moya-Leclair, E., Kamionka, E., Desrousseaux, M.-L., Texier, H., Gavalda, S., Cot, M., Guémard, E., Dalibey, M., Nomme, J., Cioci, G., Barbe, S., Chateau, M., André, I., Duquesne, S., & Marty, A. (2020). An engineered PET depolymerase to break down and Recycle Plastic Bottles. Nature, 580(7802), 216–219. https:// doi.org/10.1038/s41586-020-2149-4
Cite this article
Tian,Z. (2024). Simulation Assisted Improvement of Plastic Degradation Enzyme PETase based Machine Learning Tools. Theoretical and Natural Science,67,181-194.
Data availability
The datasets used and/or analyzed during the current study will be available from the authors upon reasonable request.
Disclaimer/Publisher's Note
The statements, opinions and data contained in all publications are solely those of the individual author(s) and contributor(s) and not of EWA Publishing and/or the editor(s). EWA Publishing and/or the editor(s) disclaim responsibility for any injury to people or property resulting from any ideas, methods, instructions or products referred to in the content.
About volume
Volume title: Proceedings of the 4th International Conference on Biological Engineering and Medical Science
© 2024 by the author(s). Licensee EWA Publishing, Oxford, UK. This article is an open access article distributed under the terms and
conditions of the Creative Commons Attribution (CC BY) license. Authors who
publish this series agree to the following terms:
1. Authors retain copyright and grant the series right of first publication with the work simultaneously licensed under a Creative Commons
Attribution License that allows others to share the work with an acknowledgment of the work's authorship and initial publication in this
series.
2. Authors are able to enter into separate, additional contractual arrangements for the non-exclusive distribution of the series's published
version of the work (e.g., post it to an institutional repository or publish it in a book), with an acknowledgment of its initial
publication in this series.
3. Authors are permitted and encouraged to post their work online (e.g., in institutional repositories or on their website) prior to and
during the submission process, as it can lead to productive exchanges, as well as earlier and greater citation of published work (See
Open access policy for details).