About AEIAdvances in Engineering Innovation (AEI) is a peer-reviewed, fast-indexing open access journal hosted by Tianjin University Research Centre on Data Intelligence and Cloud-Edge-Client Service Engineering and published by EWA Publishing. AEI is published irregularly, and it is a comprehensive journal focusing on multidisciplinary areas of engineering and at the interface of related subjects, including, but not limited to, Artificial Intelligence, Biomedical Engineering, Electrical and Electronic Engineering, Materials Engineering, Traffic and Transportation Engineering, etc.For the details about the AEI scope, please refer to the Aims and Scope page. For more information about the journal, please refer to the FAQ page or contact info@ewapublishing.org. |
Aims & scope of AEI are: · Artificial Intelligence · Computer Sciences · Aerospace Engineering · Architecture & Civil Engineering · Biomedical Engineering · Electrical and Electronic Engineering · Energy and Power Engineering · Materials Engineering · Mechanical Engineering · Traffic and Transportation Engineering |
Article processing charge
A one-time Article Processing Charge (APC) of 450 USD (US Dollars) applies to papers accepted after peer review. excluding taxes.
Open access policy
This is an open access journal which means that all content is freely available without charge to the user or his/her institution. (CC BY 4.0 license).
Your rights
These licenses afford authors copyright while enabling the public to reuse and adapt the content.
Peer-review process
Our blind and multi-reviewer process ensures that all articles are rigorously evaluated based on their intellectual merit and contribution to the field.
Editors View full editorial board
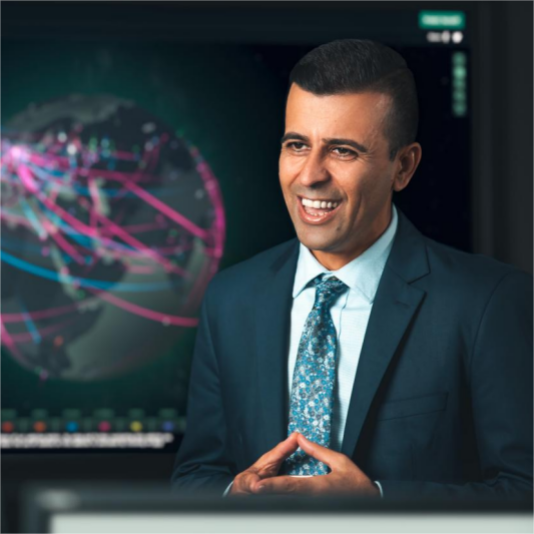
Chicago, US
momar3@iit.edu
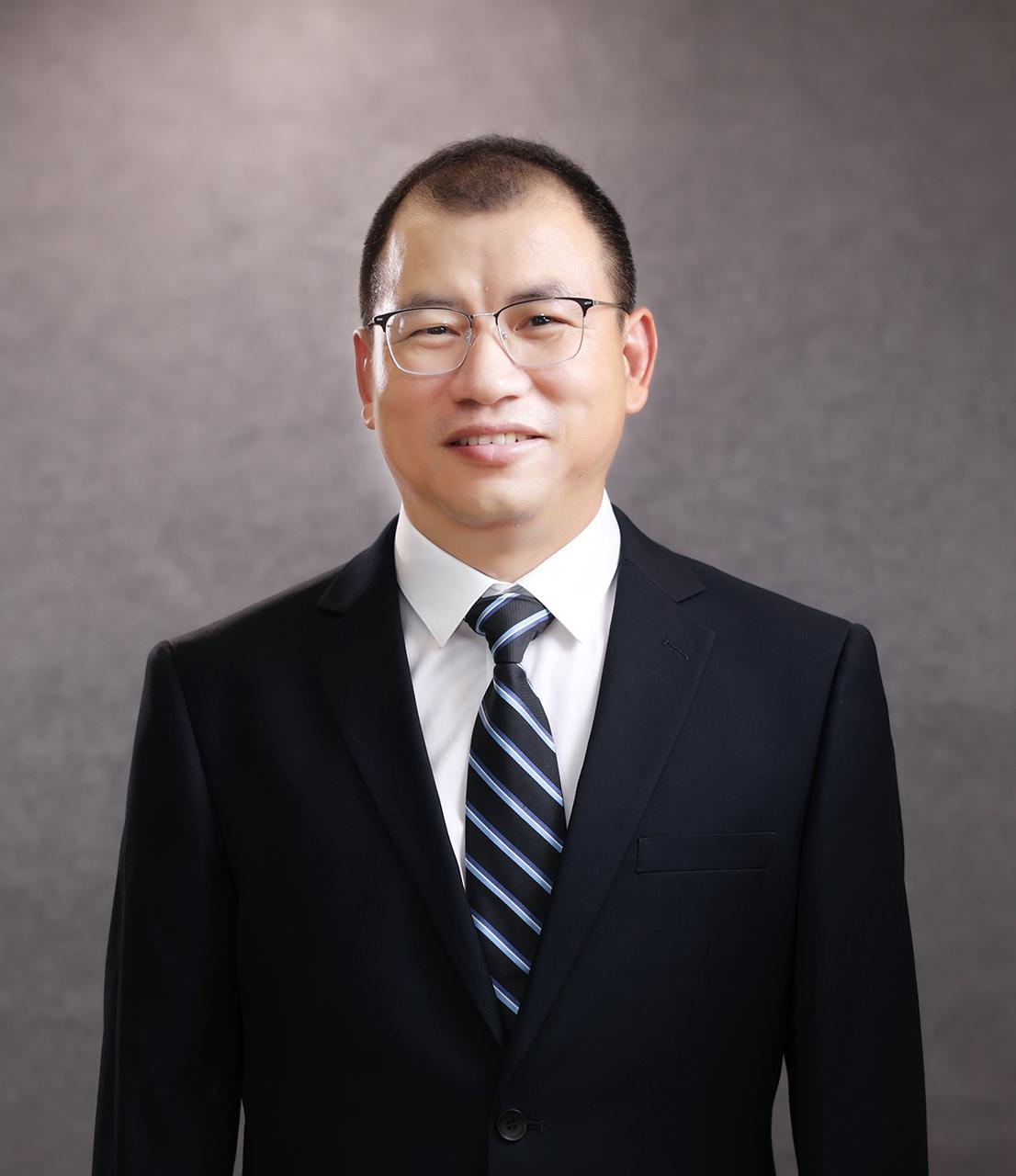
Tianjin, China
rgz@tju.edu.cn
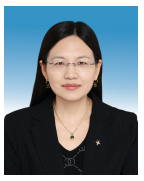
Tianjin, China
zhangli2006@tust.edu.cn
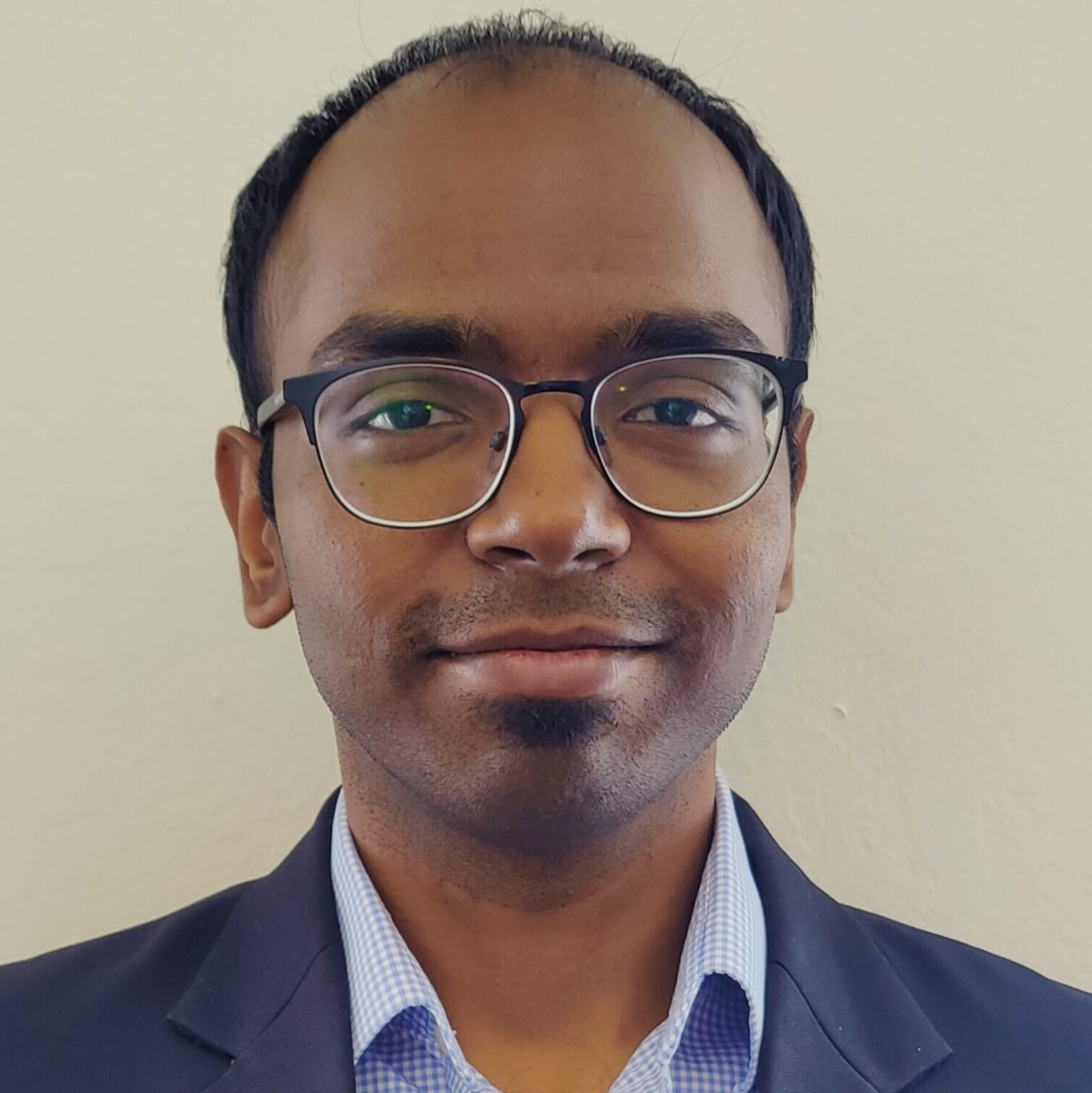
Boston, US
rkpaul@bu.edu
Latest articles View all articles
This study investigates the optimization of ultrasonic-assisted electroless plating to address the issue of high internal porosity in Ni-P Alloy coatings which deposited on X-ray mirror molds. By refining ultrasonic processing parameters, we successfully produced electroless plating layers that meet stringent performance requirements. Experimental results demonstrate that employing an ultrasonic frequency of 40 kHz and an acoustic intensity of 350 W/m² significantly reduces porosity, with internal voids accounting for only 0.03% of the total coating volume—a marked improvement over conventional electroless plating. Additionally, the maximum observed pore radius was limited to 9.8 μm, satisfying the specifications for Ni-P alloy coatings on small-scale X-ray mirror molds.
This paper proposes a CNN-Transformer hybrid model for ink formulation prediction, named CTNet. The model leverages Convolutional Neural Networks (CNN) to extract local features from the spectral reflectance of sample surfaces and incorporates the self-attention mechanism of the Transformer to achieve efficient mapping between color and formulation. In addition, Bayesian optimization is introduced for hyperparameter tuning, further enhancing model performance. Experimental results demonstrate that CTNet outperforms CNN, RNN, LSTM, and the standard Transformer model in terms of Mean Absolute Error (MAE), achieving higher prediction accuracy. This provides an effective solution for high-precision and automated ink color matching, showing promising potential for industrial applications.
This study thoroughly investigates how civil engineering management can effectively control project costs. Through analyzing key cost control strategies within civil engineering management, the research reveals the significance of economic principles and rational resource utilization. Using a case study approach, diagnostic analysis of actual engineering projects was conducted, validating the effectiveness of measures such as detailed budgeting, strengthened cost accounting, and enhanced cost management. The findings suggest that implementing scientifically sound civil engineering management can significantly reduce project costs and enhance project efficiency. Additionally, the study recommends reforming traditional management approaches and integrating modern management concepts and methodologies, further optimizing cost control in civil engineering projects. The results offer essential reference value for improving cost management, increasing project benefits, and promoting long-term civil engineering development.
To address the challenges in detecting abrasion-resistant color fastness samples – including limited sample instances, non-uniform shapes, and insufficiently distinct texture variations that compromise localization accuracy – this paper optimizes the detection framework through the integration of three key strategies: Global Attention Mechanism (GAM), Dynamic Sampling (DySample), and Adaptively Spatial Feature Fusion (ASFF), thereby enhancing detection accuracy and efficiency. Initially, Mosaic data augmentation is implemented to enrich dataset diversity and improve model robustness. Subsequently, the GAM attention mechanism is embedded into the backbone network to enhance target feature extraction capabilities. DySample replaces conventional upsampling methods in the neck network to achieve more effective feature reconstruction. Finally, the ASFF module is integrated into the Detect module within the head network to enable adaptively spatial weight learning for multi-scale feature map fusion. Compared with baseline algorithms, the improved framework demonstrates performance gains of 1.2% in Precision, 3.0% in Recall, 1.2% in mAP@0.5, and 13.5% in mAP@0.5:0.95. Experimental results validate the effectiveness of the proposed method, which maintains satisfactory performance across additional datasets, demonstrating strong robustness and superior generalization capability.
Volumes View all volumes
2025
Volume 16April 2025
Find articlesVolume 16March 2025
Find articlesVolume 16March 2025
Find articles2024
Volume 14December 2024
Find articlesVolume 13November 2024
Find articlesVolume 12October 2024
Find articlesAnnouncements View all announcements
Advances in Engineering Innovation
We pledge to our journal community:
We're committed: we put diversity and inclusion at the heart of our activities...
Advances in Engineering Innovation
The statements, opinions and data contained in the journal Advances in Engineering Innovation (AEI) are solely those of the individual authors and contributors...
Indexing
The published articles will be submitted to following databases below:
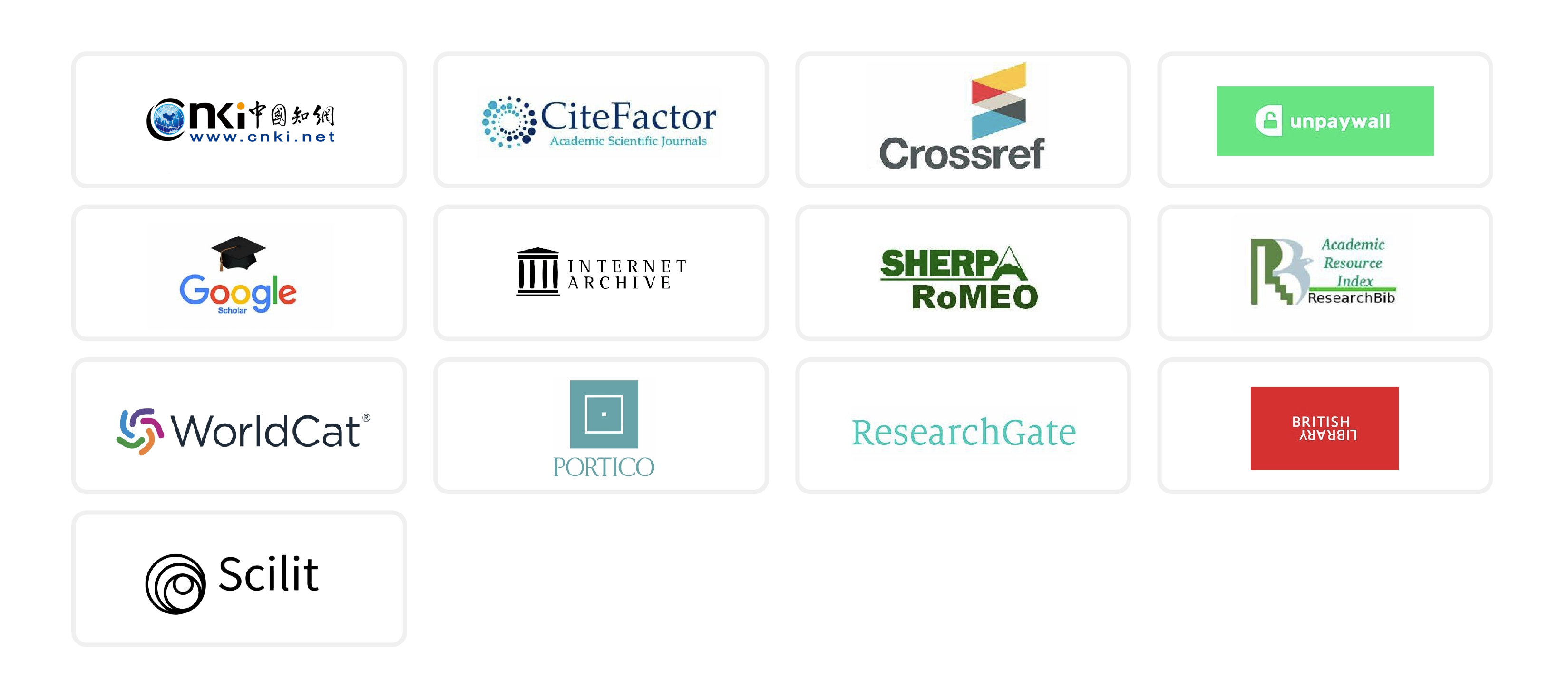