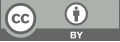
Enhancing cloth-changing person re-identification with Silhouette-Keypoint fusion
- 1 School of Computer Science and Communication Engineering, JiangSu University, Jiangsu, China
* Author to whom correspondence should be addressed.
Abstract
In recent years, person re-identification (ReID) has experienced significant advancements due to its diverse real-world applications. However, traditional benchmarks often assume consistent attire across captured images, failing to reflect the reality of pedestrians frequently changing their clothing. This discrepancy has led to the emergence of the cloth-changing person re-identification (CC-ReID) problem and the development of relevant benchmarks. CC-ReID poses a substantial challenge, as pedestrians’ primary visual cues, particularly their clothing, vary between query and gallery images, while non-attire-related features remain relatively weak. To address this gap and advance research in CC-ReID, this paper introduces a novel task termed Silhouette-Keypoint Fusion Re-Identification (SKF-ReID). This represents a dual-stream framework capable of extracting silhouette and keypoint details within the shape stream, subsequently transferring this data to the ReID stream to enrich appearance features with clothing-independent insights. Additionally, we employ the Maximum Mean Discrepancy (MMD) loss to ensure similarity between shape and appearance features, thereby enhancing the accuracy of cloth-changing person re-identification. Our proposed approach undergoes rigorous evaluation across benchmark cloth-changing person re-identification datasets, showcasing cutting-edge performance.
Keywords
Cloth-changing Person ReID, Clothing Attention Degradation, Human Semantic Attention
[1]. Zhao, H., Tian, M., Sun, S. Shao, J., & Tang, X. (2017). Spindle Net: Person Re-identification with Human Body Region Guided Feature Decomposition and Fusion. In 2017 IEEE Conference on Computer Vision and Pattern Recognition (CVPR) (pp. 4929-4938). IEEE.
[2]. Jin, X., Lan, C., Zeng, W., Wei, G., & Chen, Z. (2019). Semantics-Aligned Representation Learning for Person Re-identification. arXiv preprint arXiv:1905.13143. DOI: 10.48550/arXiv.1905.13143.
[3]. He, K., Zhang, X., Ren, S., & Sun, J. (2016). Deep residual learning for image recognition, in CVPR, 2016, pp. 2, 4, 5.
[4]. Chen, L. C., Papandreou, G., Schroff, F., & Adam, H. . (2017). Rethinking Atrous Convolution for Semantic Image Segmentation. (2017). DOI: 10.48550/arXiv.1706.05587.
[5]. Cao, Z., Hidalgo, G., Simon, T., Wei, S. E., & Sheikh, Y. (2018). Openpose: Realtime multi-person 2D pose estimation using part affinity fields. IEEE Transactions on Pattern Analysis and Machine Intelligence, 40(12), 2893-2906.
[6]. Q. Yang, A. Wu, W.-S. Zheng, (2019). Person reidentification by contour sketch under moderate clothing change, in TPAMI, 2019, pp. 1, 2, 5, 8.
[7]. X. Gu, H. Chang, B. Ma, S. Bai, S. Shan, & X. Chen. (2022). Clothes-changing person re-identification with RGB modality only, in CVPR, 2022, pp. 1, 3, 6, 7.
[8]. Jin, X., He, T., Zheng, K., Yin, Z., Shen, X., Huang, Z., Feng, R., Huang, J., Hua, X., & Chen, Z. (2021). Cloth-Changing Person Re-identification from A Single Image with Gait Prediction and Regularization. arXiv preprint arXiv:2103.15537. DOI: 10.48550/arXiv.2103.15537.
[9]. Gao, Z., Wei, S., Guan, W., Zhu, L., Wang, M., & Chen, S. (2023). Identity-Guided Collaborative Learning for Cloth-Changing Person Reidentification. arXiv preprint arXiv:2304.04400.
[10]. Yang, Z., Lin, M., Zhong, X., Wu, Y., & Wang, Z. (2023). Good is Bad: Causality Inspired Cloth-debiasing for Cloth-changing Person Re-identification. Proceedings of the Conference on Computer Vision and Pattern Recognition (CVPR), 2023.
[11]. Li, S., Chen, H., Yu, S. He, Z., Zhu, F., Zhao, R., Chen, J., & Qiao, Y. (2017). COCAS+: Large-Scale Clothes-Changing Person Re-Identification With Clothes Templates. In IEEE, 2023. 4
[12]. Li, S., Chen, H., Yu, S. He, Z., Zhu, F., Zhao, R., Chen, J., & Qiao, Y. (2017). COCAS+: Large-Scale Clothes-Changing Person Re-Identification With Clothes Templates. In IEEE, 2023. 4
[13]. Miao, J., Wu, Y., Liu, P., Ding, Y., & Yang, Y. . (2019). Pose-Guided Feature Alignment for Occluded Person Re-Identification. In 2019 IEEE/CVF International Conference on Computer Vision (ICCV) (pp. 3996-4005). IEEE.
[14]. Huang. Y, Wu. Q, Xu. J, Zhong. Y, & Zhang. Z. (2021). Clothing status awareness for long-term person re-identification, in ICCV, 2021, pp. 1, 3, 7.
[15]. Ioffe, S., Szegedy, C. (2015). Batch Normalization: Accelerating Deep Network Training by Reducing Internal Covariate Shift. Journal of Machine Learning Research, 2015. doi: 10.48550/arXiv.1502.03167.
[16]. Qian, X. , Wang, W. , Zhang, L. , Zhu, F. , & Xue, X. (2021). Long-term cloth-changing person re-identification, in ACCV, 2020, pp. 1-3, 6, 7.
[17]. Kingma, D., Ba, J. (2014). Adam: A Method for Stochastic Optimization. Computer Science, 2014. doi: 10.48550/arXiv.1412.6980.
[18]. G. Wang, Y. Yuan, X. Chen, J. Li, & X. Zhou. (2018) Learning discriminative features with multiple granularities for person re-identification, in Proceedings of the 26th ACM international conference on Multimedia, 2018, pp. 274-282.
[19]. Sun, Y., Zheng, L., Yang, Y., Tian, Q., & Wang, S. (2017). Beyond Part Models: Person Retrieval with Refined Part Pooling (and A Strong Convolutional Baseline). In European Conference on Computer Vision (pp. 418-434). Springer, Cham.
Cite this article
Li,X. (2025). Enhancing cloth-changing person re-identification with Silhouette-Keypoint fusion. Theoretical and Natural Science,95,63-69.
Data availability
The datasets used and/or analyzed during the current study will be available from the authors upon reasonable request.
Disclaimer/Publisher's Note
The statements, opinions and data contained in all publications are solely those of the individual author(s) and contributor(s) and not of EWA Publishing and/or the editor(s). EWA Publishing and/or the editor(s) disclaim responsibility for any injury to people or property resulting from any ideas, methods, instructions or products referred to in the content.
About volume
Volume title: Proceedings of the 2nd International Conference on Applied Physics and Mathematical Modeling
© 2024 by the author(s). Licensee EWA Publishing, Oxford, UK. This article is an open access article distributed under the terms and
conditions of the Creative Commons Attribution (CC BY) license. Authors who
publish this series agree to the following terms:
1. Authors retain copyright and grant the series right of first publication with the work simultaneously licensed under a Creative Commons
Attribution License that allows others to share the work with an acknowledgment of the work's authorship and initial publication in this
series.
2. Authors are able to enter into separate, additional contractual arrangements for the non-exclusive distribution of the series's published
version of the work (e.g., post it to an institutional repository or publish it in a book), with an acknowledgment of its initial
publication in this series.
3. Authors are permitted and encouraged to post their work online (e.g., in institutional repositories or on their website) prior to and
during the submission process, as it can lead to productive exchanges, as well as earlier and greater citation of published work (See
Open access policy for details).