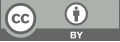
Survival Prediction of Agricultural Trees by Optimizing Long and Short Term Memory Networks Based on Multi-head Attention Mechanism
- 1 Economics and Management, Yunnan Agricultural University, Kunming, Yunnan, 650051, China
- 2 Economics and Management, Yunnan Agricultural University, Kunming, Yunnan, 650051, China
- 3 Economics and Management, Yunnan Agricultural University, Kunming, Yunnan, 650051, China
- 4 Economics and Management, Yunnan Agricultural University, Kunming, Yunnan, 650051, China
- 5 Economics and Management, Yunnan Agricultural University, Kunming, Yunnan, 650051, China
* Author to whom correspondence should be addressed.
Abstract
In this study, long short-term memory network (LSTM) model was optimized based on multi-head attention mechanism to effectively predict the survival of agricultural trees. Based on the in-depth analysis of a large number of agricultural tree survival data, the corresponding prediction model was constructed. In the training phase, we analyzed the confusion matrix of the training set, and the results revealed that the accuracy of the model in predicting the survival of agricultural trees was as high as 99.74%. In addition, the performance on the independent test set is also very good, with an accuracy of 99.40%, although there is a slight decrease compared to the training set (0.34%), but both maintain a high accuracy level of more than 99%. This shows that the model has very high prediction accuracy and maintains good generalization ability across different data sets. Further, by drawing the ROC curve and calculating the AUC (area under the curve), the result is 0.9857, which fully reflects the strong ability of the model in the tree survival prediction task. An AUC value higher than 0.9 indicates that the model has a very low error rate on the classification task, which ensures the reliability of the prediction results. This study shows that the LSTM optimization model based on multi-head attention mechanism can provide a high-precision tree survival prediction tool for agricultural management, so as to help farmers make more effective decisions. This result not only has theoretical value, but also has important significance for the growth management and sustainable development of trees in practical application. Through this research, we expect to be able to contribute to the development of smart agriculture.
Keywords
Multi-head attention mechanism, Long and short term memory network, Agricultural tree survival prediction
[1]. Zhou, Z., et al. "Improving the classification accuracy of fishes and invertebrates using residual convolutional neural networks." ICES Journal of Marine Science 80.5 (2023): 1256-1266.
[2]. Nandika, Muhammad Rizki, et al. "Assessing the Shallow Water Habitat Map** Extracted from High-Resolution Satellite Image with Multi Classification Algorithms." Geomatics and Environmental Engineering 17.2 (2023).
[3]. Lopez-Vazquez, Vanesa, et al. "Deep learning based deep-sea automatic image enhancement and animal species classification." Journal of Big Data 10.1 (2023): 37.
[4]. Ahmad, Usman, et al. "Large Scale Fish Images Classification and Localization using Transfer Learning and Localization Aware CNN Architecture." Comput. Syst. Sci. Eng. 45.2 (2023): 2125-2140.
[5]. Fan, Jianchao, and Chuan Liu. "Multitask gans for oil spill classification and semantic segmentation based on sar images." IEEE Journal of Selected Topics in Applied Earth Observations and Remote Sensing 16 (2023): 2532-2546.
[6]. Aziz, Rabia Musheer, et al. "CO‐WOA: novel optimization approach for deep learning classification of fish image." Chemistry & Biodiversity 20.8 (2023): e202201123.
[7]. Danqing Ma, , Shaojie Li, Bo Dang, Hengyi Zang, Xinqi Dong. "Fostc3net:A Lightweight YOLOv5 Based On the Network Structure Optimization." (2024).
[8]. Zongqing Qi, , Danqing Ma, Jingyu Xu, Ao Xiang, Hedi Qu. "Improved YOLOv5 Based on Attention Mechanism and FasterNet for Foreign ObjectDetection on Railway and Airway tracks." (2024).
[9]. Lu, Liangdong, Jia Xu, and Jiuchang Wei. "Understanding the effects of the textual complexity on government communication: Insights from China’s online public service platform. "Telematics and Informatics 83 (2023): 102028.
[10]. Kumari, Rina, et al. "Identifying multimodal misinformation leveraging novelty detection and emotion recognition." Journal of Intelligent Information Systems 61.3 (2023): 673-694.
Cite this article
Dai,Y.;Zhou,J.;Bai,X.;Hua,J.;Sun,H. (2025). Survival Prediction of Agricultural Trees by Optimizing Long and Short Term Memory Networks Based on Multi-head Attention Mechanism. Theoretical and Natural Science,94,31-37.
Data availability
The datasets used and/or analyzed during the current study will be available from the authors upon reasonable request.
Disclaimer/Publisher's Note
The statements, opinions and data contained in all publications are solely those of the individual author(s) and contributor(s) and not of EWA Publishing and/or the editor(s). EWA Publishing and/or the editor(s) disclaim responsibility for any injury to people or property resulting from any ideas, methods, instructions or products referred to in the content.
About volume
Volume title: Proceedings of the 3rd International Conference on Environmental Geoscience and Earth Ecology
© 2024 by the author(s). Licensee EWA Publishing, Oxford, UK. This article is an open access article distributed under the terms and
conditions of the Creative Commons Attribution (CC BY) license. Authors who
publish this series agree to the following terms:
1. Authors retain copyright and grant the series right of first publication with the work simultaneously licensed under a Creative Commons
Attribution License that allows others to share the work with an acknowledgment of the work's authorship and initial publication in this
series.
2. Authors are able to enter into separate, additional contractual arrangements for the non-exclusive distribution of the series's published
version of the work (e.g., post it to an institutional repository or publish it in a book), with an acknowledgment of its initial
publication in this series.
3. Authors are permitted and encouraged to post their work online (e.g., in institutional repositories or on their website) prior to and
during the submission process, as it can lead to productive exchanges, as well as earlier and greater citation of published work (See
Open access policy for details).