Volume 92
Published on October 2024Volume title: Proceedings of the 6th International Conference on Computing and Data Science
As computing and communication technologies continue to evolve, leading technology companies are investing significant resources in self-driving car techniques. With the use of deep learning in driverless driving, driverless cars are enabling them to sense, make decisions and control in complex environments, leading to truly autonomous driving. Deep learning is used for environment perception, which enables driverless cars to understand their surroundings by recognizing information such as road surfaces, pedestrians and vehicles, and also used for decision formulation, by predicting the behavior of other vehicles, pedestrians and identifying the environment around the road conditions to control the driverless car to make the optimal driving decision. But in order to achieve fully autonomous driving, there are still some technical aspects. Therefore, we deep learning in the existing driverless technology is reviewed, mainly discussing the application in the direction of driverless implementation and safety inspection. Through reading a large amount of literature, we discuss the problems that hinder the development of the driverless field, and at the same time, how to solve the problems in the future to promote the breakthrough of the driverless field. The application of deep learning in driverless not only enhances the intelligence level of driverless cars, but also prepares for the commercial application of driverless cars in the future. It enables driverless cars to better identify and adapt to complex traffic environments. Finally, realizing truly autonomous driving.
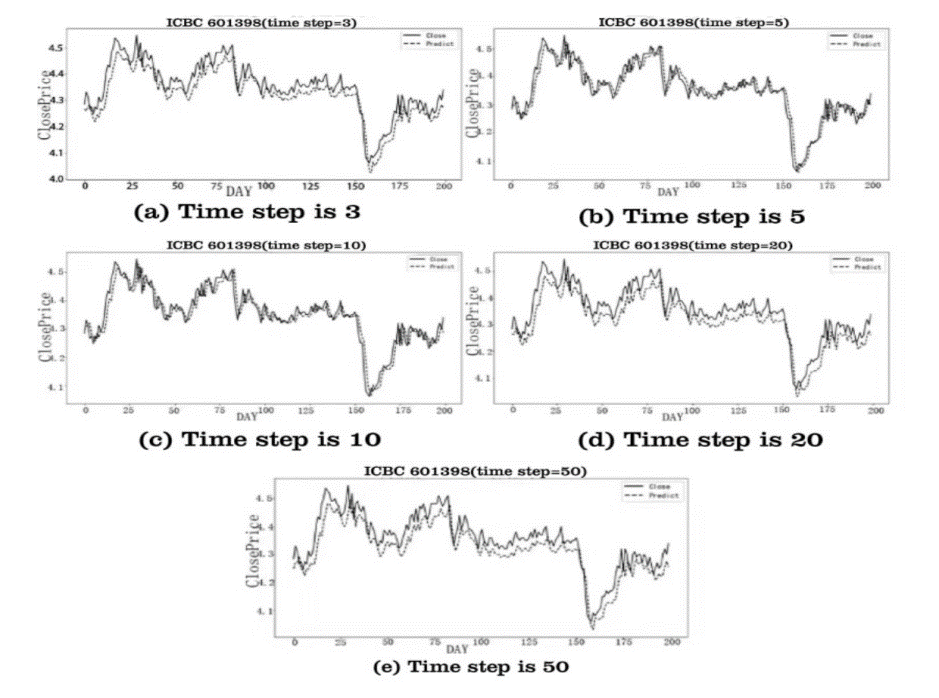
With the rapid development of information technology, Big Data has emerged as one of the important characteristics of the contemporary era. In the secondary market, the application of Big Data is increasingly widespread, which has a profound impact on market structure, trading patterns, and investment decisions. This paper aims to explore the utilization of Big Data in the secondary market, including data analysis techniques, enhancement of decision efficiency, augmentation of market transparency, and innovation in risk management practices while discussing the drawbacks of using Big Data. Then, the study focuses on how to improve and enhance the application of big data in the secondary market in the future. The findings underscore that Big Data plays an instrumental role in shaping the dynamics of the secondary market, this is predominantly manifested in the prediction of stock market trends, the assessment of investment risks, and so on. However, certain challenges persist. Continual development and improvement of Big Data can facilitate more informed investment decision-making.
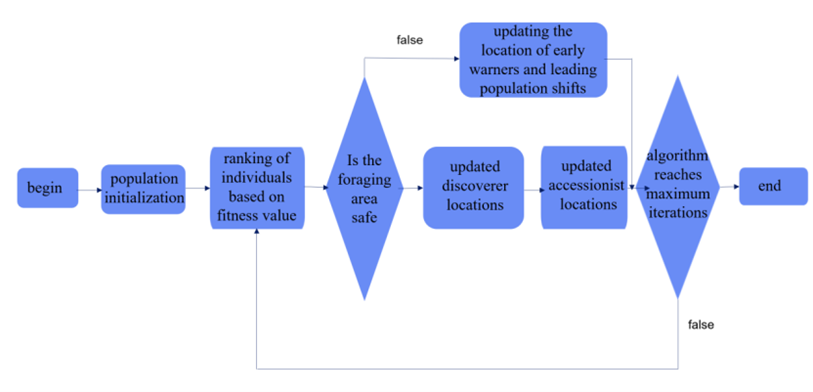
Unmanned aerial vehicle, generally referred to as UAV, due to its high-performance and low-cost characteristics, it is particularly widely used in both military and civil applications, and is often used to perform a variety of complex missions. Trajectory planning is the basis for UAVs to realize autonomous flight, which ensures that UAVs avoid obstacles and threatening areas when performing tasks, and guarantees the safe execution of tasks. The operation of a UAV requires finding the safest and most efficient trajectory from a starting point to a specified end point based on several specific constraints. Current UAV trajectory planning algorithms, such as swarm algorithm, particle swarm algorithm, and ant colony algorithm, have been widely recognized, but still have many limitations when they are applied to complex and variable terrain. Therefore, this paper proposes an improved UAV trajectory planning algorithm based on simulated bird migration, and uses matlab for simulation and algorithm evaluation. The simulation results indicate that the improved search strategy is effective, the algorithm can find a better solution in each iteration, and the search strategy of the algorithm can effectively guide the algorithm towards a more optimized region. Overall, this algorithm has high research value in both military and civilian fields.
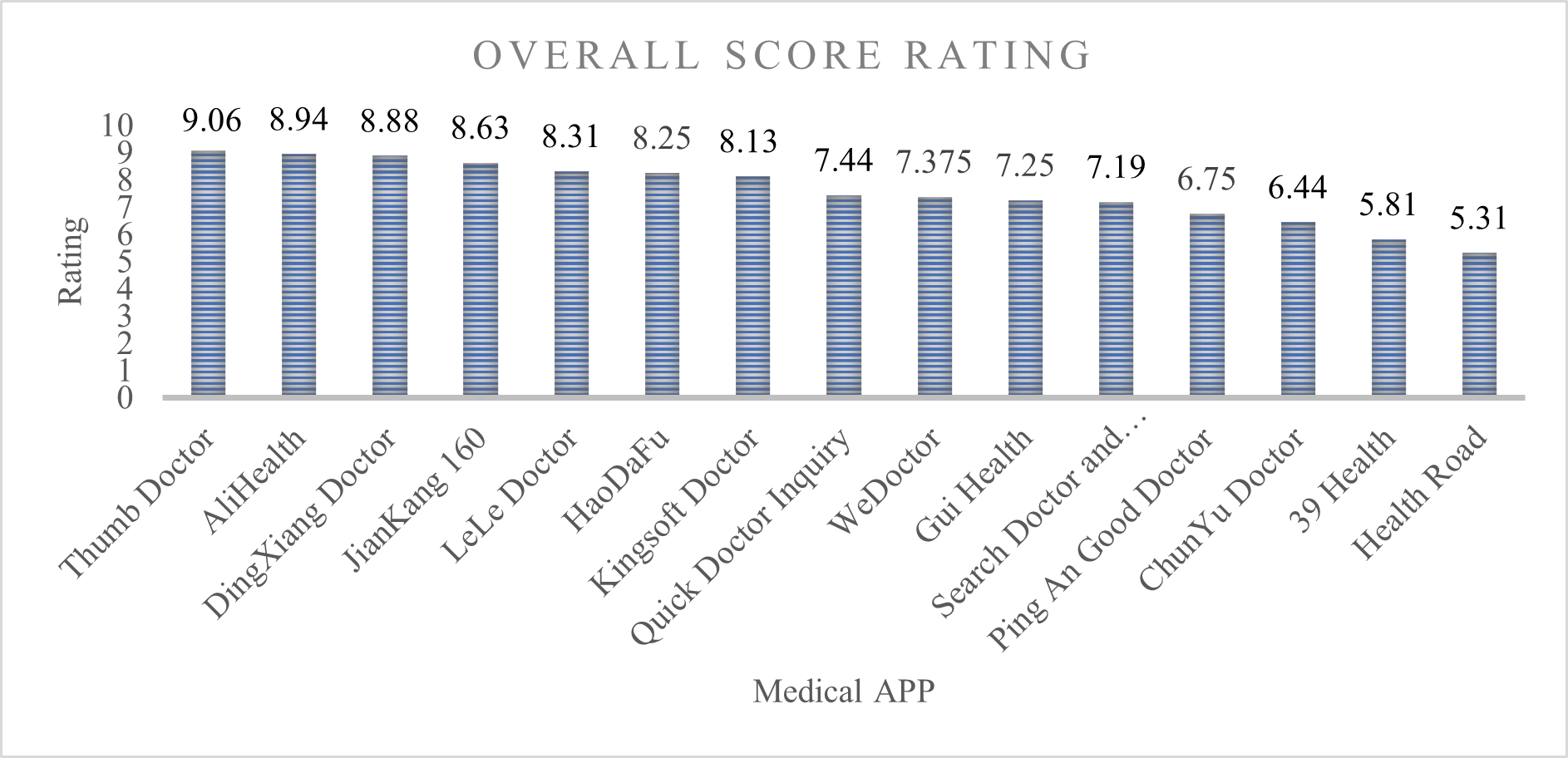
In light of the rapid advancement of internet-based healthcare and the consequent transformation in medical consultation modalities, an increasing number of individuals, particularly the younger demographic, are opting to seek medical advice or assistance via mobile phones through various online medical platforms or applications. However, the wide range of medical apps with different operational models, varying levels of expertise among resident specialists, and inconsistent quality of medical guidance presents a significant challenge for users in discerning which apps provide accurate and reliable guidance. Consequently, this study aims to comprehensively evaluate the service quality of domestic medical apps from multiple channels and perspectives by utilizing case studies. Rooted in educational materials and diagnostic guidelines for relative diseases, evaluation criteria and a quantitative scoring system are established in this study. The findings are indicated in terms of the accuracy and professionalism of medical consultation services and user experience. This study also proposes that domestic mobile medical apps should enhance their diagnostic quality, expand physician resources, standardize management practices, and strengthen platform infrastructure while developing new functionalities to benefit patients.
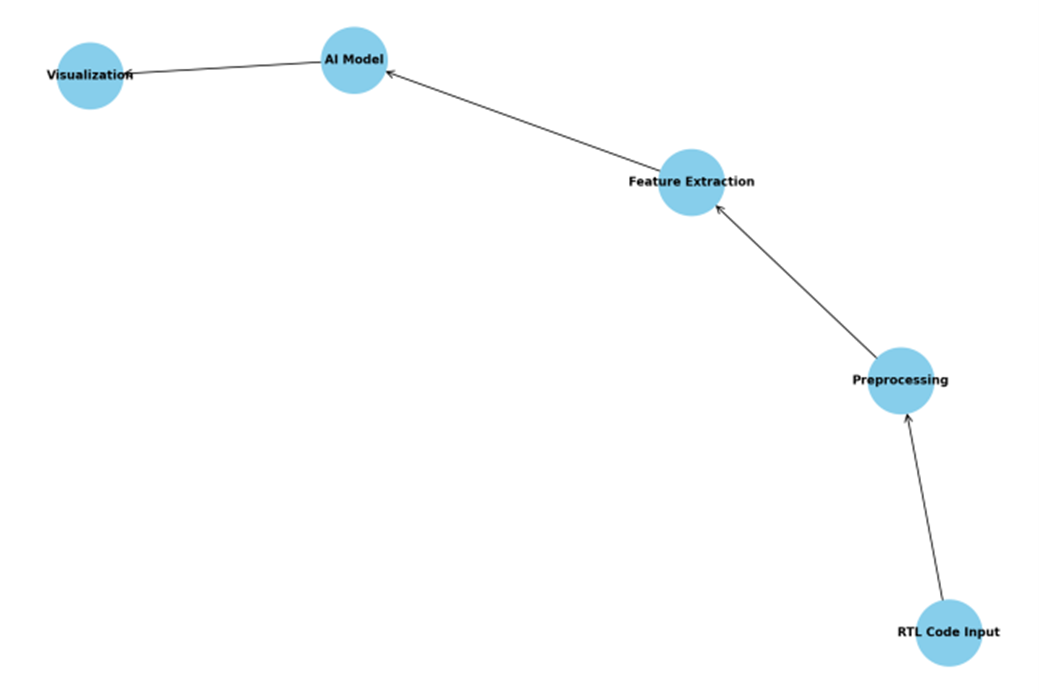
This paper presents a novel AI-driven approach for enhancing chip design verification through automated bug detection in Register Transfer Level (RTL) code. The proposed method integrates advanced machine learning techniques with domain-specific knowledge of chip design to address the challenges of increasing complexity and time-to-market pressures in modern integrated circuit development. Our system employs a comprehensive data preprocessing pipeline that effectively captures syntactic and semantic features of RTL code, feeding into an innovative attention-based neural network model. The model demonstrates superior bug detection accuracy across diverse design categories and bug types compared to traditional methods and existing AI-assisted approaches. Extensive experimental evaluation on a large-scale dataset of RTL designs, including both open-source projects and industry collaborations, validates the effectiveness of our method. The proposed approach achieves a 95% accuracy in bug detection, with a 28-35% reduction in verification time when applied to real-world chip design projects. The paper addresses the interpretability of AI decisions in the context of chip design, presenting novel visualization techniques that enhance trust and facilitate adoption among RTL designers. While acknowledging current limitations, we discuss future research directions, including integration with formal verification methods and extension to system-level verification scenarios. This work contributes significantly to AI-assisted chip design, paving the way for more efficient and reliable development of complex integrated circuits.
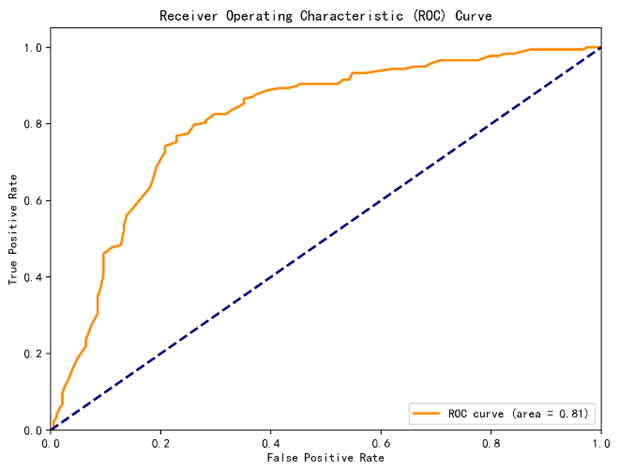
The disparity in the development and application of educational resources between urban and rural areas is pronounced, with rural students often encountering significant challenges in acquiring geographic knowledge and developing spatial cognitive skills due to their geographical isolation and limited access to educational resources. This study aims to investigate the effectiveness of geography education resource development in rural areas by examining the influence of various educational resource characteristics on students' scores in geography education. Through the application of data analysis and machine learning predictive methods, this research explores the impact of 13 variables, including gender, age, parental education level, family background, internet accessibility, outdoor practical activities, and family outdoor travel frequency. These factors were modeled and assessed using a range of machine learning algorithms. The findings reveal differential impacts of these characteristics on students' scores in geography education, with models such as Random Forest and XGBoost demonstrating superior performance in predicting scores in geography courses. This research provides empirical data and a scientific framework to support the optimization of geography education resources in rural areas, offering theoretical and practical insights for advancing educational equity and fostering local socioeconomic development.
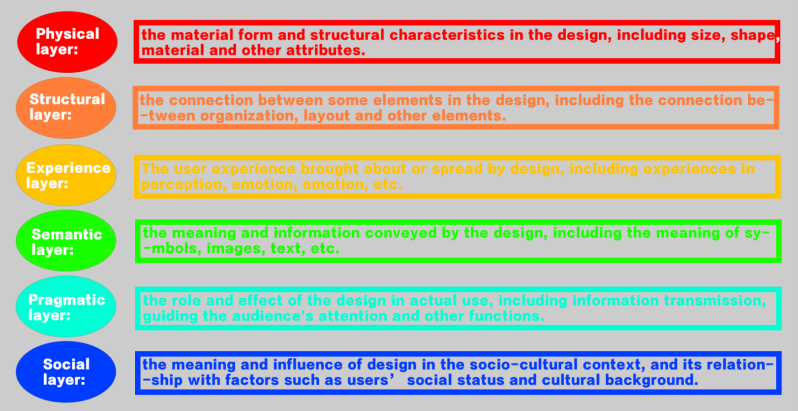
In today's information age, the diversification of interaction methods has led to a focus on building interactive cognitive capabilities within the design academic community. While these methods promote social multi-modal integration, they also introduce challenges such as increased cognitive learning costs, complexity, and unclear feedback. This article explores a new paradigm for visual function compensation in interactive cognitive poster design, utilizing the "behavior-meaning-object" effectiveness from the SAPAD theoretical framework and integrating the TOPSIS method for evaluation. The goal is to objectively assess design solutions and guide designers in creating more effective strategies. Additionally, the research addresses systematic approaches for planning functional compensation and interaction scales in visual communication, offering insights to enhance the current direction of interaction design in China. Developing China's interactive cognitive framework is a long-term process that involves resolving differences through sharing, communication, and mutual promotion. Designers should focus on the connections between system elements and prioritize audience needs, aiming to create design solutions that bridge gaps in interaction models.
The rapid proliferation of big data across various industries, such as healthcare, finance, and social media, has created an urgent need for robust and efficient distributed neural network training frameworks. These frameworks must handle vast volumes of data while ensuring high performance and accuracy in machine learning tasks. As organizations increasingly rely on machine learning models to extract actionable insights from big data, selecting the right framework becomes critical. This paper provides a comprehensive comparison of two leading distributed neural network training frameworks: Apache Spark and TensorFlow. These frameworks are widely adopted due to their scalability and flexibility in handling complex data-driven tasks. The study evaluates their performance and accuracy in big data modeling and prediction, focusing on key metrics such as training speed, model accuracy, resource utilization, and scalability. Utilizing datasets that mirror real-world applications, the study includes thorough data preprocessing, model construction, and distributed training experiments across both frameworks. The findings reveal that while TensorFlow achieves superior model accuracy, Apache Spark demonstrates better scalability and resource efficiency, particularly in large-scale data environments. These insights offer valuable guidance for researchers and industry practitioners in selecting the most appropriate framework for their specific big data applications, ensuring optimal performance and resource management.
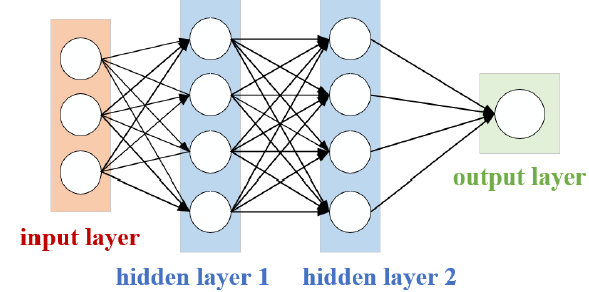
In recent years, deep learning technology has made remarkable achievements in the field of computer vision, promoting the rapid development of image recognition, image generation, image understanding and other tasks. The combination of deep learning and computer vision has brought revolutionary changes to tasks such as image recognition, image generation and image understanding. This paper systematically introduces the development of deep learning and computer and the evolution of key technologies. Further, in order to give readers a deeper understanding of the field of computer vision, this article details the latest research results of some well-known scholars in computer vision, which are published in flagship conferences. The main contribution of this paper is to review the latest research results of computer vision in detail, and look forward to the future research direction and space in this field.
Eating disorders (ED) have the highest case mortality rate among all mental health issues, and their prevalence has increased significantly in recent years. However, fewer than 20% of sufferers receive treatment. Chatbots, or conversational agents, that target EDs are a promising way to bridge this gap and supplement existing support by being cost-effective, accessible, and non-human. The technical compositions behind these chatbots vary, each with their own strengths and weaknesses. Generally, the field of ED chatbots is emerging and only a handful of platforms have come out, including one designed to replace ED helpline support and one to support youth body image. While many ethical and practical challenges affect ED chatbots, there are ways to make progress, and the collaboration between developers, clinicians, ethicists, and the public will be vital in this pursuit. Integrating ED chatbots into healthcare and social media platforms are future directions that could significantly advance ED healing.