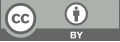
Extensive analysis of rain in australia by exploratory data analysis, feature engineering and modeling
- 1 South China University of Technology
- 2 Shanghai University
* Author to whom correspondence should be addressed.
Abstract
Accurate rainfall forecasts help in planning outdoor activities, agricultural practices, and water resource management, thereby mitigating the impact of extreme weather events. This article provides an in-depth analysis of rainfall in Australia, focusing on predicting whether it will rain tomorrow using logistic regression. The research aims to develop an accurate model to help predict rainfall events for better preparedness and planning. We obtained datasets from a number of Australian weather stations. The dataset contains 142,193 daily weather observations spanning approximately ten years. The recorded information includes various details such as date, location, humidity, wind direction, clouds, temperature, etc. This shows that the model performs well in distinguishing between rainy and non-rainy days with an accuracy of about 0.875. The findings of this study have important implications for various stakeholders including meteorologists, disaster management agencies, and the public.
Keywords
logistic regression, weather forecasting, data analysis, feature engineering, machine learning
[1]. Daniel, L., Sigauke, C., Chibaya, C., Mbuvha, R.: Short-Term Wind Speed Forecasting Using Statistical and Machine Learning Methods. Algorithms 13(6), 132(2020).
[2]. Qi, D., Majda, A. J.: Using machine learning to predict extreme events in complex systems. Proceedings of the National Academy of Sciences 117(1), 52-59 (2019).
[3]. Cifuentes, J., Marulanda, G., Bello, A., Reneses, J.: Air Temperature Forecasting Using Machine Learning Techniques: A Review. Energies13(16), 4215 (2020).
[4]. Joshi, R., Kumar, N., Agarwal, S.: One-Hot Encoding Revisited: High-Cardinality Category Encoding for Machine Learning. Journal of Big Data 8(1), 1-28 (2021).
[5]. Boschetti, A., Massaron, L.: Python Data Science Essentials - Third Edition. Birmingham, UK: Packt Publishing Ltd (2018).
[6]. Zhou, Z.: Machine Learning. Tsinghua University Press (2016).
[7]. Kwak, S. K., Kim, J. H.: Statistical data preparation: management of missing values and outliers. Korean journal of anesthesiology 70(4), 407–411 (2017).
[8]. Ipsen, N. B., Mattei, P. A., Frellsen, J.: How to deal with missing data in supervised deep learning? 10th International Conference on Learning Representations (ICLR 2022), Virtual conference, France (2022).
[9]. Cao, X.H., Stojkovic, I., Obradovic, Z.: A Robust Data Scaling Algorithm to Improve Classification Accuracies in Biomedical Data. BMC Bioinformatics 17(1), 359 (2016).
[10]. Luque, A., Carrasco, A., Martín, A., de las Heras, A.: The impact of class imbalance in classification performance metrics based on the binary confusion matrix. Pattern Recognition 91, 216-231 (2019).
[11]. Lever, J.: Classification evaluation: it is important to understand both what a classification metric expresses and what it hides. Nature Methods 13(8), 603 (2016).
[12]. Narkhede, S.: Understanding auc-roc curve. Towards Data Science 26(1), 220-227 (2018).
Cite this article
Qian,Z.;Sun,K. (2023). Extensive analysis of rain in australia by exploratory data analysis, feature engineering and modeling. Theoretical and Natural Science,7,63-71.
Data availability
The datasets used and/or analyzed during the current study will be available from the authors upon reasonable request.
Disclaimer/Publisher's Note
The statements, opinions and data contained in all publications are solely those of the individual author(s) and contributor(s) and not of EWA Publishing and/or the editor(s). EWA Publishing and/or the editor(s) disclaim responsibility for any injury to people or property resulting from any ideas, methods, instructions or products referred to in the content.
About volume
Volume title: Proceedings of the 2023 International Conference on Environmental Geoscience and Earth Ecology
© 2024 by the author(s). Licensee EWA Publishing, Oxford, UK. This article is an open access article distributed under the terms and
conditions of the Creative Commons Attribution (CC BY) license. Authors who
publish this series agree to the following terms:
1. Authors retain copyright and grant the series right of first publication with the work simultaneously licensed under a Creative Commons
Attribution License that allows others to share the work with an acknowledgment of the work's authorship and initial publication in this
series.
2. Authors are able to enter into separate, additional contractual arrangements for the non-exclusive distribution of the series's published
version of the work (e.g., post it to an institutional repository or publish it in a book), with an acknowledgment of its initial
publication in this series.
3. Authors are permitted and encouraged to post their work online (e.g., in institutional repositories or on their website) prior to and
during the submission process, as it can lead to productive exchanges, as well as earlier and greater citation of published work (See
Open access policy for details).