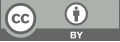
Latent space manipulation of stable diffusion model for image generation
- 1 Georgia Institute of Technology
* Author to whom correspondence should be addressed.
Abstract
The creation of the diffusion model was a piece of exciting news in the deep learning community. Millions of people are excited to try the new DALLE 2 AI artist and the state-of-the-art stable diffusion model. One of the greatest advantages of these AI artists is that they can apply many distinct artistic styles to the same image. Nonetheless, the image created using a specific artistic style sometimes seems too strong or too weak. The ability of adjusting the strength of artistic styles of the generated images enables users of this algorithm greater degrees of freedom in terms of creating images of their wishes. Moreover, the ability of adjusting the strength of artistic styles of the generated images can also serve an educational purpose from which users can understand artistic styles in concreate terms. This paper proposed a novel method of adjusting the level of artistic styles of the generated image which utilized the continuity of the latent space of the Contrastive Language-Image Pretraining (CLIP) encoding and calculate the augmenting latent vector. This novel method provides a greater level of tailored manipulability of the generated image.
Keywords
deep learning, diffusion model, image generation
[1]. Ho, J., Jain, A., & Abbeel, P. (2020). Denoising diffusion probabilistic models. Advances in Neural Information Processing Systems, 33, 6840-6851.
[2]. Menick, J., & Kalchbrenner, N. (2018). Generating high fidelity images with subscale pixel networks and multidimensional upscaling. arXiv preprint arXiv:1812.01608.
[3]. Bond-Taylor, S., Leach, A., Long, Y., & Willcocks, C. G. (2021). Deep generative modelling: A comparative review of VAEs, GANs, normalizing flows, energy-based and autoregressive models. arXiv preprint arXiv:2103.04922.
[4]. Flores Garcia, H., Aguilar, A., Manilow, E., Vedenko, D., & Pardo, B. (2021). Deep Learning Tools for Audacity: Helping Researchers Expand the Artist's Toolkit. In 5th Workshop on Machine Learning for Creativity and Design at NeurIPS 2021.
[5]. Elgammal, A., Liu, B., Elhoseiny, M., & Mazzone, M. (2017). Can: Creative adversarial networks, generating "art" by learning about styles and deviating from style norms. arXiv preprint arXiv:1706.07068.
[6]. Creswell, A., White, T., Dumoulin, V., Arulkumaran, K., Sengupta, B., & Bharath, A. A. (2018). Generative adversarial networks: An overview. IEEE signal processing magazine, 35(1), 53-65.
[7]. Rombach, R., Blattmann, A., Lorenz, D., Esser, P., & Ommer, B. (2022). High-resolution image synthesis with latent diffusion models. In Proceedings of the IEEE/CVF Conference on Computer Vision and Pattern Recognition, 10684-10695.
[8]. Lütkepohl, H. (2013). Vector autoregressive models. In Handbook of Research Methods and Applications in Empirical Macroeconomics, 139-164.
[9]. Goodfellow, I., Pouget-Abadie, J., Mirza, M., Xu, B., et, al. (2020). Generative adversarial networks. Communications of the ACM, 63(11), 139-144.
[10]. Ramesh, A., Pavlov, M., Goh, G., Gray, S., Voss, C., et, al. (2021). Zero-shot text-to-image generation. In International Conference on Machine Learning, 8821-8831.
[11]. Pound, M (2022) Stable Diffusion Version 2.0. URL: https://colab.research.google.com/drive/1roZqqhsdpCXZr8kgV_Bx_ABVBPgea3lX?usp=sharing
Cite this article
Qi,B. (2023). Latent space manipulation of stable diffusion model for image generation. Theoretical and Natural Science,5,763-770.
Data availability
The datasets used and/or analyzed during the current study will be available from the authors upon reasonable request.
Disclaimer/Publisher's Note
The statements, opinions and data contained in all publications are solely those of the individual author(s) and contributor(s) and not of EWA Publishing and/or the editor(s). EWA Publishing and/or the editor(s) disclaim responsibility for any injury to people or property resulting from any ideas, methods, instructions or products referred to in the content.
About volume
Volume title: Proceedings of the 2nd International Conference on Computing Innovation and Applied Physics (CONF-CIAP 2023)
© 2024 by the author(s). Licensee EWA Publishing, Oxford, UK. This article is an open access article distributed under the terms and
conditions of the Creative Commons Attribution (CC BY) license. Authors who
publish this series agree to the following terms:
1. Authors retain copyright and grant the series right of first publication with the work simultaneously licensed under a Creative Commons
Attribution License that allows others to share the work with an acknowledgment of the work's authorship and initial publication in this
series.
2. Authors are able to enter into separate, additional contractual arrangements for the non-exclusive distribution of the series's published
version of the work (e.g., post it to an institutional repository or publish it in a book), with an acknowledgment of its initial
publication in this series.
3. Authors are permitted and encouraged to post their work online (e.g., in institutional repositories or on their website) prior to and
during the submission process, as it can lead to productive exchanges, as well as earlier and greater citation of published work (See
Open access policy for details).