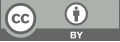
Application of machine learning in wireless communication
- 1 Shenzhen College of International Education
- 2 Nanjing University of Aeronautics and Astronautics
- 3 Beijing University of Posts and Telecommunications
* Author to whom correspondence should be addressed.
Abstract
With the rapid development of wireless communication technology, people have put forward higher requirements for the speed and quality of data transmission in wireless communication while enjoying a convenient life. Wireless communication systems are expected to be combined with artificial intelligence to meet these requirements. Machine learning (ML) can rely on different algorithms to process data without explicit programming. It can also optimize wireless systems by solving complex problems that traditional mathematics cannot solve. This paper briefly introduces wireless communication, machine learning, and the necessity of combining machine learning. The potential and applications of machine learning in various aspects of wireless communication, such as channel estimation, spectrum allocation, adaptive interference suppression, etc., are listed. The paper also introduces the various conveniences that machine learning in wireless communication brings to people in practical applications and the potential hazards that improper applications may bring.
Keywords
Wireless Communication, Machine Learning, Application Situation
[1]. W. Chin, Z. Fan, and R. Haines, 'Emerging technologies and research challenges for 5G wireless networks', IEEE Wireless Commun., vol. 21, no. 2, pp. 106–112, Apr. 2014, doi: 10.1109/MWC.2014.6812298.
[2]. Y. Mao, C. You, J. Zhang, K. Huang, and K. B. Letaief, 'A Survey on Mobile Edge Computing: The Communication Perspective'. arXiv, Jun. 13, 2017. Accessed: Jul. 05, 2023. [Online]. Available: http://arxiv.org/abs/1701.01090
[3]. Y. Sun, M. Peng, Y. Zhou, Y. Huang, and S. Mao, 'Application of Machine Learning in Wireless Networks: Key Techniques and Open Issues'. arXiv, Feb. 28, 2019. Accessed: Jul. 05, 2023. [Online]. Available: http://arxiv.org/abs/1809.08707
[4]. M. A. Alsheikh, S. Lin, D. Niyato, and H.-P. Tan, 'Machine Learning in Wireless Sensor Networks: Algorithms, Strategies, and Applications', IEEE Commun. Surv. Tutorials, vol. 16, no. 4, pp. 1996–2018, 2014, doi: 10.1109/COMST.2014.2320099.
[5]. C. Jiang, H. Zhang, Y. Ren, Z. Han, K.-C. Chen, and L. Hanzo, 'Machine Learning Paradigms for Next-Generation Wireless Networks', IEEE Wireless Commun., vol. 24, no. 2, pp. 98–105, Apr. 2017, doi: 10.1109/MWC.2016.1500356WC.
[6]. M. Bkassiny, Y. Li, and S. K. Jayaweera, 'A Survey on Machine-Learning Techniques in Cognitive Radios', IEEE Commun. Surv. Tutorials, vol. 15, no. 3, pp. 1136–1159, 2013, doi: 10.1109/SURV.2012.100412.00017.
[7]. R. Li, Z. Zhao, X. Chen, J. Palicot, and H. Zhang, 'TACT: A Transfer Actor-Critic Learning Framework for Energy Saving in Cellular Radio Access Networks', IEEE Trans. Wireless Commun., vol. 13, no. 4, pp. 2000–2011, Apr. 2014, doi: 10.1109/TWC.2014.022014.130840.
[8]. T. Park, N. Abuzainab, and W. Saad, 'Learning How to Communicate in the Internet of Things: Finite Resources and Heterogeneity', IEEE Access, vol. 4, pp. 7063–7073, 2016, doi: 10.1109/ACCESS.2016.2615643.
[9]. T. J. O’Shea and J. Hoydis, ‘An Introduction to Deep Learning for the Physical Layer’. arXiv, Jul. 11, 2017. Accessed: Jul. 06, 2023. [Online]. Available: http://arxiv.org/abs/1702.00832
[10]. H. Peng and X. Shen, ‘Deep Reinforcement Learning Based Resource Management for Multi-Access Edge Computing in Vehicular Networks’, IEEE Trans. Netw. Sci. Eng., vol. 7, no. 4, pp. 2416–2428, Oct. 2020, doi: 10.1109/TNSE.2020.2978856.
[11]. X. Shen et al., ‘AI-Assisted Network-Slicing Based Next-Generation Wireless Networks’, IEEE Open J. Veh. Technol., vol. 1, pp. 45–66, 2020, doi: 10.1109/OJVT.2020.2965100.
[12]. M. Chen, U. Challita, W. Saad, C. Yin, and M. Debbah, ‘Artificial Neural Networks-Based Machine Learning for Wireless Networks: A Tutorial’, IEEE Commun. Surv. Tutorials, vol. 21, no. 4, pp. 3039–3071, 2019, doi: 10.1109/COMST.2019.2926625.
Cite this article
Hua,S.;Wang,Q.;Xu,X. (2023). Application of machine learning in wireless communication. Theoretical and Natural Science,12,130-135.
Data availability
The datasets used and/or analyzed during the current study will be available from the authors upon reasonable request.
Disclaimer/Publisher's Note
The statements, opinions and data contained in all publications are solely those of the individual author(s) and contributor(s) and not of EWA Publishing and/or the editor(s). EWA Publishing and/or the editor(s) disclaim responsibility for any injury to people or property resulting from any ideas, methods, instructions or products referred to in the content.
About volume
Volume title: Proceedings of the 2023 International Conference on Mathematical Physics and Computational Simulation
© 2024 by the author(s). Licensee EWA Publishing, Oxford, UK. This article is an open access article distributed under the terms and
conditions of the Creative Commons Attribution (CC BY) license. Authors who
publish this series agree to the following terms:
1. Authors retain copyright and grant the series right of first publication with the work simultaneously licensed under a Creative Commons
Attribution License that allows others to share the work with an acknowledgment of the work's authorship and initial publication in this
series.
2. Authors are able to enter into separate, additional contractual arrangements for the non-exclusive distribution of the series's published
version of the work (e.g., post it to an institutional repository or publish it in a book), with an acknowledgment of its initial
publication in this series.
3. Authors are permitted and encouraged to post their work online (e.g., in institutional repositories or on their website) prior to and
during the submission process, as it can lead to productive exchanges, as well as earlier and greater citation of published work (See
Open access policy for details).