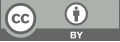
Correlation between fishing yield and microplastic levels across nations
- 1 Wuhan Britain-China School, Wuhan, China
* Author to whom correspondence should be addressed.
Abstract
This research looks into the association between fishing and microplastic contamination across countries, with a particular focus on how the growth of microplastic levels can impact fishery production. Through the use of a mixed-effect model we were able to look through data from 100 nations during the period 1990-2021, where fishery yield data was extracted from The World Bank and microplastic concentration data was obtained from the National Centers for Environmental Information; population data served as a control variable in our analysis. We found that there is indeed a significant negative correlation between levels of microplastics and fishing yield: an increase by 1 piece of microplastic per cubic meter leads to decrease in fishing yield by anything between 65 and 100 metric tonnes (95% confidence interval). This relationship held true for about 93% of all coastal countries studied. To accommodate for differences amongst nations, we introduced random intercepts and slopes in our mixed-effect model which helped capture variations specific to each country while still identifying an overarching pattern. The research we are doing is on the connection between fish catches and microplastic pollution which takes place in the different countries of the world, where we focus more on how high microplastic levels influence fishery production. Having made use of a mixed-effect model, we have been able to look at data that represents 100 nations within the period of time between 1990 and 2021; The World Bank provided us with fishery yield data while microplastic concentration data came from the National Centers for Environmental Information. In addition to these variables, population data was used as a control variable. The summary of our analysis points towards a significant inverse relationship noted between microplastic levels and fishing yield: an increase by one piece of plastic results in a decline by somewhere between 65 to 100 metric tonnes (95% confidence interval). This generalization held true for about 93% coastal countries considered under this study. To capture specific variations among nations but also identify an overall trend line while dealing with inter-country variability, random intercepts and slope components were included as part of our mixed-effect model methodology.
Keywords
Microplastic levels, Fishing yield, Mixed Effect Model, Correlation
[1]. Akanyange, S. N., Zhang, Y., Zhao, X., Adom-Asamoah, G., Ature, A. R. A., Anning, C., ... & Crittenden, J. C. (2022). A holistic assessment of microplastic ubiquitousness: Pathway for source identification in the environment. Sustainable Production and Consumption, 33, 113-145.
[2]. Zeng, E. Y. (Ed.). (2023). Microplastic contamination in aquatic environments: an emerging matter of environmental urgency. Elsevier.
[3]. Cole, M., Lindeque, P., Halsband, C., & Galloway, T. S. (2011). Microplastics as contaminants in the marine environment: a review. Marine pollution bulletin, 62(12), 2588-2597.
[4]. Rochman, C. M., Hoh, E., Kurobe, T., & Teh, S. J. (2013). Ingested plastic transfers hazardous chemicals to fish and induces hepatic stress. Scientific reports, 3(1), 1-7.
[5]. Pauly, D., & Zeller, D. (2016). Catch reconstructions reveal that global marine fisheries catches are higher than reported and declining. Nature communications, 7(1), 10244.
[6]. Perissi, I., Bardi, U., El Asmar, T., & Lavacchi, A. (2017). Dynamic patterns of overexploitation in fisheries. Ecological modelling, 359, 285-292.
[7]. Costello, C., Ovando, D., Clavelle, T., Strauss, C. K., Hilborn, R., Melnychuk, M. C., ... & Leland, A. (2016). Global fishery prospects under contrasting management regimes. Proceedings of the national academy of sciences, 113(18), 5125-5129.
[8]. Chaturvedi, S., Yadav, B. P., Siddiqui, N. A., & Chaturvedi, S. K. (2020). Mathematical modelling and analysis of plastic waste pollution and its impact on the ocean surface. Journal of Ocean Engineering and Science, 5(2), 136-163.
[9]. Besseling, E., Quik, J. T., Sun, M., & Koelmans, A. A. (2017). Fate of nano-and microplastic in freshwater systems: A modeling study. Environmental pollution, 220, 540-548.
[10]. Henderson, L., & Green, C. (2020). Making sense of microplastics? Public understandings of plastic pollution. Marine pollution bulletin, 152, 110908.
[11]. Anderson, A. G., Grose, J., Pahl, S., Thompson, R. C., & Wyles, K. J. (2016). Microplastics in personal care products: Exploring perceptions of environmentalists, beauticians and students. Marine pollution bulletin, 113(1-2), 454-460.
[12]. Bolker, B. M., Brooks, M. E., Clark, C. J., Geange, S. W., Poulsen, J. R., Stevens, M. H. H., & White, J. S. S. (2009). Generalized linear mixed models: a practical guide for ecology and evolution. Trends in ecology & evolution, 24(3), 127-135.
[13]. Zuur, A. F., Ieno, E. N., Walker, N. J., Saveliev, A. A., & Smith, G. M. (2009). Mixed effects models and extensions in ecology with R (Vol. 574, p. 574). New York: springer.
[14]. Wood, S. N. (2011). Fast stable restricted maximum likelihood and marginal likelihood estimation of semiparametric generalized linear models. Journal of the Royal Statistical Society Series B: Statistical Methodology, 73(1), 3-36.
[15]. Henson, R., & Friston, K. (2007). Convolution models for fMRI. Statistical parametric mapping: The analysis of functional brain images, 178-192.
[16]. Patterson, H. D., & Thompson, R. (1971). Recovery of inter-block information when block sizes are unequal. Biometrika, 58(3), 545-554.
[17]. https://experience.arcgis.com/experience/b296879cc1984fda833a8acc93e31476/page/Page/?views=Data-Table,Data-Information#data_s=id%3AdataSource_1-18cf9a85fdd-layer-4%3A20760
[18]. https://experience.arcgis.com/experience/b296879cc1984fda833a8acc93e31476/page/Page/?views=Data-Table,Data-Information#data_s=id%3AdataSource_1-18cf9a85fdd-layer-4%3A20760
[19]. https://data.worldbank.org/indicator/SP.POP.TOTL?end=
[20]. Eriksen, M., Cowger, W., Erdle, L. M., Coffin, S., Villarrubia-Gómez, P., Moore, C. J., ... & Wilcox, C. (2023). A growing plastic smog, now estimated to be over 170 trillion plastic particles afloat in the world’s oceans—Urgent solutions required. Plos one, 18(3), e0281596.
Cite this article
Wang,Z. (2024). Correlation between fishing yield and microplastic levels across nations. Theoretical and Natural Science,42,192-202.
Data availability
The datasets used and/or analyzed during the current study will be available from the authors upon reasonable request.
Disclaimer/Publisher's Note
The statements, opinions and data contained in all publications are solely those of the individual author(s) and contributor(s) and not of EWA Publishing and/or the editor(s). EWA Publishing and/or the editor(s) disclaim responsibility for any injury to people or property resulting from any ideas, methods, instructions or products referred to in the content.
About volume
Volume title: Proceedings of the 2nd International Conference on Mathematical Physics and Computational Simulation
© 2024 by the author(s). Licensee EWA Publishing, Oxford, UK. This article is an open access article distributed under the terms and
conditions of the Creative Commons Attribution (CC BY) license. Authors who
publish this series agree to the following terms:
1. Authors retain copyright and grant the series right of first publication with the work simultaneously licensed under a Creative Commons
Attribution License that allows others to share the work with an acknowledgment of the work's authorship and initial publication in this
series.
2. Authors are able to enter into separate, additional contractual arrangements for the non-exclusive distribution of the series's published
version of the work (e.g., post it to an institutional repository or publish it in a book), with an acknowledgment of its initial
publication in this series.
3. Authors are permitted and encouraged to post their work online (e.g., in institutional repositories or on their website) prior to and
during the submission process, as it can lead to productive exchanges, as well as earlier and greater citation of published work (See
Open access policy for details).