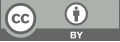
Investment Portfolio and Optimization Based on the Recent Popular Stocks in the US Stock Market
- 1 School of Mathematics and Statistics, Central South University, Changsha, China
* Author to whom correspondence should be addressed.
Abstract
The US stock market remains a focal point for both institutional and individual investors, with portfolio optimization being a critical need. Traditional approaches to asset allocation often rely on single models or incremental improvements, which struggle to address the complexity and instability of financial markets. Hence, this study adopts a model-based analytical framework to address these limitations. First, theoretical models are collected and derived to establish the research foundation. Second, the financial market is analyzed using quantitative data from the Yahoo Finance database. Third, econometric models are constructed, parameters are estimated, and models are optimized. Fourth, data visualization techniques are employed to generate analytical charts. Finally, model results are evaluated and compared to provide investment strategy recommendations. Advanced models, including the mean-variance model, Black-Litterman model, and genetic algorithm, are introduced to enhance adaptability to market complexity. The mean-variance model provides intuitive risk-return insights but relies heavily on historical data. The Black-Litterman model incorporates investor views but overlooks asset-specific risks. The genetic algorithm offers flexibility in handling constraints but requires significant computational resources. While these models provide valuable insights, investors should integrate their own market understanding to optimize portfolio decisions. This study highlights the importance of combining model-based analysis with investor expertise to navigate financial market uncertainties effectively.
Keywords
American stock market, Portfolio and optimization, Mean-variance model, Black-litterman model, Genetic algorithm
[1]. Fan, Z. J. (2024). U.S. stocks hit record highs. China Business News, A05, 1-2.
[2]. Qi, X. X. (2024). The way of money management: Steady strategy and risk control. China Venture Capital, No. 23, 32-34.
[3]. Gu, H. Y. (2020). Analysis of the influence of individual investor's investment psychology factors on investment behavior. Trade Fair Economy, No. 11, 62-64.
[4]. Lewis, D. (2024). Do these 2 stocks belong in the Magnificent 7? Nasdaq. https://www.nasdaq.com/articles/do-these-2-stocks-belong-in-the-magnificent-7
[5]. Mohanty, S. (2024). MSFT vs. GOOGL: Which 'Magnificent Seven' stock is the better buy? Nasdaq. https://www.nasdaq.com/articles/msft-vs-googl-which-magnificent-seven-stock-better-buy
[6]. Markowitz, H. (1952). Portfolio selection. Journal of Finance, 7(1), 77-91.
[7]. Markowitz, H. (1965). The optimization of a quadratic function subject to linear constraints. Naval Research Logistics Quarterly, 3, 111-133.
[8]. Dang, S. L., Zhang, P., & Li, J. X. (2023). Admissible mean-variance portfolio optimization with cardinality constraints. Fuzzy Systems and Mathematics, 37(4), 92-103.
[9]. Brauneis, A., & Mestel, R. (2019). Cryptocurrency portfolios in a mean-variance framework. Finance Research Letters, 28, 259-264.
[10]. Michaud, R. O. (1989). The Markowitz optimization enigma: Is ‘optimized’ optimal? Financial Analysts Journal, 45(1), 31-42.
[11]. Black, F., & Litterman, R. (1992). Global portfolio optimization. Financial Analysts Journal, 48(5), 28-43.
[12]. Kolm, P., & Ritter, G. (2017). On the Bayesian interpretation of Black–Litterman. European Journal of Operational Research, 258(2), 564-572.
[13]. Song, Z. Y., Zhou, Z. B., Yu, L. A., & Ren, T. T. (2023). Research on portfolio optimization strategy based on hybrid integrated forecasting algorithm and Black-Litterman model. Chinese Journal of Management Science. https://doi.org/10.16381/j.cnki.issn1003-207x.2023.1895
[14]. Xu, B. X. (2024). Portfolio optimization based on genetic algorithm and CVaR. Economic Research Guide, No. 13, 71-74.
Cite this article
Yu,Q. (2025). Investment Portfolio and Optimization Based on the Recent Popular Stocks in the US Stock Market. Theoretical and Natural Science,101,52-59.
Data availability
The datasets used and/or analyzed during the current study will be available from the authors upon reasonable request.
Disclaimer/Publisher's Note
The statements, opinions and data contained in all publications are solely those of the individual author(s) and contributor(s) and not of EWA Publishing and/or the editor(s). EWA Publishing and/or the editor(s) disclaim responsibility for any injury to people or property resulting from any ideas, methods, instructions or products referred to in the content.
About volume
Volume title: Proceedings of CONF-MPCS 2025 Symposium: Mastering Optimization: Strategies for Maximum Efficiency
© 2024 by the author(s). Licensee EWA Publishing, Oxford, UK. This article is an open access article distributed under the terms and
conditions of the Creative Commons Attribution (CC BY) license. Authors who
publish this series agree to the following terms:
1. Authors retain copyright and grant the series right of first publication with the work simultaneously licensed under a Creative Commons
Attribution License that allows others to share the work with an acknowledgment of the work's authorship and initial publication in this
series.
2. Authors are able to enter into separate, additional contractual arrangements for the non-exclusive distribution of the series's published
version of the work (e.g., post it to an institutional repository or publish it in a book), with an acknowledgment of its initial
publication in this series.
3. Authors are permitted and encouraged to post their work online (e.g., in institutional repositories or on their website) prior to and
during the submission process, as it can lead to productive exchanges, as well as earlier and greater citation of published work (See
Open access policy for details).