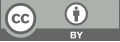
Towards global recognition: Chinese recognition with non-local attention
- 1 Dalian University of Technology
* Author to whom correspondence should be addressed.
Abstract
As a part of image recognition, Chinese character recognition has a great application market in China, such as license plate recognition, logistics information recognition and so on. In recent years, the application of CNN has set off a frenzy of computer vision. Especially in the task of image recognition, CNN is widely used because of its high accuracy and few calculation parameters. However, CNN’s local operation of using repeated filters to process images also has its shortcomings, for example, it can’t pay attention to the relationship between distant pixels in the image. To solve this problem, we tried to add non-local operation to CNN to improve its performance. We chose the classic model of ResNetV2-50 as the foundation, and added non-local blocks to it. We compared the results of the two models and found that the accuracy increased by 4%.
Keywords
convolutional neural network, non-local block, Chinese character recognition
[1]. LeCun, Yann, Yoshua Bengio, and Geoffrey Hinton. (2015) ”Deep learning.” nature 521.7553: 436444.
[2]. Y. Lecun; L. Bottou; Y. Bengio and P. Haffner. (1998) ”Gradient-based learning applied to document recognition”. IEEE
[3]. Krizhevsky, A , Sutskever, I. and Hinton, G.E. (2012) ”ImageNet Classification with Deep Convolutional Neural Networks”.
[4]. Shyang-Lih Chang; Li-Shien Chen; Yun-Chung Chung and Sei-Wan Chen. (2004) ”Automatic license plate recognition”. IEEE
[5]. C.-L. Liu; S. Jaeger and M. Nakagawa. (2004) ”Online recognition of Chinese characters: the state-of-the-art”. IEEE
[6]. Kaiming He, Xiangyu Zhang, Shaoqing Ren, and Jian Sun.(2015) ”Deep residual learning for image recognition”.IEEE.doi:CVPR.2016.90
[7]. Kaiming He, Xiangyu Zhang, Shaoqing Ren, and Jian Sun.(2016)”Identity mappings in deep residual networks”.
[8]. Vaswani, AshishShazeer, NoamParmar, NikiUszkoreit, JakobJones, LlionGomez, Aidan NKaiser, LukaszPolosukhin, and Illia. (2017) ”Attention Is All You Need”. arXiv.1706.03762
[9]. X. Wang, Ross B. Girshick, A. Gupta,and Kaiming He. (2018) “Non-local Neural Networks,”. IEEE. DOI:CVPR.2018.00813.
[10]. B Li,D Lima. (2021) ”Facial expression recognition via ResNet-50”. j.ijcce.2021.02.002.
Cite this article
Guo,Y. (2023). Towards global recognition: Chinese recognition with non-local attention. Theoretical and Natural Science,5,691-697.
Data availability
The datasets used and/or analyzed during the current study will be available from the authors upon reasonable request.
Disclaimer/Publisher's Note
The statements, opinions and data contained in all publications are solely those of the individual author(s) and contributor(s) and not of EWA Publishing and/or the editor(s). EWA Publishing and/or the editor(s) disclaim responsibility for any injury to people or property resulting from any ideas, methods, instructions or products referred to in the content.
About volume
Volume title: Proceedings of the 2nd International Conference on Computing Innovation and Applied Physics (CONF-CIAP 2023)
© 2024 by the author(s). Licensee EWA Publishing, Oxford, UK. This article is an open access article distributed under the terms and
conditions of the Creative Commons Attribution (CC BY) license. Authors who
publish this series agree to the following terms:
1. Authors retain copyright and grant the series right of first publication with the work simultaneously licensed under a Creative Commons
Attribution License that allows others to share the work with an acknowledgment of the work's authorship and initial publication in this
series.
2. Authors are able to enter into separate, additional contractual arrangements for the non-exclusive distribution of the series's published
version of the work (e.g., post it to an institutional repository or publish it in a book), with an acknowledgment of its initial
publication in this series.
3. Authors are permitted and encouraged to post their work online (e.g., in institutional repositories or on their website) prior to and
during the submission process, as it can lead to productive exchanges, as well as earlier and greater citation of published work (See
Open access policy for details).