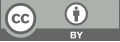
Identifying Novel Aging-Related Biomarkers for Age-related Macular Degeneration with Integrative Bioinformatics Approaches
- 1 Chongqing DEPU Foreign Language School, Chongqing, China
* Author to whom correspondence should be addressed.
Abstract
Age-related macular degeneration (AMD) is the leading cause of visual impairment in older adults worldwide and is a condition that causes visual deprivation. There exist two subcategories of this disease with the wet form of this disease being the focus of our study. Using bioinformatic analysis, this research conducted investigations to uncover the genes related to aging that may be biomarkers for the development of AMD. First, we compared the expression levels of samples from AMD/CNV patients and a control group using the GEO microarrays (GSE29801) in order to obtain differentially expressed genes (DEGs). WGCNA, combined with functional enrichment analysis, is utilized to discover and validate the gene module crucial for AMD. Differentially expressed aging-related genes (DEARGs) were identified by overlapping significant gene sets. The subcellular location of hub DEARGs and their corresponding cell subpopulations were determined and predicted using the Geo dataset GSE155288. Pan-cancer analyses were used to confirm those hub DEARGs’ function in other diseases. Moreover, both Protein-Protein Interaction (PPI) and AlphaFold prediction were employed to validate the protein interaction among the key DEARGs. Lastly, a potential target drug was selected, with portions of them validated through drug-protein interactions. In further analysis of our result, the collect gene set of 7 DEARGs was divided into the immune-related group and the non-immune-related group. These groups uncovered two distinct pathways of AMD development, with one triggering inflammatory responses by promoting macrophage proliferation and the other inducing choroidal neovascularization formation due to malfunctioning growth regulator genes
Keywords
Age-related macular degeneration (AMD), Age-related gene, transcriptomics
[1]. Fleckenstein, M., Keenan, T. D. L., Guymer, R. H., Chakravarthy, U., Schmitz-Valckenberg, S., Klaver, C. C., Wong, W. T. and Chew, E. Y. (2021) Age-related macular degeneration. Nature Reviews. Disease Primers, 7(1), 31.
[2]. Cheung, G. C. M., Lai, T. Y. Y., Gomi, F., Ruamviboonsuk, P., Koh, A. and Lee, W. K. (2017) Anti-VEGF Therapy for Neovascular AMD and Polypoidal Choroidal Vasculopathy. Asia-Pacific Journal of Ophthalmolog, 6(6), 527–534.
[3]. Hussain, R. M. and Ciulla, T. A. (2017) Emerging vascular endothelial growth factor antagonists to treat neovascular age-related macular degeneration. Expert Opinion on Emerging Drugs, 22(3), 235–246
[4]. Barrett, T., Wilhite, S. E., Ledoux, P., Evangelista, C., Kim, I. F., Tomashevsky, M., Marshall, K. A., Phillippy, K. H., Sherman, P. M., Holko, M., Yefanov, A., Lee, H., Zhang, N., Robertson, C. L., Serova, N., Davis, S. and Soboleva, A. (2013) NCBI GEO: archive for functional genomics data sets--update. Nucleic Acids Research, 41, D991–D995.
[5]. Langfelder, P. and Horvath, S. (2008). WGCNA: an R package for weighted correlation network analysis. BMC Bioinformatics, 9, 559.
[6]. Zhang, B. and Horvath, S. (2005) A general framework for weighted gene co-expression network analysis. Statistical Applications in Genetics and Molecular Biology, 4, Article17.
[7]. Thomas, P. D., Ebert, D., Muruganujan, A., Mushayahama, T., Albou, L. P. and Mi, H. (2022) PANTHER: Making genome-scale phylogenetics accessible to all. Protein Science : a Publication of the Protein Society, 31(1), 8–22.
[8]. Kanehisa, M. and Goto, S. (2000) KEGG: kyoto encyclopedia of genes and genomes. Nucleic Acids Research, 28(1), 27–30.
[9]. Liu, X., Jin, S., Hu, S., Li, R., Pan, H., Liu, Y., Lai, P., Xu, D., Sun, J., Liu, Z., Gao, Y., Zhao, Y., Liu, F., Xiao, Y., Li, Y., Wen, Y., Chen, Z., Xu, B., Lin, Y., Ran, M., Li, Q., Yang, S., Li, H., Tu, P., Haniffa, M., Teichmann, S., Bai, F. and Wang, Y. (2022) Single-cell transcriptomics links malignant T cells to the tumor immune landscape in cutaneous T cell lymphoma. Nature Communications, 13(1), 1158.
[10]. Miao, Y. R., Zhang, Q., Lei, Q., Luo, M., Xie, G. Y., Wang, H. and Guo, A. Y. (2020) ImmuCellAI: A Unique Method for Comprehensive T-Cell Subsets Abundance Prediction and its Application in Cancer Immunotherapy. Advanced Science, 7(7), 1902880.
[11]. Newman, A. M., Liu, C. L., Green, M. R., Gentles, A. J., Feng, W., Xu, Y., Hoang, C. D., Diehn, M. and Alizadeh, A. A. (2015) Robust enumeration of cell subsets from tissue expression profiles. Nature Methods, 12(5), 453–457.
[12]. Tang, Z., Kang, B., Li, C., Chen, T. and Zhang, Z. (2019) GEPIA2: an enhanced web server for large-scale expression profiling and interactive analysis. Nucleic Acids Research, 47(W1), W556–W560
[13]. UniProt Consortium (2019) UniProt: a worldwide hub of protein knowledge. Nucleic Acids Research, 47(D1), D506–D515. 14.
[14]. Yates, A. D., Achuthan, P., Akanni, W., Allen, J., Allen, J., Alvarez-Jarreta, J., Amode, M. R., Armean, I. M., Azov, A. G., Bennett, R., Bhai, J., Billis, K., Boddu, S., Marugán, J. C., Cummins, C., Davidson, C., Dodiya, K., Fatima, R., Gall, A., Giron, C. G., Gil, L., Grego, T., Haggerty, L., Haskell, E., Hourlier, T., Izuogu, O. G., Janacek, S. H., Juettemann, T., Kay, M., Lavidas, I., Le, T., Lemos, D., Gonzalez Martinez, J., Maurel, T., McDowall, M., McMahon, A., Mohanan, S., Moore, B., Nuhn, M., Oheh, D. N., Parker, A., Parton, A., Patricio, M., Sakthivel, M. P., Salam, A. I. A., Schmitt, B. M., Schuilenburg, H., Sheppard, D., Sycheva, M., Szuba, M., Taylor, K., Thormann, A., Threadgold, G., Vullo, A., Walts, B., Winterbottom, A., Zadissa, A., Chakiachvili, M., Flint, B., Frankish, A., Hunt, S. E., IIsley, G., Kostadima, M., Langridge, N., Loveland, J. E., Martin, F. J., Morales, J., Mudge, J. M., Muffato, M., Perry, E., Ruffier, M., Trevanion, S. J., Cunningham, F., Howe, K. L., Zerbino, D. R. and Flicek, P. (2020). Ensembl 2020. Nucleic acids research, 48(D1), D682–D688.
[15]. Abramson, J., Adler, J., Dunger, J., Evans, R., Green, T., Pritzel, A., Ronneberger, O., Willmore, L., Ballard, A. J., Bambrick, J., Bodenstein, S. W., Evans, D. A., Hung, C. C., O'Neill, M., Reiman, D., Tunyasuvunakool, K., Wu, Z., Žemgulytė, A., Arvaniti, E., Beattie, C., Bertolli, O., Bridgland, A., Cherepanov, A., Congreve, M., Cowen-Rivers, A., Cowie, A., Figurnov, M., Fuchs, F., Gladman, H., Jain, R., Khan, Y., Low, C., Perlin, K., Potapenko, A., Savy, P., Singh, S., Stecula, A., Thillaisundaram, A., Tong, C., Yakneen, S., Zhong, E., Zielinski, M., Žídek, A., Bapst, V., Kohli, P., Jaderberg, M., Hassabis, D. and Jumper, J. M. (2024) Accurate structure prediction of biomolecular interactions with AlphaFold 3. Nature, 630(8016), 493–500.
[16]. Kim, S., Chen, J., Cheng, T., Gindulyte, A., He, J., He, S., Li, Q., Shoemaker, B. A., Thiessen, P. A., Yu, B., Zaslavsky, L., Zhang, J. and Bolton, E. E. (2023) PubChem 2023 update. Nucleic Acids Research, 51(D1), D1373–D1380.
[17]. Morris, G. M., Huey, R., and Olson, A. J. (2008). Using AutoDock for ligand-receptor docking. Current protocols in bioinformatics, Chapter 8.
[18]. Raynard, C., Tessier, N., Huna, A., Warnier, M., Flaman, J. M., Van Coppenolle, F., Ducreux, S., Martin, N. and Bernard, D. (2022) Expression of the Calcium-Binding Protein CALB1 Is Induced and Controls Intracellular Ca2+ Levels in Senescent Cells. International Journal of Molecular Sciences, 23(16), 9376.
[19]. Ehrenberg, M., Pierce, E. A., Cox, G. F. and Fulton, A. B. (2013) CRB1: one gene, many phenotypes. Seminars in Ophthalmology, 28(5-6), 397–405.
[20]. Ehrenberg, M., Pierce, E. A., Cox, G. F. and Fulton, A. B. (2013) CRB1: one gene, many phenotypes. Seminars in Ophthalmology, 28(5-6), 397–405.
[21]. Peng, S., Li, J. J., Song, W., Li, Y., Zeng, L., Liang, Q., Wen, X., Shang, H., Liu, K., Peng, P., Xue, W., Zou, B., Yang, L., Liang, J., Zhang, Z., Guo, S., Chen, T., Li, W., Jin, M., Xing, X. B., Wan, P., Liu, C., Lin, H., Wei, H., Lee, R. W. J., Zhang, Feng. and Wei, L. (2024). CRB1-associated retinal degeneration is dependent on bacterial translocation from the gut. Cell, 187(6), 1387–1401.e13.
[22]. Richter, L., Flodman, P., Barria von-Bischhoffshausen, F., Burch, D., Brown, S., Nguyen, L., Turner, J., Spence, M. A. and Bateman, J. B. (2008) Clinical variability of autosomal dominant cataract, microcornea and corneal opacity and novel mutation in the alpha A crystallin gene (CRYAA). American Journal of Medical Genetics. Part A, 146A(7), 833–842.
[23]. Zhang, S., Liu, C., Wang, Q., Zhou, H., Wu, H., Zhuang, J., Cao, Y., Shi, H., Zhang, J. and Wang, J. (2023) CRYAA and GJA8 promote visual development after whisker tactile deprivation. Heliyon, 9(3), e13897.
[24]. Billesbølle, C. B., Azumaya, C. M., Kretsch, R. C., Powers, A. S., Gonen, S., Schneider, S., Arvedson, T., Dror, R. O., Cheng, Y. and Manglik, A. (2020) Structure of hepcidin-bound ferroportin reveals iron homeostatic mechanisms. Nature, 586(7831), 807–811.
[25]. Maus, M., López-Polo, V., Mateo, L., Lafarga, M., Aguilera, M., De Lama, E., Meyer, K., Sola, A., Lopez-Martinez, C., López-Alonso, I., Guasch-Piqueras, M., Hernandez-Gonzalez, F., Chaib, S., Rovira, M., Sanchez, M., Faner, R., Agusti, A., Diéguez-Hurtado, R., Ortega, S., Manonelles, A., Engelhardt, S., Monteiro, F., Attolini, C., Prats, N., Albaiceta, G., Cruzado, J. and Serrano, M. (2023) Iron accumulation drives fibrosis, senescence and the senescence-associated secretory phenotype. Nature Metabolism, 5(12), 2111–2130.
[26]. Ganz, T. and Nemeth, E. (2012) Hepcidin and iron homeostasis. Biochimica et Biophysica Acta, 1823(9), 1434– 1443.
[27]. Halder, G., Callaerts, P. and Gehring, W. J. (1995) Induction of ectopic eyes by targeted expression of the eyeless gene in Drosophila. Science, 267(5205), 1788–1792.
[28]. Lima Cunha, D., Arno, G., Corton, M. and Moosajee, M. (2019) The Spectrum of PAX6 Mutations and GenotypePhenotype Correlations in the Eye. Genes, 10(12), 1050.
[29]. Zhang, H., Wang, Y., Liu, C., Li, W., Zhou, F., Wang, X. and Zheng, J. (2022) The Apolipoprotein C1 is involved in breast cancer progression via EMT and MAPK/JNK pathway. Pathology, Research and Practice, 229, 153746.
[30]. Ren, L., Yi, J., Yang, Y., Li, W., Zheng, X., Liu, J., Li, S., Yang, H., Zhang, Y., Ge, B., Zhang, S., Fu, W., Dong, D., Du, G., Wang, X. and Wang, J. (2022) Systematic pan-cancer analysis identifies APOC1 as an immunological biomarker which regulates macrophage polarization and promotes tumor metastasis. Pharmacological Research, 183, 106376.
[31]. Khalil, H., Tazi, M., Caution, K., Ahmed, A., Kanneganti, A., Assani, K., Kopp, B., Marsh, C., Dakhlallah, D. and Amer, A. O. (2016) Aging is associated with hypermethylation of autophagy genes in macrophages. Epigenetics, 11(5), 381–388. Wong, C. K., Smith, C. A., Sakamoto, K., Kaminski, N., Koff, J. L. and Goldstein, D. R. (2017). Aging Impairs Alveolar Macrophage Phagocytosis and Increases Influenza-Induced Mortality in Mice. Journal of immunology, 199(3), 1060–1068.
[32]. Wong, C. K., Smith, C. A., Sakamoto, K., Kaminski, N., Koff, J. L. and Goldstein, D. R. (2017). Aging Impairs Alveolar Macrophage Phagocytosis and Increases Influenza-Induced Mortality in Mice. Journal of immunology, 199(3), 1060–1068.
[33]. Hagbi-Levi, S., Grunin, M., Jaouni, T., Tiosano, L., Rinsky, B., Elbaz-Hayoun, S., Peled, A. and Chowers, I. (2017) Proangiogenic characteristics of activated macrophages from patients with age-related macular degeneration. Neurobiology of Aging, 51, 71–82.
[34]. Nakamura, R., Sene, A., Santeford, A., Gdoura, A., Kubota, S., Zapata, N. and Apte, R. S. (2015) IL10-driven STAT3 signalling in senescent macrophages promotes pathological eye angiogenesis. Nature Communications, 6, 7847.
[35]. Imai, T., Hieshima, K., Haskell, C., Baba, M., Nagira, M., Nishimura, M., Kakizaki, M., Takagi, S., Nomiyama, H., Schall, T. J. and Yoshie, O. (1997) Identification and molecular characterization of fractalkine receptor CX3CR1, which mediates both leukocyte migration and adhesion. Cell, 91(4), 521–530.
[36]. Beli, E., Dominguez, J. M., 2nd, Hu, P., Thinschmidt, J. S., Caballero, S., Li Calzi, S., Luo, D., Shanmugam, S., Salazar, T. E., Duan, Y., Boulton, M. E., Mohr, S., Abcouwer, S. F., Saban, D. R., Harrison, J. K. and Grant, M. B. (2016) CX3CR1 deficiency accelerates the development of retinopathy in a rodent model of type 1 diabetes. Journal of Molecular Medicine, 94(11), 1255–1265.
[37]. Landsman, L., Bar-On, L., Zernecke, A., Kim, K. W., Krauthgamer, R., Shagdarsuren, E., Lira, S. A., Weissman, I. L., Weber, C. and Jung, S. (2009) CX3CR1 is required for monocyte homeostasis and atherogenesis by promoting cell survival. Blood, 113(4), 963–972.
[38]. Stevens, T. S., Bressler, N. M., Maguire, M. G., Bressler, S. B., Fine, S. L., Alexander, J., Phillips, D. A., Margherio, R. R., Murphy, P. L. and Schachat, A. P. (1997) Occult choroidal neovascularization in age-related macular degeneration. A natural history study. Archives of Ophthalmology, 115(3), 345–350.
[39]. Little, K., Ma, J. H., Yang, N., Chen, M. and Xu, H. (2018) Myofibroblasts in macular fibrosis secondary to neovascular age-related macular degeneration - the potential sources and molecular cues for their recruitment and activation. EBioMedicine, 38, 283–291.
[40]. Little, K., Llorián-Salvador, M., Tang, M., Du, X., Marry, S., Chen, M. and Xu, H. (2020) Macrophage to myofibroblast transition contributes to subretinal fibrosis secondary to neovascular age-related macular degeneration. Journal of Neuroinflammation, 17(1), 355.
Cite this article
Zhao,L. (2025). Identifying Novel Aging-Related Biomarkers for Age-related Macular Degeneration with Integrative Bioinformatics Approaches. Theoretical and Natural Science,90,1-22.
Data availability
The datasets used and/or analyzed during the current study will be available from the authors upon reasonable request.
Disclaimer/Publisher's Note
The statements, opinions and data contained in all publications are solely those of the individual author(s) and contributor(s) and not of EWA Publishing and/or the editor(s). EWA Publishing and/or the editor(s) disclaim responsibility for any injury to people or property resulting from any ideas, methods, instructions or products referred to in the content.
About volume
Volume title: Proceedings of ICMMGH 2025 Workshop: Computational Modelling in Biology and Medicine
© 2024 by the author(s). Licensee EWA Publishing, Oxford, UK. This article is an open access article distributed under the terms and
conditions of the Creative Commons Attribution (CC BY) license. Authors who
publish this series agree to the following terms:
1. Authors retain copyright and grant the series right of first publication with the work simultaneously licensed under a Creative Commons
Attribution License that allows others to share the work with an acknowledgment of the work's authorship and initial publication in this
series.
2. Authors are able to enter into separate, additional contractual arrangements for the non-exclusive distribution of the series's published
version of the work (e.g., post it to an institutional repository or publish it in a book), with an acknowledgment of its initial
publication in this series.
3. Authors are permitted and encouraged to post their work online (e.g., in institutional repositories or on their website) prior to and
during the submission process, as it can lead to productive exchanges, as well as earlier and greater citation of published work (See
Open access policy for details).